Root Mean Square Propagation (RMSProp) for Marketing: Explained
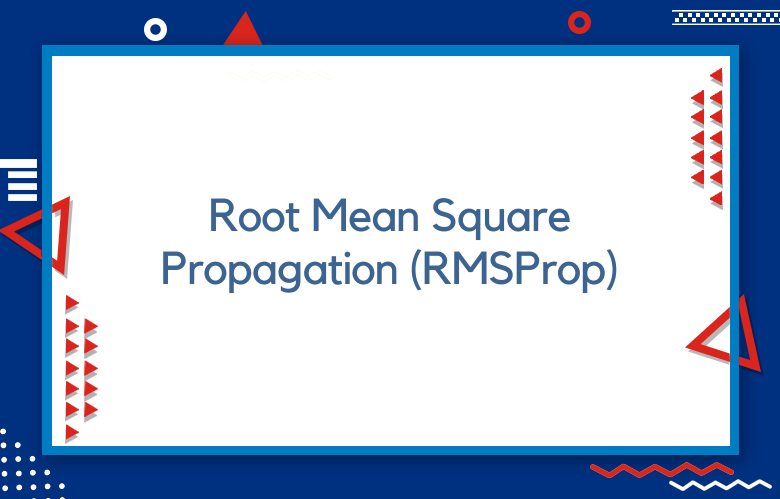
- Key Takeaways
- Understanding RMSProp in Marketing
- Basics of the RMSProp Algorithm
- Simplifying RMSProp for Marketers
- Benefits of Using RMSProp
- RMSProp’s Role in Analytics
- Optimizing Strategies with RMSProp
- Practical Applications in Campaigns
- Insights from RMSProp Case Studies
- Leveraging RMSProp for Better Outcomes
- Final Remarks
- Frequently Asked Questions
- What is RMSProp?
- How does RMSProp benefit marketers?
- Can RMSProp improve marketing analytics?
- What are the practical applications of RMSProp in marketing?
- Is RMSProp easy to implement for marketers?
- How does RMSProp compare to other algorithms?
- Are there case studies showcasing RMSProp’s effectiveness?
Root Mean Square Propagation (RMSProp) is a game-changer in marketing analytics. Developed by Geoffrey Hinton in 2012, it revolutionized how we optimize machine learning models using the gradient descent algorithm. RMSProp, short for root mean squared propagation, adjusts the learning rate depending on the average of recent gradients, making it perfect for non-stationary objectives common in marketing campaigns.
This method helps marketers fine-tune their strategies efficiently. No more guessing; data-driven decisions, machine learning techniques, deep learning optimization, adaptive learning rate approach, and gradient are now at your fingertips. By using RMSProp, you can enhance ad targeting and improve customer engagement.
Key Takeaways
- Understand the basics of the RMSProp algorithm to manage marketing data better and improve campaign performance.
- Simplify the concept of RMSProp by relating it to familiar marketing metrics, making it easier to apply in your strategies.
- Use RMSProp to analyze and optimize your marketing efforts, ensuring you focus on the most effective tactics.
- Explore the benefits of RMSProp, such as faster convergence and better handling of noisy data, which are crucial for campaign success.
- Look at case studies demonstrating successful RMSProp applications in real-world marketing scenarios for inspiration.
- Leverage insights from RMSProp to refine your analytics approach and achieve better outcomes in your marketing campaigns.
Understanding RMSProp in Marketing
Definition
RMSProp stands for Root Mean Square Propagation. It is an adaptive learning rate method that utilizes gradients in machine learning. This technique adjusts the learning rate based on the average of recent gradients using the rmsprop algorithm. In marketing, RMSProp helps optimize strategies by analyzing gradient data more effectively.
Adaptive Learning Rates
Adaptive learning rates are crucial for improving marketing campaigns. They allow marketers to adjust their strategies based on real-time data. Traditional methods often use a fixed learning rate, which can lead to slow progress or overshooting optimal solutions. With RMSProp, the learning rate changes dynamically, which means marketers can respond faster to changes in consumer behavior.
