Fairness and Bias Mitigation for Digital Marketing Strategies & Best Practices
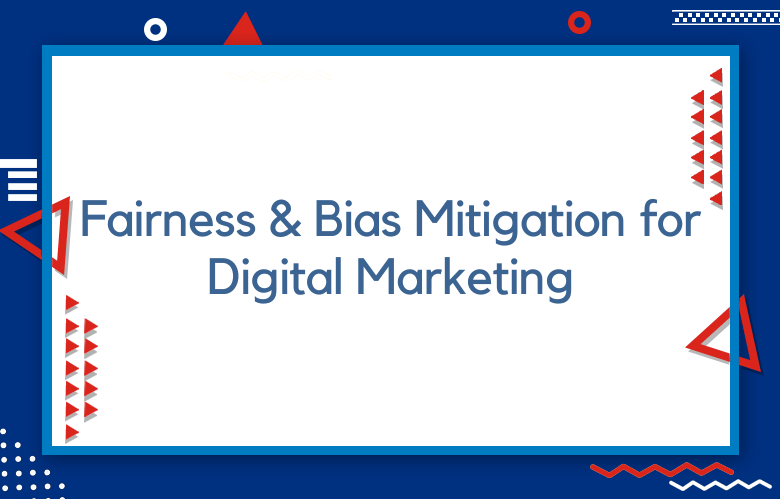
In digital marketing, fairness and bias mitigation are crucial considerations, as algorithms and data-driven strategies significantly shape customer experiences and outcomes. Unaddressed biases in data or algorithms can lead to unfair treatment of specific customer groups, reinforce stereotypes, and result in skewed marketing insights, potentially harming the brand reputation and customer trust.
Fairness and Bias Mitigation for Digital Marketing: Strategies & Best Practices
Fairness in digital marketing ensures that marketing strategies, algorithms, and data practices do not inadvertently favor or discriminate against specific audience segments. This includes being mindful of how data is collected, analyzed, and used to make marketing decisions, from ad targeting and content personalization to customer segmentation and predictive analytics.
Bias mitigation strategies involve a proactive approach to identifying, assessing, and addressing potential biases at every stage of the marketing process. This includes diversifying data sources to prevent narrow or skewed perspectives, regularly auditing algorithms for biased outcomes, and involving diverse teams in developing and implementing marketing strategies to bring a wide range of perspectives and reduce unconscious biases.
Best practices for ensuring fairness and mitigating bias in digital marketing also include transparency about data use, providing customers with control over their data, and adhering to ethical guidelines and regulatory requirements related to data privacy and consumer rights.
By prioritizing fairness and bias mitigation, digital marketers can create more inclusive, effective, and ethical marketing strategies that resonate with diverse audiences, build stronger customer relationships, and drive sustainable business growth.
- Key Takeaways
- Understanding Digital Marketing Bias
- Identifying Marketing Data Biases
- Impact of AI Bias
- Preventing Bias Strategies
- Detecting Algorithmic Bias
- Reducing Bias Techniques
- Ethical Considerations in AI
- Fairness vs. Accuracy Trade-offs
- Regulatory Measures for Bias
- Summary
- Frequently Asked Questions
- What is digital marketing bias?
- How can bias be identified in marketing data?
- Why is detecting algorithmic bias significant in digital marketing?
- What are some techniques for reducing bias in digital marketing?
- Why are fairness vs. accuracy trade-offs significant in AI applications for digital marketing?
In digital marketing, ensuring fairness and diversity and mitigating bias in algorithmic decision-making are paramount. By understanding these concepts, businesses can reach a broader audience while fostering trust and credibility.
Fairness in digital marketing involves providing equal opportunities for all consumers, regardless of background, demographics, race, or big data. On the other hand, bias mitigation aims to eliminate discriminatory practices that may hinder the effectiveness of marketing strategies by promoting nondiscrimination and diversity.
Striking a balance between fairness, nondiscrimination, and bias mitigation is crucial for creating inclusive and impactful campaigns that resonate with diverse audiences. Join us as we delve into these topics in digital marketing, utilizing technology and big data.
Key Takeaways
Understanding Digital Marketing Bias
Recognize the presence of bias and discrimination in digital marketing strategies to address it effectively and make decisions.
Identifying Marketing Data Biases
Scrutinize data sources for potential biases that can skew marketing decisions and outcomes.
Impact of AI Bias
Understand how training data and decision-making with technology can perpetuate discrimination and influence digital marketing campaigns negatively.
