Utilizing Gaussian Mixture Models for Marketing Success
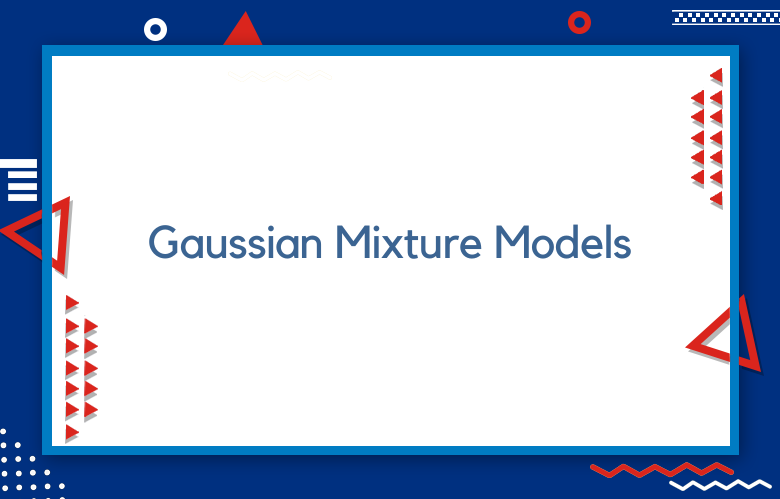
- Key Takeaways
- Understanding Gaussian Mixture Models
- The Role of GMM in Marketing
- Customer Segmentation with GMM
- Enhancing Campaign Strategies
- Comparing GMM with K-Means
- Technical Aspects of GMM
- Implementing GMM for Marketing
- Data-Driven Decisions Using GMM
- Practical Applications in Marketing
- Final Remarks
- Frequently Asked Questions
How can businesses better understand their customers? The answer lies in Gaussian Mixture Models (GMM) for marketing. GMM is a powerful tool that helps marketers segment their audience effectively. It analyzes customer data, revealing hidden patterns and preferences. With this insight, businesses can tailor their strategies to meet specific needs.
Using a GMM model allows companies to identify distinct customer groups. This means more targeted campaigns and improved engagement. By leveraging GMM, marketers can make data-driven decisions that enhance overall performance.
Key Takeaways
- Gaussian Mixture Models (GMM) help marketers better understand customer groups by identifying data patterns.
- Use GMM for effective customer segmentation, allowing for more personalized marketing strategies that resonate with different audiences.
- When comparing GMM to K-Means, remember that GMM can capture more complex data distributions, making it a powerful tool for nuanced analysis.
- Implement GMM in your marketing efforts by starting with clean data and clear objectives to maximize effectiveness.
- Make data-driven decisions by regularly analyzing GMM results and adjusting strategies based on customer behavior trends identified through the model.
Understanding Gaussian Mixture Models
Definition of GMM
Gaussian Mixture Models (GMM) are statistical models. They represent a mixture of several Gaussian distributions, each representing a cluster in the data. The model assumes that all data points are generated from a mix of these distributions, making it robust for understanding complex datasets.
The foundation of GMM lies in probability theory. Each component of the mixture has its mean and variance, which help define the shape of each Gaussian curve. The overall model combines these curves to form a complete picture of the data distribution.
Differences from Other Methods
GMM stands out from other clustering methods like K-means. K-means assigns each data point to the nearest cluster center. It does not consider how closely data points fit into clusters. In contrast, GMM uses probabilities for clustering.
This probabilistic nature allows GMM to handle overlapping clusters better than other methods. For example, if two clusters share some data points, GMM can assign different probabilities to those points based on their position relative to each cluster’s center.
Importance in Data Analysis
GMM is essential in various fields because it can capture complex data distributions. In marketing, businesses analyze consumer behavior using GMM. It helps identify distinct customer segments based on purchasing patterns.
For instance, a retailer may find that customers fall into three main groups: budget shoppers, brand loyalists, and impulse buyers. By understanding these segments, companies can tailor their marketing strategies effectively.
GMM also finds applications in image processing. It has ability to separate different objects in an image by modeling color distributions as Gaussian mixtures. This technique improves object recognition and segmentation tasks.
