Non-Negative Matrix Factorization (NMF) for Marketing: Applications
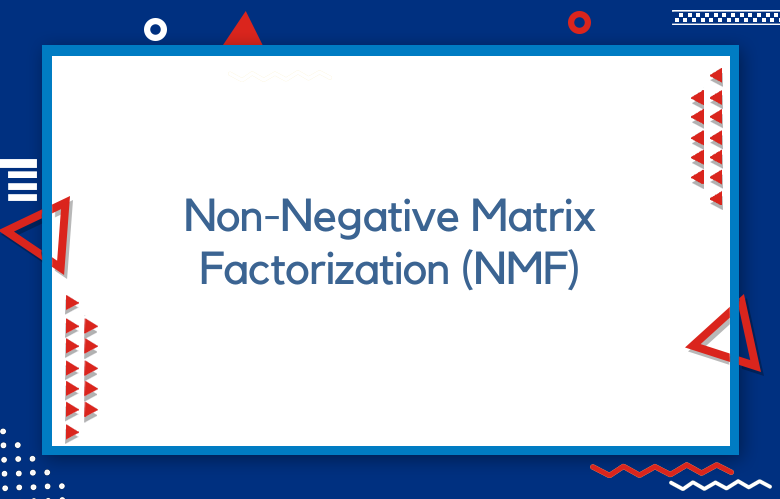
- Key Takeaways
- Understanding Non-Negative Matrix Factorization
- NMF in Marketing Applications
- Segmentation Strategies with NMF
- Recommendation Systems Using NMF
- Implementing NMF in Marketing Projects
- Optimizing NMF Techniques for Better Results
- Challenges and Solutions in NMF
- Future Trends in NMF for Marketing
- Summary
- Frequently Asked Questions
- What is Non-Negative Matrix Factorization (NMF)?
- How is NMF used in marketing?
- What are the benefits of using NMF for segmentation?
- Can NMF improve recommendation systems?
- What challenges are associated with implementing NMF?
- How can I optimize NMF for better results?
- What are future trends in NMF for marketing?
Did you know 70% of marketing efforts fail due to poor data analysis? Non-negative matrix factorization (NMF) for marketing can change that. NMF helps businesses uncover hidden patterns in data, leading to more intelligent decisions. It breaks down complex information into simpler parts, making understanding customer preferences and behaviors easier. This powerful tool can enhance targeted campaigns and improve customer satisfaction.
With NMF, marketers can identify key factors driving sales and tailor their strategies accordingly. It’s all about maximizing results with minimal effort. Dive into how NMF transforms marketing strategies and boosts your business’s performance.
Key Takeaways
- Non-negative Matrix Factorization (NMF) is a powerful tool for uncovering patterns in marketing data, helping businesses understand customer behaviors and preferences.
- Use NMF for segmentation by identifying distinct customer groups, which can lead to more targeted and effective marketing strategies.
- To successfully apply NMF in marketing projects, you must have clean data and a clear goal for the analysis.
- Optimize NMF techniques by regularly updating your models and incorporating new data to keep your marketing strategies relevant and effective.
- Stay informed about future trends in NMF, as advancements can provide new opportunities for improving marketing effectiveness and customer engagement.
Understanding Non-Negative Matrix Factorization
Definition and Significance
Non-negative matrix factorization (NMF) is a matrix factorization method that breaks down a large matrix into two smaller matrices with non-negative elements. This method is useful in data analysis across many fields, including marketing, where companies can analyze customer behavior.
NMF helps reveal patterns in large datasets. For example, it can find hidden features in product reviews or social media interactions.
Unique Characteristics for Marketing
NMF has unique traits that suit marketing applications well. One key feature is the non-negativity constraint. All elements in the matrices must be zero or positive. This mirrors real-world situations where negative values, like sales figures or customer counts, do not make sense.
Another advantage of NMF is its ability to produce collective factorization. This means it can identify common themes across different datasets. For instance, a company can analyze customer feedback from various sources and find shared sentiments about products. This collective insight allows marketers to focus on what truly matters to customers.
Mathematical Foundation
The mathematical foundation of NMF relies on the concept of matrix factorization. The goal is to approximate an original data matrix by multiplying two smaller matrices: W (the basis matrix) and H (the coefficient matrix). The equation looks like this:
W contains the factor matrices in this equation, while H represents the coefficients that combine these factors.
The factorization rank determines how many features to extract from the original data matrix. Choosing an appropriate rank is crucial for effective results. A low rank may oversimplify the data, while a high rank can lead to overfitting.
The non-negativity constraint ensures that all elements in W and H remain non-negative. This characteristic makes NMF particularly effective in marketing tasks like topic modeling or customer segmentation.
Applications and Examples
Marketers use NMF for various applications:
- Customer Segmentation: Identifying distinct groups within a customer base.
- Recommendation Systems: Suggesting products based on user preferences.
- Sentiment Analysis: Understanding customer feelings towards products or services.