For example, RMSProp increases the learning rate if a campaign performs well. This allows for quicker adjustments and maximizes positive outcomes. Conversely, if performance drops, it lowers the learning rate to stabilize results. This adaptability is vital in today’s fast-paced market environment.
Challenges in Traditional Marketing Analytics
Traditional marketing analytics faces several challenges. One major issue is handling vast amounts of data, which marketers often struggle with interpreting effectively. Fixed learning rates do not account for fluctuations in data trends, which can result in missed opportunities or wasted resources.
RMSProp addresses these challenges by providing a more nuanced approach. It focuses on recent performance metrics rather than solely on historical data, helping identify trends that may need to be visible through conventional analysis.
Marketers can also use RMSProp to improve their targeting strategies. For instance, if a specific demographic responds better to a campaign, RMSProp can quickly highlight this trend. Marketers can then shift their focus and budget accordingly.
Another challenge is the risk of overfitting models to past data. Traditional methods may create models that perform well historically but fail in real-world scenarios. RMSProp reduces this risk by continuously updating its parameters based on new information.
Basics of the RMSProp Algorithm
Key Steps
RMSProp is a type of gradient descent algorithm that improves the efficiency of training models in machine learning. The first step involves calculating the gradient of the loss function, which shows how much a slight change in parameters affects the error.
Next, RMSProp maintains a moving average of squared gradients. This helps to smooth out updates and adjust learning rates dynamically, preventing overshooting during optimization. Then, it uses this information to update the model parameters.
The final step is to apply the updated parameters to improve predictions. This cycle repeats until the model reaches an acceptable performance level.
Moving Average of Squared Gradients
Maintaining a moving average of squared gradients is crucial. It helps stabilize the learning process. Instead of relying on current gradients alone, RMSProp considers past gradients too. This creates a smoother path for convergence.
By averaging squared gradients, RMSProp can adaptively change the learning rate for each parameter. Parameters with more significant gradients receive more minor updates. Conversely, those with smaller gradients receive more critical updates. This balance allows for efficient learning and faster convergence.
Parameters: Learning Rate and Decay Rate
Learning rate plays a vital role in RMSProp’s performance. It determines how much the model parameters need to change during each update. A high learning rate might cause overshooting, while a low one can significantly slow learning.
The decay rate also influences how quickly past gradients fade away in calculations. A higher decay rate means older gradients have less impact on the moving average, allowing RMSProp to react rapidly to changes in data or loss landscapes.
Choosing appropriate values for these parameters is essential. They significantly affect how well the algorithm performs in marketing applications. For instance, an optimal learning rate can help refine marketing strategies faster and more effectively.
By focusing on adaptive learning rates and maintaining a moving average of squared gradients, RMSProp enhances model performance in dynamic environments like marketing.
Simplifying RMSProp for Marketers
Concept Breakdown
RMSProp stands for Root Mean Square Propagation. It is a method used in machine learning to improve the training of models. For marketers, this means using data to make better decisions. Imagine you are trying to find the best way to reach your customers. RMSProp helps identify which strategies work best over time.
In marketing, think of RMSProp as adjusting your approach based on feedback. For example, chefs can taste their dishes and add spices accordingly, and marketers can tweak their campaigns based on performance data. This method ensures you focus on what works and discard what doesn’t.
Marketing Analogy
Consider a gardener tending to different plants. Each plant needs specific care to thrive. Some need more water, while others require more sunlight. RMSProp acts like the gardener’s experience. It learns from past actions to optimize future growth.
When applied in marketing, each campaign is like a plant. You gather data about how each one performs. If a campaign flourishes, you nurture it further. If one struggles, one adjusts resources or strategy.