Preventing Bias Strategies
Implement proactive measures like diverse dataset collection to mitigate biases in digital marketing initiatives and decision-making.
Detecting Algorithmic Bias
Utilize tools and methods to identify and rectify algorithmic biases and discrimination in digital marketing algorithms.
Reducing Bias Techniques
Employ algorithm transparency and fairness constraints to reduce biases in digital marketing practices, decision-making, and discrimination.
Understanding Digital Marketing Bias
Define Bias
Bias in digital marketing refers to systemic errors or deviations from the actual values in data collection, analysis, interpretation, and decision-making processes. It can significantly impact research outcomes, leading to flawed insights and ineffective strategies, affecting decision-making, and risking people.
Types of Biases
Selection Bias
This occurs when segments of the audience, which put people overrepresented or underrepresented in the data, skew results.
Confirmation Bias
It involves favoring information that confirms preconceived beliefs while ignoring contradictory evidence.
Measurement Bias
Arises from inaccuracies in data collection methods, leading to unreliable insights.
Algorithmic Bias
Refers to biases embedded in algorithms that target specific demographics or exclude others unfairly.
Importance of Addressing Biases
Enhanced Decision-Making
Mitigating biases ensures that decisions are based on accurate and unbiased information, leading to more effective campaigns.
Improved Audience Targeting
By reducing biases, marketers can reach a broader and more diverse audience, enhancing engagement and conversion rates.
Ethical Considerations
Addressing biases is crucial for upholding ethical standards in digital marketing practices and promoting fairness among all audience segments.
Identifying Marketing Data Biases
Selection Bias
Marketers must recognize potential biases in their data collection processes, including AI. One common type is selection bias, where certain groups are overrepresented, skewing results. This can occur when marketing campaigns target specific demographics more than others.
Biases like selection bias can distort the accuracy of insights drawn from marketing data. For instance, if a company predominantly collects feedback from younger customers, it may overlook the preferences of older demographic groups. As a result, marketing strategies may not effectively resonate with all customer segments.
Response Bias
When analyzing marketing data, it’s crucial to address human biases that can influence responses. Response bias occurs when participants provide inaccurate information due to social desirability or other factors. This can lead to misleading conclusions about customer behaviors and preferences.
In digital marketing, response bias can manifest in various ways. For example, customers might be inclined to give positive feedback on surveys to please the brand, even if their experiences differ. This skewed data can misguide marketers in understanding true customer sentiments and needs.
Impact of AI Bias
Detecting Bias
AI systems can perpetuate discrimination in digital marketing by amplifying existing biases in the data on which they are trained. These biases can lead to unfair targeting and exclusion of specific demographics.
Challenges arise in detecting bias within AI algorithms used in marketing. The complexity of these systems makes it difficult to pinpoint where biases may be present, leading to potential harms such as reinforcing stereotypes or limiting opportunities for specific groups.
Mitigating Unintended Consequences
Mitigating AI bias in marketing algorithms is crucial to ensure fair and ethical practices. Strategies such as diversifying training data and implementing bias detection tools can help reduce the impact of biases on targeted advertising campaigns.
Pros
- Enhances inclusivity
- Improves brand reputation
Cons
- Requires additional resources
- Challenges in identifying all sources of bias
Ethical Implications
Using inherent biases in marketing strategies raises significant ethical concerns. Marketers must consider the consequences of using biased algorithms, which can result in discriminatory practices that alienate potential customers.
Addressing these ethical implications involves a proactive approach toward bias mitigation, transparency in algorithmic decision-making, and continuous monitoring for any signs of discriminatory outcomes.
Preventing Bias Strategies
Research Process
Implement strategies for a robust research process to prevent bias in digital marketing. Before data collection, begin by defining clear objectives and hypotheses. Utilize diverse datasets to ensure nondiscrimination and representation of various groups.
Employ rigorous statistical analyses to identify and address any biases during the research phase. Regularly review and validate the data sources to maintain the integrity of the findings. By adopting these methods, organizations can mitigate potential biases effectively.
Data Collection Methods
Using valid and reliable data collection methods ensures fairness and mitigates bias in digital marketing campaigns. Incorporate both quantitative and qualitative approaches to gather comprehensive insights.
Utilize surveys, interviews, and observational studies to capture diverse perspectives from different demographic groups. Implement standardized protocols for data collection to minimize errors and enhance the reliability of the results.
