Markov Chain Attribution Model for Marketing Mastery
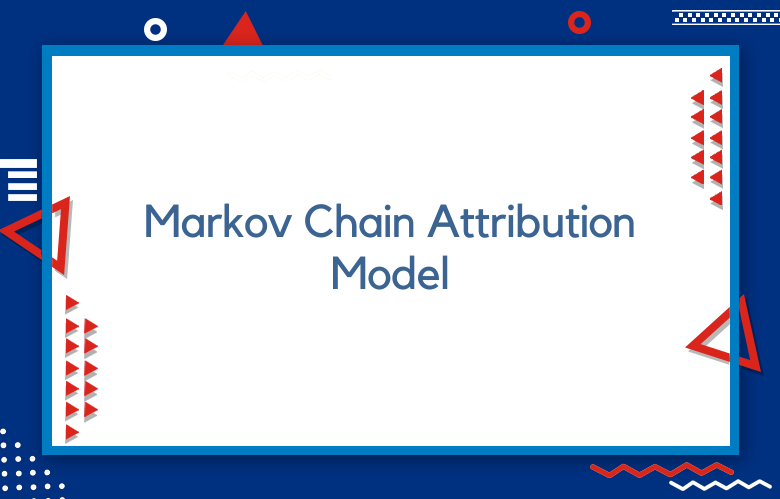
- Key Takeaways
- Basics of Markov Chains
- Key Components of Attribution Models
- Building the Markov Chain Model
- Data Collection and Preparation
- Evaluating Model Effectiveness
- Overcoming Implementation Challenges
- Applications in Marketing
- Benefits of Real-Time Tracking
- Closing Thoughts
- Frequently Asked Questions
- What is a Markov Chain Attribution Model?
- How does a Markov Chain work in marketing?
- What are the key components of an attribution model?
- How do I collect data for a Markov Chain model?
- What challenges might I face when implementing this model?
- How can I evaluate the effectiveness of my Markov Chain model?
- What are the benefits of real-time tracking in attribution models?
Imagine being able to truly measure the impact of your marketing efforts with an efficient attribution model. The Markov Chain Attribution Model for marketing offers this power. This model helps marketers understand customer journeys by analyzing how different touchpoints influence conversions. It goes beyond traditional methods, providing insights into which channels deserve credit for sales using a probabilistic model.
Key Takeaways
- Understand the basics of Markov chains to understand how they can effectively model customer journeys in marketing.
- Focus on key components of attribution models, including touchpoints and conversion paths, to enhance your marketing strategies.
- When building a Markov chain model, ensure accurate data collection and preparation for reliable results.
- Regularly evaluate your model’s effectiveness to refine your marketing efforts and improve ROI.
- Be aware of common implementation challenges and prepare solutions to overcome them for a smoother process.
- Utilize real-time tracking to gain immediate insights into customer behavior, allowing for timely adjustments in your marketing campaigns.
Basics of Markov Chains
Definition
Markov chains are mathematical systems that model sequences of events. They use a set of states and the probabilities of moving from one state to another in a Markov graph. Each event depends only on the previous state, a property known as memorylessness. This means that the probability of transitioning to a future state is independent of the past states, making the model’s predictions solely based on the current state.
Markov chains help understand how different marketing touchpoints influence customer behavior. By analyzing these chains, businesses can see how customers move through their sales funnel using a Markov graph.
Current State Influence
The current state significantly influences the probability of subsequent events in a Markov chain. For example, if customers visit an online store, their following action might depend on whether they viewed a product or added it to their cart.
This dependency allows marketers to predict future actions based on current behaviors. Understanding these probabilities helps marketers tailor their strategies effectively.
Importance of Transitions
Transitions between states are crucial when analyzing marketing touchpoints. Each transition represents a customer’s journey through various interactions with a brand. Recognizing these transitions helps identify which touchpoints are most effective at driving conversions.
For instance, if many customers transition from email campaigns to website visits, email marketing is working well. Marketers can then focus on optimizing these channels further.
Types of Markov Chains
Different types of Markov chains are used in the analysis. Homogeneous Markov chains have constant probabilities for transitions over time. This means the likelihood of moving from one state to another remains the same, regardless of when the transition occurs.
In contrast, order Markov chains consider multiple previous states to determine the next state. This approach can provide more accurate predictions but requires more complex calculations.
Markov Chains Graph
A Markov chain graph visually represents states and transitions. Each node represents a state, while arrows show possible transitions and their probabilities. This graph helps marketers understand customer journeys and identify potential bottlenecks.
Using a graph also allows teams to communicate findings. It provides a straightforward way to showcase how customers interact with different marketing channels.