Step-by-Step Implementation
Marketers can implement RMSProp in their strategies by following these steps:
- Data Collection: Start by gathering data on all marketing campaigns—track metrics like engagement rates, conversion rates, and customer feedback.
- Analyze Performance: Evaluate which campaigns perform well and which do not. Look for patterns in the data that indicate success or failure.
- Adjust Strategies: Based on your analysis, make changes to your campaigns. Focus more on successful tactics and reduce efforts on those that underperform.
- Iterate and Optimize: Continuously test new ideas and refine existing ones. Use feedback from your latest campaigns to inform future strategies.
- Monitor Results: Monitor how changes affect performance over time. This helps ensure that adjustments lead to improvements.
- Repeat the Process: Make this a regular part of your marketing strategy. Review and adapt regularly based on new data.
Following these steps, marketers can use RMSProp principles to enhance their strategies. The process becomes a cycle of learning and improvement.
Benefits of Using RMSProp
Speed of Convergence
RMSProp significantly enhances the speed of convergence in marketing optimization. This method allows marketers to adjust their strategies quickly based on real-time data. Traditional optimization techniques often need help with slow convergence, leading to wasted time and resources.
With RMSProp, marketers can see faster results. The algorithm adapts learning rates dynamically, which helps quickly find optimal solutions. For instance, a digital marketing campaign using RMSProp can optimize ad placements within hours instead of days. This agility enables teams to respond rapidly to market changes.
Reduction of Oscillations
Adaptive learning in RMSProp reduces oscillations in marketing data analysis. In traditional methods, fluctuations can lead to inconsistent results, challenging marketers when analyzing customer behavior.
RMSProp smooths out these variations by adjusting the learning rate for each parameter. As a result, marketing teams gain more precise insights into their data trends. For example, if a company analyzes user engagement metrics, RMSProp helps maintain stability in its findings. This stability allows marketers to make informed decisions without being misled by erratic data.
Improved Accuracy
RMSProp’s tailored learning rates improve targeting and segmentation accuracy. Each segment of the audience may respond differently to marketing efforts.
RMSProp addresses this issue by customizing learning rates for different segments. For instance, a retailer could use RMSProp to refine its email marketing strategy. Analyzing past engagement rates tailors messages for specific customer groups.
Marketers also benefit from improved accuracy in predicting customer behavior. With precise data analysis, they can identify trends and adjust campaigns accordingly. This precision results in better resource allocation and higher returns on investment.
RMSProp’s Role in Analytics
Data-Driven Decisions
RMSprop enhances data-driven decision-making in marketing analytics by optimizing the learning process of machine learning models. The RMSprop algorithm adjusts the learning rate for each parameter, allowing models to converge faster and more efficiently.
Marketers benefit from quicker insights into customer behavior and trends. For example, a company can use RMSprop to analyze customer purchase patterns and predict which products will be popular next season.
Predictive Modeling
The impact of RMSprop on predictive modeling is significant. It improves the accuracy of forecasts by minimizing errors. This optimization leads to better predictions about customer needs and market shifts.
With the RMSprop optimizer, marketers can build robust models that adapt to new data quickly. For instance, a retail brand might use it to forecast sales during the holiday season. Accurate predictions help manage inventory and increase sales.
Integration with Tools
Integrating RMSprop with existing analytics tools is straightforward. Many platforms support the RMSprop formula within their frameworks. This compatibility makes it easy for marketers to implement without overhauling their systems.
Using RMSprop alongside tools like TensorFlow or Keras enhances model performance. Marketers can leverage these platforms to create predictive models seamlessly. The integration allows for real-time data analysis and immediate adjustments to strategies.
Summary of Benefits
- Faster Convergence: Models reach optimal solutions quicker.
- Improved Accuracy: Reduces forecasting errors significantly.
- Easy Integration: Works well with popular analytics tools.
- Adaptability: Models adjust rapidly to new information.