In finance, GMM aids in risk assessment by modeling asset returns. By identifying underlying patterns, analysts can better understand market behaviors.
Summary of Key Points
- GMM uses multiple Gaussian distributions.
- It provides a probabilistic approach to clustering.
- The model captures complex patterns in diverse fields like marketing and finance.
The Role of GMM in Marketing
Hidden Patterns
GMM can uncover hidden patterns in consumer behavior. Businesses collect vast amounts of data, including customer preferences, purchase history, and demographics. GMM helps analyze this data effectively. It recognizes the clusters of similar behaviors among consumers.
For example, a retailer may find that younger shoppers prefer certain products while older shoppers favor others. They can create specific promotions or advertisements for each group, improving engagement.
Market Trends
GMM is significant for analyzing market trends and segmentation. Marketers need to understand how different segments behave over time. GMM provides a flexible approach to segmenting markets depending on different factors, such as spending habits or product preferences.
In 2020, a study showed that companies using GMM saw better results in targeting their audiences. They could adapt quickly to changes in consumer behavior. This adaptability is crucial in today’s fast-paced market environment.
Predictive Analytics
GMM enhances predictive analytics in marketing strategies. Businesses want to anticipate future consumer actions. GMM allows them to model different scenarios based on historical data, allowing them to predict which products will be popular next season.
For instance, a clothing brand might use GMM to forecast trends for the upcoming summer collection. They analyze past sales data and current fashion trends, and the model helps them decide which styles will sell well.
Market Regimes
Understanding the market regime is vital for businesses. GMM can help identify these regimes by analyzing shifts in consumer preferences and behaviors over time. For example, during economic downturns, consumers may significantly change their buying habits. GMM effectively captures these changes.
A company might notice that sales of luxury items decline during a recession while those of essential goods remain steady. By recognizing these patterns early, businesses can adjust their marketing strategies quickly.
Customer Segmentation with GMM
Behavior Analysis
GMM helps businesses analyze customer behavior. It groups customers based on their purchasing patterns and preferences. For example, a retail store might find that some customers buy high-end products while others prefer discounts. GMM quickly identifies these distinct groups.
By clustering data points, GMM uncovers hidden relationships. Companies can see which features are important to different segments, which leads to a better understanding of customer needs.
Advantages Over Traditional Methods
GMM offers several advantages over traditional segmentation methods. First, it provides a probabilistic approach, which means it considers the uncertainty in data. Conventional methods often assign customers to a single segment, which can be limiting.
Second, GMM adapts well to complex data distributions. It can handle overlapping segments better than older techniques.
Finally, GMM enhances marketing strategies. It enables more precise targeting and personalization. For instance, a company might send different promotions to each customer group based on their likelihood of purchasing specific items.
Real-World Applications
Many businesses successfully use GMM for market segmentation. E-commerce platforms utilize it to recommend products. By analyzing past purchases, they create tailored suggestions for each user.
Banks also apply GMM to identify customer profiles. They segment clients based on spending habits and savings patterns. This helps them design specific financial products that meet diverse needs.
Another example is in the travel industry. Airlines use GMM to segment travelers into leisure and business categories. Each group receives targeted offers that appeal specifically to their interests.
Tailored Marketing Strategies
Using GMM leads to more effective marketing efforts. Companies can develop campaigns that resonate with each segment’s unique preferences. For example, a clothing brand could launch separate advertising campaigns for budget-conscious shoppers and luxury buyers.
This targeted approach increases customer engagement. When customers receive relevant offers, they are more likely to respond positively. As a result, businesses see higher conversion rates and improved customer loyalty.
GMM also allows continuous refinement of strategies. As market trends change, companies can adjust their models accordingly, keeping them ahead of competitors who rely on static segmentation methods.
Enhancing Campaign Strategies
Personalized Campaigns
GMM provides valuable insights into customer behavior. These insights help marketers develop personalized campaigns. By analyzing data, GMM identifies distinct customer groups. Each group has unique preferences and needs. Marketers can tailor messages to resonate with each segment. This personalization increases engagement and conversion rates.