Visual and Verbal Aids
Visual aids such as charts, graphs, and infographics play a vital role in presenting data objectively without introducing discrimination or biases. Ensure that visual representations are clear, concise, and accurately reflect the conveyed information.
Leverage verbal aids such as detailed explanations, annotations, and contextual information to provide additional clarity. By combining visual and verbal aids, marketers can effectively communicate complex data while minimizing the potential for misinterpretation.
Detecting Algorithmic Bias
Data Comparison
To detect algorithmic bias, various data sources must be compared to uncover discrepancies and potential biases. Look for disparities in outcomes based on different input variables.
Seek out inconsistencies in the data that could indicate underlying biases. Utilize techniques such as statistical analysis and machine learning algorithms to identify patterns of bias.
Testing and Validation
Testing and validating data collection tools are crucial steps in detecting algorithmic bias. Regularly assess their performance to ensure accurate and unbiased data collection.
Implement robust validation processes to verify the integrity of the collected data. Conduct thorough tests to evaluate the reliability and fairness of the algorithms used in decision-making.
Expert Feedback and Sensitivity Analyses
Engage with experts in the field to gather valuable feedback on potential biases in the algorithmic decision-making process. Their insights can provide critical perspectives on areas where bias may be introduced.
Conduct sensitivity analyses to assess how changes in input variables impact the algorithm’s outcomes. By systematically varying parameters, you can pinpoint areas where biases are most likely to occur.
Reducing Bias Techniques
Adjusting Methods
Techniques for adjusting data collection methods are crucial to reduce biases in digital marketing. By ensuring diverse representation in training data, companies can mitigate biases effectively. Independent research plays a vital role in identifying and addressing biases in algorithms.
Resampling or weighting data sources is another effective way to mitigate selection bias. By giving more weight to underrepresented groups or samples, companies can improve the overall fairness of their models. This technique helps in creating a more balanced dataset for analysis.
Transforming Data
Transforming or standardizing data is essential to reduce measurement or analysis bias. Through this process, companies can ensure that different variables are on the same scale, preventing any particular feature from dominating the model. Technology and tools play a significant role in automating these processes, making it easier for businesses to implement bias mitigation strategies.
Pros
- Enhances the accuracy and fairness of algorithms.
- Improves decision-making processes by reducing biased outcomes.
Cons
- Requires continuous monitoring and adjustment.
- Implementation may involve additional costs initially.
Importance of Mitigation
Mitigating biases in digital marketing is not just about ethical considerations; it also impacts business performance. Biased algorithms can lead to discriminatory practices, alienating specific customer segments and tarnishing a company’s reputation. Businesses can enhance customer trust and loyalty by employing techniques like resampling, weighting, and data transformation,
Ethical Considerations in AI
Data Analysts
Data analysts play a crucial role in evaluating the fairness of AI algorithms used in digital marketing. They must constantly assess the data inputs and outputs to identify and rectify any biases affecting the decision-making process. By ensuring transparency and accountability, data analysts can create more ethical AI systems.
Consumer Trust
Maintaining consumer trust is paramount in digital marketing. When deployed without adequate ethical considerations, AI technologies can lead to privacy breaches and misuse of personal data. This can result in a loss of consumer trust, damage to the brand’s reputation, and potential legal repercussions.
Brand Reputation
The implications of unethical AI practices on brand reputation cannot be overstated. Brands prioritizing fairness and bias mitigation in their digital marketing strategies are more likely to build a positive brand reputation among consumers. On the other hand, brands associated with biased or discriminatory practices risk alienating their target audience and facing public backlash.
Fairness Vs. Accuracy Trade-offs
Prioritizing Fairness
When prioritizing fairness in digital marketing decision-making, companies often face a trade-off with accuracy. Balancing fairness while maintaining high accuracy levels can be challenging.
Striving for fairness may involve adjusting algorithms to reduce bias toward certain demographics or groups. This adjustment can lower error rates in decision-making processes but might also result in a slight decrease in overall accuracy.
Impact on Marketing Strategies
The focus on fairness can influence how marketing strategies are developed and implemented. By prioritizing fairness, companies aim to ensure their campaigns reach a diverse audience without perpetuating stereotypes or discrimination.
However, this emphasis on fairness could potentially impact the accuracy of targeting efforts. For instance, by avoiding using certain demographic factors in targeting ads, companies may miss out on reaching specific segments of their target audience.