These applications show how NMF transforms raw data into actionable insights for businesses.
NMF in Marketing Applications
Marketing Tasks
NMF can be used in various marketing tasks. It helps in customer segmentation. Marketers can identify distinct groups with similar behaviors and preferences by analyzing customer data, allowing for targeted marketing campaigns.
NMF also aids in product recommendation systems. Companies can recommend products based on user behavior. The NMF model uncovers hidden patterns within user-item interactions, leading to more relevant customer suggestions.
Data-Driven Decisions
NMF enhances data-driven decision-making in marketing strategies. Marketers can analyze large datasets quickly and effectively, extracting meaningful insights from complex data structures. The NMF object simplifies this process by breaking down data into interpretable components.
This method enables marketers to prioritize their efforts. They can focus on high-impact areas identified through NMF embeddings. For example, a company may discover that certain demographics respond better to specific promotions. This knowledge guides campaign adjustments and resource allocation.
Customer Engagement
NMF plays a crucial role in optimizing customer engagement and targeting. It allows businesses to understand customer preferences deeply. By using the NMF class, companies can tailor their messages to fit different segments.
For instance, a retailer might find that younger consumers prefer eco-friendly products. With this insight, they can create targeted ads emphasizing sustainability, which increases the chances of effectively engaging customers.
Furthermore, NMF helps measure campaign effectiveness. Marketers can evaluate how well their strategies resonate with the target audience and adjust their tactics based on real-time feedback from NMF analysis.
Use Cases
- Customer Segmentation: Identifying distinct customer groups for targeted marketing.
- Recommendation Systems: Suggesting products based on user behavior.
- Campaign Optimization: Adjusting marketing strategies based on performance metrics.
- Preference Analysis: Understanding customer likes and dislikes for better targeting.
- Trend Analysis: Detecting emerging trends within consumer behavior.
Segmentation Strategies with NMF
Cluster Assignment
NMF helps to identify cluster assignments by breaking down enormous datasets into smaller, manageable segments. The W matrix from NMF represents the relationship between customers and features. Each row in this matrix corresponds to a customer, while each column represents a feature or characteristic. Marketers can see which features are most relevant for different customer groups by analyzing the values in the W matrix.
Interpreting the W matrix allows marketers to derive actionable customer segments. For instance, a high value in a specific column indicates that a customer is strongly associated with that feature. This insight helps businesses understand what drives customer behavior.
Importance of Segment Analysis
Segment analysis is crucial for tailoring marketing efforts. It allows businesses to analyze their customers better, leading to more personalized marketing campaigns.
For example, if one segment shows high interest in eco-friendly products, marketing efforts can highlight sustainability. Another segment may prefer luxury items, allowing businesses to adjust their messaging accordingly. Companies that align marketing strategies with customer preferences increase engagement and drive sales.
Visualizing Segment Characteristics
Visualizing segment characteristics derived from NMF enhances understanding and communication. Various techniques exist for visual representation. Heatmaps are effective for displaying the W matrix. They show how different features relate to each customer segment visually.
Another technique is using cluster plots. These plots allow marketers to see how different segments compare against one another based on selected features. Such visual tools help teams identify trends and patterns quickly. Marketers can easily share these insights across departments, ensuring everyone understands the target audience.
Moreover, dashboards can provide real-time updates on segment performance. Companies can track how well their tailored marketing efforts are performing over time. This ongoing analysis helps refine strategies further and adapt to changing market conditions.
Recommendation Systems Using NMF
Building Steps
Creating a recommendation system with non-negative matrix factorization (NMF) involves several key steps. First, data for different products, including user ratings, should be gathered. This data forms a matrix where rows represent users and columns represent items.
Next, apply NMF to this matrix. The algorithm decomposes the original matrix into two smaller matrices. One represents user preferences, while the other shows item characteristics. After that, reconstruct the original matrix using these two new matrices. This reconstruction helps to predict missing ratings.
Finally, the reconstructed matrix will be used to generate product recommendations. Businesses can offer personalized suggestions by identifying items with high predicted ratings for each user.
Improving Accuracy
NMF enhances the accuracy of product recommendations by focusing on shared features among items. Traditional methods often need help with sparse data, where many users do not rate all products. NMF effectively addresses this issue.
For instance, if a user rates several action movies highly, NMF identifies common traits in those films and suggests other action movies with similar features. This method increases the likelihood that users will enjoy the recommended products.
Moreover, NMF minimizes noise in the data. It filters out irrelevant information and focuses on significant patterns in user behavior. As a result, consumers receive better-targeted recommendations that align with their interests.
Collaborative Filtering Impact
Collaborative filtering plays a vital role in enhancing user experience through personalized suggestions. This technique relies on user interactions rather than item characteristics alone. When combined with NMF, collaborative filtering becomes even more powerful.
By analyzing how similar users rate products, businesses can recommend items others liked but the user has yet to discover. For example, consider User A and User B have identical tastes in music. User A enjoys a specific album that User B hasn’t listened to yet, so the system can recommend that album to User B.