Marketers can explore how different segments respond to various offers. For example, a campaign targeting young adults may focus on social media platforms. In contrast, a campaign for seniors might use email or direct mail.
Resource Allocation
GMM plays a significant role in optimizing resource allocation. Marketers often need more budgets, so they must decide wisely where to invest their resources. GMM helps identify which campaigns yield the best return on investment.
By analyzing past performance, GMM uncovers trends in market sentiment. It reveals which strategies work best under certain conditions. Knowing this allows marketers to adjust their campaigns accordingly.
Effective resource allocation ensures that funds reach the most impactful areas. This approach minimizes waste and maximizes results.
Measuring Effectiveness
Measuring the effectiveness of campaigns informed by GMM analysis is vital. Marketers should establish clear metrics before launching any campaign. These metrics could include click-through, conversion, or customer retention rates.
After a campaign concludes, marketers can analyze the data using GMM models to determine how well the campaign performed across different segments. This analysis provides critical feedback for future efforts.
Marketers should consider using A/B testing as part of their strategy. By comparing two campaign versions, they can see which one performs better. This method allows for real-time adjustments based on market response.
Tracking changes in market sentiment also plays a crucial role in measuring success. Understanding consumer reactions to external factors like inflation or economic shifts informs future campaigns.
Comparing GMM with K-Means
Cluster Shapes
GMM offers more flexibility in defining cluster shapes. It can create elliptical clusters, which means it can adapt to the data’s distribution. This feature is crucial for complex clustering scenarios. K-Means, on the other hand, assumes that clusters are spherical. This rigid shape may only fit some datasets, leading to less accurate clustering results.
Understanding customer segments is vital in marketing. A company using K-Means on customer data that forms elongated or irregular shapes might misrepresent the segments. GMM would better capture these patterns, providing deeper insights into customer behavior.
Probabilistic Vs Deterministic
GMM uses a probabilistic approach to clustering. Each data point is likely to belong to each cluster, allowing for uncertainty and overlapping clusters. As a result, GMM can provide richer information about how customers relate to different segments.
K-Means operates deterministically. It assigns each data point to the nearest cluster center without considering probabilities. This method works well when clusters are well-separated but can fail in cases of overlap. For example, if two customer groups share similar characteristics, K-Means might force them into separate clusters. GMM would recognize their similarities and allow for shared membership.
Performance Scenarios
GMM often outperforms K-Means in various scenarios. It excels when dealing with complex datasets with clusters that overlap or have different shapes. It effectively handles variations in cluster size and density.
For instance, consider a marketing campaign targeting diverse demographics. A company analyzing spending habits may find distinct groups among its customers. Some may be high spenders, while others are budget-conscious. Using GMM allows marketers to identify these nuanced segments more accurately than K-Means could.
Another scenario involves customer preferences that change over time. GMM can adapt as new data comes in, offering continuous insights into shifting trends. K-Means requires re-evaluating cluster centers and may not keep pace with dynamic markets.
In summary, both clustering methods have strengths and weaknesses. GMM provides flexibility and adaptability, which K-Means lacks. Companies can significantly enhance their marketing strategies by choosing the right method based on the dataset’s nature.
Technical Aspects of GMM
Mathematical Principles
GMM relies on a statistical method called expectation maximization (EM). This method helps find the parameters of the GMM by maximizing the likelihood function. The process has two main steps: the expectation step and the maximization step.
In the expectation step, the algorithm calculates the expected value of the log-likelihood function. It uses current estimates of the parameters to determine how likely each data point belongs to each cluster. The maximization step then updates these parameters based on this information. This cycle continues until the model converges to a solution.
Covariance Matrices
Covariance matrices play a crucial role in GMMs. They define the shape and orientation of clusters. Each cluster in a GMM can have its covariance matrix, which allows for different shapes.
For example, a cluster with a diagonal covariance matrix forms an elongated shape along the axes. If it has a full covariance matrix, it can take on any elliptical shape. This flexibility makes GMM more potent than straightforward methods like K-Means, which assume spherical clusters.