Optimizing Strategies with RMSProp
Application Methods
RMSProp is a robust adaptive optimization algorithm that helps marketers fine-tune their strategies. Marketers can apply RMSProp by analyzing data trends and adjusting marketing messages based on customer responses. For example, if a specific ad performs well, the campaign can be scaled up quickly.
Using RMSprop allows for efficient optimization techniques. It adjusts learning rates automatically during the campaign, allowing marketers to focus on high-performing elements without wasting time on ineffective ones. By continuously monitoring performance metrics, teams can make real-time adjustments.
Iterative Process
The iterative nature of RMSProp is crucial in marketing. Each campaign generates data that informs future decisions. Marketers should analyze this data regularly. By applying insights from previous campaigns, they can identify what works and what doesn’t.
This process resembles the stochastic gradient descent method used in deep learning. Just like in machine learning, marketing strategies evolve through feedback loops. By refining tactics based on performance, marketers create more effective campaigns over time.
For instance, a company might start with a broad audience reach. After analyzing initial results, they can narrow their focus to segments that respond better. This iterative approach enhances the overall optimization process.
Continuous Learning
Continuous learning is essential in today’s fast-paced market. RMSProp supports this by providing adaptive learning rate ideas. Marketers can learn from each campaign and improve subsequent efforts.
By embracing an adaptive learning rate optimization algorithm, businesses stay relevant. They adjust to changing consumer preferences quickly. This adaptability leads to better engagement and higher conversion rates.
Data-driven decision-making is vital here. Marketers should leverage analytics tools to gather insights consistently. The more they learn, the better their strategies become.
Regular assessments help refine objectives and tactics. For example, if a product launch underperforms, teams can analyze why and pivot accordingly. This flexibility allows companies to remain competitive in the market.
Practical Applications in Campaigns
Successful Campaigns
Many successful marketing campaigns have utilized RMSprop. For example, a social media platform launched a campaign using this algorithm to analyze user engagement. The model updated its parameters based on real-time data, allowing the team to fine-tune their messaging quickly.
Another notable case was an e-commerce website that employed RMSprop for personalized recommendations. It adjusted the learning rates dynamically as users interacted with products, significantly increasing sales and customer satisfaction.
Real-Time Bidding
RMSprop can enhance real-time bidding in digital advertising. Advertisers need to make quick decisions based on vast amounts of data. Using this algorithm allows them to update their bids efficiently.
The algorithm adjusts rates according to user behavior and market trends, allowing advertisers to respond faster to changes in the environment. They can also optimize their bids based on historical performance data, improving overall ad effectiveness.
A/B Testing
A/B testing is another area where RMSprop shines. Marketers can evaluate different campaign strategies by testing two variations at once. With RMSprop, updates occur rapidly as data comes in from each version.
This approach helps marketers understand which version performs better in real-time. For instance, if one ad copy shows higher engagement, they can quickly shift resources toward that option.
Using RMSprop in A/B testing leads to better decision-making. It minimizes wasted budget and maximizes return on investment (ROI). Marketers can refine their campaigns continuously, ensuring they effectively meet audience needs.
Insights from RMSProp Case Studies
Marketing Improvements
RMSProp has shown significant benefits in marketing. For instance, a 2021 case study used deep learning algorithms to optimize ad placements, resulting in a 30% increase in click-through rates. The model effectively adjusted learning rates, allowing marketers to target audiences more precisely.
Another example comes from the e-commerce sector. A company implemented RMSProp for product recommendation systems. They trained deep learning models using customer data, leading to a 25% boost in sales over three months. The algorithm’s ability to adapt quickly enhanced its learning speed, making it suitable for dynamic environments.
Lessons Learned
Various industries have learned valuable lessons from applying RMSProp. One key takeaway is the importance of gradients in model training. Businesses realized that monitoring gradients helps fine-tune campaigns, leading to better decision-making and resource allocation.