Balancing Fairness and Accuracy
Balancing fairness and accuracy in marketing campaigns requires thoughtful consideration and strategic planning. Companies need to leverage advanced analytics and data-driven insights to identify areas where biases may exist and take proactive steps to mitigate them.
One approach is incorporating feedback loops into decision-making processes to continuously evaluate and refine algorithms for improved fairness outcomes. By regularly assessing the impact of decisions on different user groups, companies can iteratively improve their strategies while minimizing potential biases.
Another strategy involves utilizing various data sources to enhance the diversity of information used in decision-making. This approach helps reduce the reliance on a limited set of variables that may introduce biases and allows for a more comprehensive understanding of customer behavior and preferences.
Pros and Cons
Pros
- Enhanced inclusivity in marketing campaigns
- Reduced risk scores associated with biased decision-making
Cons
- Potential decrease in targeting accuracy
- Increased complexity in algorithm development
Regulatory Measures for Bias
Nondiscrimination Laws
Nondiscrimination laws ensure that digital marketing practices are fair and unbiased. These laws prohibit discrimination based on various factors such as race, gender, age, or disability. By adhering to these laws, companies can create a level playing field for all consumers.
One key benefit of nondiscrimination laws is promoting inclusivity and diversity in marketing campaigns. Companies that embrace these laws are more likely to attract a broader customer base and build trust among diverse audiences. By avoiding discriminatory practices, businesses can enhance their reputation and credibility in the market.
Review
Regular review of marketing strategies is essential to identify and address any biases that may be present. Conducting thorough reviews of advertising materials, targeting criteria, and data analysis processes can help companies detect and rectify any instances of bias. By implementing robust review mechanisms, organizations can ensure that their marketing efforts are inclusive and respectful of all individuals.
By incorporating review processes into their marketing operations, companies demonstrate a commitment to transparency and accountability. This proactive approach helps mitigate biases and fosters a culture of continuous improvement within the organization. Through regular reviews, businesses can adapt to changing consumer preferences and societal norms, staying ahead of potential bias-related issues.
Representative
Ensuring that marketing campaigns represent diverse perspectives is essential for mitigating biases. By featuring a wide range of voices, images, and stories in their advertisements, companies can avoid stereotypes and misconceptions that may perpetuate bias. Including diverse representations in marketing materials promotes fairness and resonates with a broader audience.
Companies that prioritize representative marketing strategies often see increased customer engagement and loyalty. When consumers see themselves reflected in advertisements, they are more likely to connect with the brand on a deeper level. By being representative in their messaging, businesses can foster positive relationships with customers from various backgrounds.
Summary
Understanding and addressing bias in digital marketing is crucial for ensuring fairness and effectiveness in your strategies. By identifying, preventing, and detecting biases in marketing data and AI algorithms, you can enhance the ethical considerations in your AI applications. Balancing fairness and accuracy while adhering to regulatory measures will improve your marketing outcomes and build trust with your audience.
Take proactive steps to mitigate bias in your digital marketing efforts. Stay informed about the latest strategies to reduce bias, prioritize fairness, and comply with regulations. By doing so, you can elevate the impact of your marketing campaigns, foster inclusivity, and establish credibility in an increasingly data-driven landscape.
Frequently Asked Questions
What is digital marketing bias?
Digital marketing bias refers to the unfair advantages or disadvantages that certain groups may face in digital marketing campaigns, iwhich can impactdata analysis and decision-making processes.
How can bias be identified in marketing data?
Bias in marketing data can be identified by thoroughly analyzing demographics, user behavior patterns, and campaign performance metrics to uncover any disparities or inconsistencies.
Why is detecting algorithmic bias significant in digital marketing?
Detecting algorithmic bias is crucial in digital marketing to ensure fair treatment of all individuals, prevent discriminatory practices, and uphold ethical standards in advertising strategies.
What are some techniques for reducing human biases in algorithmic decision-making using training data in big data digital marketing?
TDiversifyingdatasets, implementing transparency measures, conducting regular audits, and utilizing unbiased algorithms for decision-making processes. Are techniques for reducing bias in digital marketing
Why are fairness vs. accuracy trade-offs significant in AI applications for digital marketing involving algorithmic decision-making, big data, deep learning, and training data?
The trade-offs between fairness and accuracy are significant, as achieving perfect accuracy may lead to biased outcomes; this highlights the importance of balancing fairness considerations to ensure equitable results for all target audiences.