Computational Considerations
Implementing GMM requires careful attention to computational aspects. Convergence criteria ensure that the algorithm stops at an appropriate time. Typical criteria include setting a threshold for changes in log-likelihood or limiting the number of iterations.
The choice of initialization also impacts convergence. Poor initialization can lead to local optima rather than finding the best solution. Techniques like k-means clustering can help provide better-starting points for the EM algorithm.
GMM Case Studies
GMM’s real-world applications illustrate its effectiveness in various fields. For instance, in marketing, businesses use GMM to segment customers based on purchasing behavior. By identifying distinct customer groups, companies can tailor their strategies effectively.
Another example is image processing. GMM helps recognize patterns and segment images based on color distributions. This application shows how versatile GMMS can be across different domains.
Advantages and Limitations
GMM offers several advantages over other clustering methods:
- It accommodates clusters of different shapes and sizes.
- It provides probabilistic cluster assignments.
- It can handle overlapping clusters better than K-Means.
However, it also has limitations:
- It may require more computational resources.
- It can be sensitive to initial parameter settings.
- Choosing the right number of components can be challenging.
Implementing GMM for Marketing
Step-by-Step Guide
Implementing a Gaussian Mixture Model (GMM) involves several steps. First, choose a programming language. Python is famous for this task. It has libraries like sci-kit-learn that simplify the process.
- Install Libraries: Use pip to install necessary packages: bash pip install numpy pandas sci-kit-learn
- Load Data: Import your marketing data into Python using pandas. python import pandas as pd data = pd.read_csv(‘marketing_data.CSV)
- Preprocess Data: Clean the dataset by handling missing values and normalizing features.
- Fit the Model: Use the GaussianMixture class from scikit-learn. Python from sklearn.mixture import GaussianMixture gmm = GaussianMixture(n_components=3) gmm.fit(data)
- Predict Clusters: After fitting the model, you can predict cluster memberships. python labels = gmm.predict(data)
- Analyze Results: Evaluate the clustering results to understand different market segments.
Importance of Data Preprocessing
Data preprocessing is crucial for accurate GMM results. Raw data often contains noise and inconsistencies. Cleaning the data improves model performance. Common preprocessing steps include:
- Handling missing values by filling or removing them.
- Normalizing features to ensure they are on similar scales.
- Encoding categorical variables if present.
These steps help in achieving better convergence of the GMM algorithm.
Common Pitfalls
Several pitfalls can occur when implementing GMM models. Recognizing these can lead to better outcomes.
- Choosing the Wrong Number of Components: Selecting too many or too few clusters can misrepresent the data.
- Ignoring Initialization: GMM can converge to local minima based on initial conditions. Run the model multiple times with different initializations.
- Overfitting: A model too complex may fit noise instead of actual patterns. Monitor model performance using validation datasets.
Addressing these issues ensures a robust implementation of GMM in marketing strategies.
Data-Driven Decisions Using GMM
GMM Implementation
GMM implementation plays a crucial role in marketing. It helps businesses analyze large amounts of financial data, which allows marketers to create targeted campaigns and tailor messages effectively by grouping customers into segments.
For example, a clothing retailer may use GMM to understand customer preferences. By analyzing purchase history and online behavior, the retailer can determine which products appeal to different customer groups, leading to more effective advertising strategies.
Forecasting Trends
GMM models excel at forecasting future trends. They rely on historical data to predict customer behavior. Businesses can anticipate changes in market dynamics using these probabilistic models.
Forecasting accurately is vital for maintaining a competitive edge in the market.
Continuous Monitoring
Continuous monitoring of GMM models is essential for relevance. Markets change rapidly, and so do customer behaviors. Regular updates ensure that the model reflects current data dynamics. This process involves re-evaluating data points and adjusting parameters as needed.
For example, a tech company might find that its previous GMM model no longer predicts sales accurately due to new product launches. The company can improve its forecasts by revisiting the model with updated financial data. This adaptability keeps businesses aligned with market demands.
Practical Applications
- Customer Segmentation: GMM helps identify distinct customer segments.
- Product Recommendations: It aids in suggesting products based on user behavior.
- Market Analysis: Businesses gain insights into overall market trends.