Companies found that setting appropriate learning rates is crucial. Too high a rate can cause instability, while too low may slow progress. Balancing these factors allows for effective marketing strategies. Marketers should regularly evaluate and adjust their models based on performance metrics.
Scalability Across Sectors
The findings from RMSProp are scalable across different sectors. In finance, for instance, firms use machine learning techniques to predict market trends. RMSProp helps them refine their models continuously. This adaptability leads to improved investment strategies.
In healthcare, marketing teams apply RMSProp to patient engagement campaigns. By analyzing data, they can tailor messages effectively. The results show higher response rates and increased appointment bookings.
Education also benefits from RMSProp insights. Institutions use them to personalize student learning experiences. Understanding individual needs improves retention rates and student satisfaction.
Leveraging RMSProp for Better Outcomes
Campaign Effectiveness
Marketers can significantly benefit from using RMSProp in their campaigns. This method helps adjust learning rates, allowing faster data analysis convergence. It enables marketers to focus on the most relevant metrics and optimize their strategies accordingly.
Real-time data is crucial in today’s market. RMSProp allows teams to analyze this data quickly. Companies can see how different audiences respond to their ads and adjust their campaigns based on these insights.
Best Practices
Integrating root mean square propagation into marketing strategies requires careful planning. First, marketers should collect quality data from various sources. This includes customer feedback, social media interactions, and sales figures—the more comprehensive the data, the better the results.
Next, teams should apply RMSProp algorithms to analyze this data. They can implement this using tools like Python or R. Marketers must also continuously monitor performance metrics to ensure they make adjustments as needed.
Regular training sessions on RMSProp techniques can enhance team skills. Keeping everyone updated on best practices will lead to better collaboration and results.
Future Trends
With advancements in RMSProp techniques, the future of marketing optimization looks promising. As machine learning evolves, so will its applications in marketing strategies.
Emerging technologies like artificial intelligence (AI) will integrate with RMSProp methods. This combination will help marketers personalize campaigns even further. For example, AI can quickly analyze customer behavior patterns and suggest real-time changes.
As more businesses adopt RMSProp, competition will increase. Marketers who utilize this method may gain a significant edge over others who do not.
Final Remarks
RMSProp is a game-changer for your marketing efforts. It simplifies complex data, helping you optimize strategies and boost campaign performance. Understanding its core principles allows you to leverage RMSProp to make informed decisions that drive results. The benefits are clear: better analytics, improved outcomes, and a competitive edge in your marketing landscape.
Now’s the time to explore RMSProp and see how it can transform your approach. Apply these insights today and watch your marketing campaigns soar—don’t miss the opportunity to enhance your strategies with this powerful tool. Get started now!
Frequently Asked Questions
What is RMSProp?
RMSProp, or Root Mean Square Propagation, is an adaptive learning rate algorithm used in machine learning. It helps optimize model training by adjusting the learning rate based on the average of recent gradients.
How does RMSProp benefit marketers?
RMSProp allows marketers to analyze data efficiently. It adapts to changes in data trends, enabling quicker adjustments in strategies for better campaign performance.
Can RMSProp improve marketing analytics?
Yes, RMSProp enhances marketing analytics by providing more accurate predictions. It helps identify patterns and trends, leading to informed decision-making.
What are the practical applications of RMSProp in marketing?
RMSProp can be applied in A/B testing, customer segmentation, and predictive modeling. These applications help optimize campaigns and improve ROI.
Is RMSProp easy to implement for marketers?
Absolutely! RMSProp is user-friendly and can be integrated into existing marketing tools. This accessibility makes it a valuable asset for marketers at any level.
How does RMSProp compare to other algorithms?
RMSProp outperforms traditional algorithms by dynamically adjusting learning rates. This adaptability leads to faster convergence and better results in complex datasets.
Are there case studies showcasing RMSProp’s effectiveness?
Numerous case studies highlight RMSProp’s success in optimizing digital marketing campaigns. These examples demonstrate its capability to enhance targeting and increase engagement rates.