Attribution Calculations
Markov chain attribution calculations help allocate credit for conversions across multiple touchpoints. Marketers can optimize their strategies by understanding how each interaction contributes to the final decision. This method offers insights into which channels drive the most value.
Key Components of Attribution Models
Essential Elements
Attribution models consist of several vital components. These elements help marketers understand how different channels contribute to conversions. The main parts include touchpoints, weights, and paths. Touchpoints are interactions between customers and brands. Weights assign value to these interactions based on their importance. Paths show the sequence of touchpoints leading to a conversion.
Different attribution models use these components in various ways. For example, a simple attribution model might give all credit to the last touchpoint. In contrast, complex attribution models distribute credit across multiple interactions. This helps marketers see the complete picture of customer behavior.
Role of Touchpoints
Touchpoints play a crucial role in tracking customer journeys. Each interaction can influence a customer’s decision to buy. As a marketer, it’s important to be attentive and identify all relevant touchpoints for practical analysis. These can include social media ads, email campaigns, and website visits.
Understanding how each touchpoint affects conversions is vital. For instance, a customer may see an ad on social media, visit the website, and receive an email before purchasing. By analyzing these interactions, marketers can better allocate resources.
Accurate Data Representation
Accurate data representation is essential in attribution analysis. Without reliable data, it is difficult to assess the effectiveness of marketing efforts. Marketers need to ensure they collect data from all channels accurately.
The current attribution model relies heavily on this data. Algorithmic attribution modeling uses algorithms to analyze large datasets. This approach provides insights into customer behavior that traditional attribution models might miss.
Probabilistic attribution models also require accurate data. They assign probabilities to different touchpoints based on historical data. This method helps marketers understand which channels are most effective over time.
Types of Attribution Models
Marketers can choose from various possible attribution models. Some common types include:
- Simple Attribution Model: Assigns all credit to one touchpoint.
- Complex Attribution Models: Distributes credit among several touchpoints based on their impact.
- Algorithmic Attribution Models: These models use algorithms for deep customer data analysis.
- Traditional Attribution Models: Focuses on first or last-click interactions only.
Each type has its strengths and weaknesses. Choosing the right model depends on business goals and available data.
Building the Markov Chain Model
Steps to Construct
Creating a Markov chain attribution model involves several steps. First, identify all marketing channels, including social media, email, and website visits. Each channel represents a touchpoint in the customer journey.
Next, collect data on customer interactions. Track how customers move between these touchpoints. This data will help build a clear picture of the journey.
After gathering data, create the Markov chain graph. Nodes in this graph represent each touchpoint, and arrows between nodes indicate transitions from one touchpoint to another.
Representing Touchpoints
In the Markov chain models, touchpoints are represented as nodes. Each node captures a specific interaction with the brand. For example, an email click could be one node, and a website visit could be another.
This representation quickly visualizes customer behavior. It shows how customers navigate through different channels before making a purchase.
Calculating Transition Probabilities
Calculating transition probabilities is crucial in Markov chain attribution modeling. Transition probabilities show the likelihood of moving from one node to another.
To calculate these probabilities, divide the number of times a transition occurs by the total number of transitions from that node. For instance, if 20 customers clicked an ad and five went to the website next, the probability is five divided by 20. This results in a transition probability of 0.25 or 25%.
Understanding these probabilities helps marketers see which paths lead to conversions. It reveals the effectiveness of each marketing channel in driving sales.
Hidden Markov Models
Hidden Markov models can add depth to this analysis. They consider not only visible touchpoints but also hidden influences on customer behavior. These hidden states can represent untracked interactions, like word-of-mouth referrals.
Marketers use hidden Markov chain models to gain insights into factors affecting decision-making processes. This approach enhances the understanding of complex customer journeys.
Absorbing Markov Chains
Another important concept is absorbing Markov chains. These chains have at least one absorbing state where customers stay permanently after conversion. This feature helps identify which touchpoints lead to final purchases.
Marketers can focus on optimizing these key touchpoints to improve conversion rates further.
Data Collection and Preparation
Required Data
Markov chain models need specific types of data to work effectively. First, they require historical data on customer interactions. This can be every touchpoint a customer has with your brand. Each interaction should be recorded with details like the channel type and the engagement time.
Next, you need conversion path data. This shows how customers move from one step to another before purchasing. Collecting this data helps in understanding which paths lead to conversions.
Finally, include the total number of leads generated through various channels. This information is crucial for calculations later on.
Data Cleaning
Data cleaning is essential for accurate analysis. Raw data often contains errors or duplicates. Removing these repetitions ensures that your model reflects true customer behavior.
Start by checking for missing values. If certain interactions are unrecorded, fill those gaps if possible. Next, standardize the format of your data. For example, ensure all timestamps are in the same format. Consistent formatting aids in better calculations.