These applications demonstrate how GMM transforms raw data into actionable insights. Companies leverage this information to enhance marketing strategies and boost sales.
Practical Applications in Marketing
Real-World Case Studies
Companies use Gaussian Mixture Models (GMM) to improve their marketing strategies. For example, a retail company analyzed customer purchasing patterns and applied GMM to segment customers based on buying behavior. This method helped identify distinct groups, such as bargain hunters and brand loyalists. As a result, the company tailored its promotions to each segment, leading to a 15% increase in sales over six months.
Another case involves an online streaming service that used GMM to analyze viewer habits. By understanding different viewing preferences, the service could be recommended more effectively, improving user engagement and satisfaction. After implementing these changes, the service saw a 20% rise in subscriptions.
Identifying Market Opportunities
GMM helps businesses spot emerging market opportunities. Companies can analyze various economic indicators using this model. For instance, GMM can reveal trends in consumer preferences over time, allowing businesses to adjust their offerings based on these insights.
A tech company recently utilized GMM to explore future market scenarios. They identified potential shifts in technology adoption among consumers. By recognizing these changes early, the company launched products that met new demands. This proactive approach gave them a competitive edge in the market.
Enhancing Customer Experience
Customer experience is vital for business success. GMM can enhance interactions through personalized marketing strategies. By analyzing data from customer feedback, companies can create tailored experiences.
For instance, a travel agency employed GMM to understand client preferences better. They segmented clients based on travel history and interests, which allowed them to send targeted offers that matched individual desires. After implementing these personalized strategies, the agency reported a 30% increase in customer satisfaction.
Market Dynamics and Performance
GMM also aids in understanding market dynamics and performance metrics. Financial markets often show complex behaviors influenced by multiple factors, and GMM can effectively model these conditions.
By applying GMM, analysts can identify specific market regimes. These regimes reflect different market performance periods, such as bullish or bearish trends. Understanding these patterns helps businesses make informed decisions.
Data Analysis with Python
Python is a powerful tool for implementing GMM in marketing analysis. Many data scientists use libraries like Scikit-learn for this purpose. These tools simplify the process of fitting GMMs to large datasets.
Businesses can leverage Python to analyze current market environments quickly. With accurate models, they gain insights into financial trends and market returns. This information supports strategic planning and enhances overall performance.
Final Remarks
Gaussian Mixture Models (GMM) are game-changers in marketing. They help you understand your customers better, target them effectively, and enhance your campaigns. By segmenting data intelligently, GMM empowers you to make data-driven decisions that drive success.
Now is the time to leverage GMM for your marketing efforts. Dive into its practical applications and see how it can transform customer engagement. Start experimenting with GMM today and unlock new opportunities for growth in your marketing campaigns. Your customers deserve it, and so does your business!
Frequently Asked Questions
What are Gaussian Mixture Models (GMM)?
Gaussian Mixture Models are statistical models that represent a data distribution as a combination of multiple Gaussian distributions. They help identify subpopulations within an overall population.
How does GMM benefit marketing?
GMM helps marketers segment customers effectively, allowing for personalized campaigns. By understanding different customer groups, businesses can develop strategies to meet specific needs and preferences.
How does GMM compare to K-Means clustering?
Unlike K-Means, which assigns each data point to a single cluster, GMM allows data points to belong to multiple clusters with varying probabilities. This flexibility often leads to better segmentation.
Can GMM improve customer targeting?
Yes, by using GMM for customer segmentation, marketers can identify distinct groups within their audience. This enables precise targeting and improves the effectiveness of marketing campaigns.
What types of data work best with GMM?
GMM is well-suited for continuous data that exhibits typical distribution characteristics. It performs effectively with large datasets where underlying patterns and groupings exist.
Is implementing GMM difficult?
Implementing GMM can be straightforward with proper tools and libraries like Python’s sci-kit-learn. However, understanding the underlying concepts is crucial for practical application in marketing.
What are the practical applications of GMM in marketing?
GMM can be applied in various areas, such as customer segmentation, anomaly detection, and targeted advertising. Its versatility makes it valuable for enhancing marketing strategies.