Use tools that automate parts of this process. Many software options can help quickly identify and remove erroneous entries, making the task less tedious and more efficient.
Importance of Historical Data
Historical data plays a significant role in understanding conversion paths. It provides context for current customer behaviors, and by analyzing past interactions, marketers can identify trends over time.
For instance, if a specific channel consistently leads to more conversions, it signals its effectiveness. This insight allows marketers to allocate resources wisely.
Graphs can visualize these trends. They show the relationship between different channels and their impact on sales. A well-prepared report will highlight these insights effectively.
Understanding these patterns helps businesses make informed decisions about future marketing strategies.
Evaluating Model Effectiveness
Assessment Criteria
Markov chain attribution models require specific criteria for effective evaluation. Conversion rates can measure effectiveness, indicating how well a model drives actions. Metrics like click-through rates also provide insight into user engagement.
Another important criterion is the removal effect. This determines how much a marketing channel contributes to conversions when excluded from the model. Marketers can analyze these removal effects to understand each channel’s value.
Comparison with Traditional Models
Markov models differ significantly from traditional attribution methods. Traditional models often use last-click or first-click attribution, which assigns all credit to one interaction, skewing results. Markov chain models distribute probabilities across multiple interactions.
For example, if a customer sees an ad, clicks on it, and receives an email before making a purchase, the Markov model assesses the impact of each touchpoint. This provides a more accurate picture of how channels work together.
Research shows that Markov models can reveal hidden patterns in consumer behavior. They may identify channels that traditional methods overlook.
Continuous Improvement Techniques
Continuous improvement is essential to enhancing the effectiveness of a Markov chain attribution model. Regularly updating data inputs is crucial for accuracy, as fresh data reflects current market conditions and consumer behaviors.
Testing different configurations of the model also proves beneficial. Analysts can adjust variables to see how changes affect results. For instance, altering the time window for data collection can lead to different probabilities and outcomes.
Consider using Shapley value calculations to assess channel contributions. This method evaluates each channel’s impact based on its marginal contribution to total results, providing a fair distribution of credit among channels.
Marketers should also conduct regular reviews of model performance. Analyzing results helps identify strengths and weaknesses in the strategy, allowing teams to pivot quickly if certain channels underperform.
Finally, integrating analytics tools enhances overall understanding. These tools can visualize data trends and highlight key findings. They help marketers make informed decisions based on real-time information.
Overcoming Implementation Challenges
Common Obstacles
Markov chain models can be complex, and many marketers face challenges when implementing them. One common obstacle is data quality. If the data is inaccurate or incomplete, it affects the model’s results. Poor data can lead to wrong conclusions about user interactions and conversions.
Another issue is understanding the algorithm itself. Marketers may struggle with the technical aspects of the model, which can slow down implementation. Teams might also find it hard to track all possible paths users take during their visits. Each touchpoint must be accounted for to represent conversion paths accurately.
Addressing Data Quality Issues
Improving data quality is essential for a successful Markov chain model. First, teams should focus on collecting comprehensive data from all user interactions. This includes tracking every transition a user makes across different channels.
Second, regular data audits are crucial. These audits help identify any inaccuracies or gaps in the information collected. Teams can set up automated systems to flag potential issues in real-time.
Third, investing in training for team members can enhance their data-handling skills. Understanding how to clean and prepare data leads to better model accuracy. By ensuring high-quality input, marketers can trust the algorithm’s outputs.
Collaboration Among Teams
Collaboration plays a vital role in overcoming implementation challenges. Breaking down silos between marketing, IT, and analytics teams can lead to smoother processes. Regular meetings can help everyone stay aligned on goals and expectations.
Creating cross-functional teams allows for shared knowledge and expertise. For instance, marketers can explain their needs while analysts provide insights into data interpretation. This teamwork helps address potential roadblocks early on.
Using project management tools can keep everyone organized. These tools allow teams to track progress and manage timelines effectively. When communicating openly, team members can quickly adapt to changes or challenges.
Applications in Marketing
Campaign Optimization
Markov chain attribution helps businesses optimize their marketing campaigns. It shows how each channel contributes to conversions. For example, a company can analyze data from Google Analytics to see which channels drive the most traffic and sales. This insight allows them to allocate budgets more effectively.
Businesses can also identify weak points in their campaigns. If a channel has low conversion rates, strategies can be adjusted. This might include changing ad placements or targeting different audiences. By understanding these dynamics, companies can improve their overall performance.
Remarketing Strategies
Remarketing is another area where Markov chain attribution shines. Companies can track audience behavior across various channels, such as Facebook and email, and see how often users return to the site before purchasing.
For instance, during the holiday season, a business may notice that users frequently engage with email offers but convert after seeing ads on social media. This information helps craft tailored remarketing strategies, and businesses can focus on specific channels, leading to higher conversions.
Successful Case Studies
Several companies have successfully applied Markov chain attribution in their marketing efforts. One notable case involved a sports apparel brand. They used the model to analyze customer interactions across multiple touchpoints, including social media and email campaigns.
The brand discovered that customers often interacted with Facebook ads before visiting their website. However, they converted after receiving targeted emails.
Another example comes from a vacation rental company. They utilized Markov chain analysis to understand customer journeys during peak booking seasons. The insights revealed that users were more likely to book after engaging with ads on Google and receiving follow-up emails.
By adjusting their marketing mix based on these findings, they improved their conversion rates by 20%. This demonstrates the power of using data-driven insights for effective campaign management.
Data-Driven Decisions
Markov chain attribution provides actionable insights for businesses. Companies can refine their campaigns and enhance user experiences by analyzing customer journeys.
Using this model encourages continuous improvement. Businesses can test new strategies and measure results effectively, which leads to better resource allocation and maximized returns on investment.
Benefits of Real-Time Tracking
Enhanced Insights
Real-time tracking provides immediate insights into marketing campaigns. It allows marketers to track customer behavior as it happens. This means they can see which ads are working and which are not. With this data, they can make quick adjustments. For example, marketers can immediately change the content or budget if an ad is underperforming.
Markov chain models offer a structured way to analyze these behaviors. They help understand how customers move through different touchpoints in their journey. By integrating real-time tracking with these models, marketers gain a clearer picture of customer interactions, leading to better-informed decisions.
Quick Adjustments
Timely data allows for rapid campaign adjustments. Marketers can respond to trends as they emerge. If an unexpected event occurs, such as a viral trend, businesses can adjust their strategies quickly and allocate more budget to successful channels instantly.
For instance, if social media engagement spikes, marketers can increase spending on those platforms. This agility helps maximize the effectiveness of marketing efforts. The ability to adapt ensures that resources are used efficiently.
Improved Decision-Making
Real-time data enhances decision-making processes. Marketers can rely on current information rather than outdated reports. This leads to more accurate forecasting and planning. Decisions based on real-time data tend to be more effective.
With Markov chain models, marketers can analyze various scenarios dynamically and predict how changes will affect outcomes in real-time. This predictive capability supports smarter strategic moves.
Maximizing Marketing Effectiveness
Timely insights from real-time tracking significantly impact marketing success. Businesses that use these insights effectively see higher returns on investment (ROI). They can identify which channels drive conversions and focus their efforts there.
By better understanding customer paths, companies can optimize their marketing funnels. When customers feel understood, they are more likely to convert.
Marketers also benefit from identifying patterns over time. Analyzing these patterns helps in crafting future strategies. Companies that leverage real-time tracking with Markov chain models often outperform competitors who do not.
Closing Thoughts
The Markov Chain Attribution Model offers a fresh perspective on tracking marketing efforts. By better understanding customer journeys, you can allocate resources more effectively. This model helps you see which channels drive results and enhance decision-making.
Embracing this approach means staying ahead in the fast-paced marketing world. Real-time tracking boosts your strategy, allowing you to adapt quickly. Don’t miss out on the opportunity to leverage this powerful tool for your campaigns. Start exploring Markov Chains today to unlock your marketing potential!
Frequently Asked Questions
What is a Markov Chain Attribution Model?
A Markov Chain Attribution Model assigns credit to marketing channels based on their contribution to customer journeys. It analyzes user interactions and transitions between channels, providing insights into how each channel influences conversions.
How does a Markov Chain work in marketing?
A Markov Chain models customer behavior as a series of states, each representing a touchpoint. The model calculates transition probabilities, helping marketers understand the likelihood of customers moving from one channel to another.
What are the key components of an attribution model?
Key components include touchpoints, conversion events, and transition probabilities. These elements help analyze how marketing channels interact and contribute to overall performance.
How do I collect data for a Markov Chain model?
Data collection involves tracking user interactions across various channels. Analytics tools gather information on clicks, impressions, and conversions to create a comprehensive dataset for analysis.
What challenges might I face when implementing this model?
Common challenges include data quality issues, integrating existing systems, and ensuring accurate tracking across multiple channels. Addressing these challenges requires careful planning and robust data management strategies.
How can I evaluate the effectiveness of my Markov Chain model?
Evaluate effectiveness by comparing predicted outcomes with actual results. Use metrics like conversion rates and return on investment (ROI) to assess the model’s impact on marketing performance.
What are the benefits of real-time tracking in attribution models?
Real-time tracking of immediate insights into customer behavior. It allows marketers to adjust strategies swiftly, optimize campaigns effectively, and enhance decision-making based on current data trends.