This approach creates a sense of community among users. It encourages the exploration of new products based on trusted peer opinions. Users feel more engaged when they receive tailored suggestions that reflect their preferences.
In summary, NMF significantly improves recommendation systems by accurately predicting ratings and enhancing collaborative filtering techniques. These advancements lead to better user experiences and increased satisfaction with product offerings.
Implementing NMF in Marketing Projects
Framework for Integration
Integrating non-negative matrix factorization (NMF) into marketing workflows requires a clear framework. Start by defining the marketing goals. Identify what insights you want from your data. For instance, do you aim to understand customer preferences or improve product recommendations?
Next, gather relevant data, including purchase history and user interactions. Create a product matrix representing items and their features. This matrix serves as the foundation for the NMF model.
After building the matrix, implement the NMF algorithm. Use appropriate software tools like Python with libraries such as Scikit-learn. The output will be two matrices: one representing users and another representing products. These matrices help identify patterns and relationships within the data.
Data Preparation Best Practices
Data preparation is crucial before applying NMF. First, clean the data to remove any inconsistencies. Ensure that all entries in the set are complete and accurate. Missing values can distort results.
Next, normalize the data. Scaling helps maintain uniformity across different features, ensuring no single feature disproportionately influences the model.
It’s also essential to transform categorical variables into numerical formats. For example, convert product categories into numbers so the model can process them effectively.
Finally, split your dataset into training and testing sets. This division allows for a better evaluation of the NMF model’s performance later.
Continuous Evaluation
Continuous evaluation of NMF models is vital in marketing projects. Regularly assess how well the model performs against set benchmarks. Use metrics like precision and recall to measure accuracy.
Gather feedback from users on the model’s recommendations. This feedback loop offers insights into areas needing improvement.
Based on this evaluation, refine the model. Update your product matrix regularly to reflect changes in inventory or customer behavior. This keeps the NMF model relevant and effective over time.
In summary, implementing NMF in marketing involves a structured approach that goes from integration to continuous assessment. Following best practices in data preparation ensures reliable outcomes, and regular evaluation allows marketers to adapt strategies based on real-world performance.
Optimizing NMF Techniques for Better Results
Advanced Techniques
Several advanced techniques can enhance the performance of non-negative matrix factorization (NMF) algorithms. One effective method is vectorization. This approach transforms data into a format that algorithms can process more efficiently. By using vectorization, NMF can handle larger datasets with improved speed.
Another advanced technique involves dimensionality reduction. Reducing dimensions helps focus on essential features while minimizing noise, leading to more accurate NMF results. It also simplifies the target matrix, making it easier to analyze.
Parameter Tuning
Parameter tuning is vital in determining the quality of segmentation and recommendations. Adjusting parameters such as the number of components can significantly impact outcomes. More components may capture more detail but can also lead to overfitting.
Finding the right balance is crucial. Tools like grid search can help identify optimal settings. This method systematically tests various combinations of parameters. The results guide marketers toward better decisions based on data-driven insights.
Hybrid Models
Hybrid models combine NMF with other analytical methods for improved outcomes. For instance, integrating hierarchical clustering with NMF can enhance segmentation. Hierarchical clustering organizes data as a tree-like structure, revealing relationships between clusters.
This combination allows marketers to understand customer groups better. They can use insights from both methods to tailor marketing strategies effectively. Combining NMF with machine learning algorithms can yield powerful predictive models.
Marketers can also explore using distance metrics in conjunction with NMF. Metrics like divergence distance loss serve as crucial metrics for assessing model performance. Marketers can refine their strategies by analyzing how well the model fits the data.
Algorithms and Implementation
Implementing several algorithms alongside NMF can provide diverse perspectives on data analysis. For example, traditional clustering methods may offer insights that complement NMF findings. Utilizing libraries like NumPy makes it easier to execute these algorithms efficiently.
The dot product is another crucial aspect in this context. It helps calculate similarities between data points and components effectively. Understanding these relationships aids in creating more targeted marketing campaigns.
Optimizing NMF techniques involves exploring advanced methods, fine-tuning parameters, and adopting hybrid models. Each step contributes to achieving better results in marketing projects.
Challenges and Solutions in NMF
Common Pitfalls
NMF faces several challenges in marketing applications. One major issue is data independence. NMF assumes that data points are independent of one another. However, in marketing, this is often different. Customers may influence each other’s behavior. This can lead to misleading results.
Another pitfall is dealing with missing values in datasets. Marketing data often needs more surveys or tracking issues. Missing data can skew the results of NMF analysis and lead to incorrect conclusions about customer preferences and behaviors.
Addressing Data Issues
Marketers should consider using constraints in their NMF models to tackle data independence issues. Constraints can help account for relationships between data points, and regularization techniques can improve model accuracy.
Handling missing values requires different strategies. One effective method is imputation. This involves filling in missing values based on existing data patterns. Techniques like mean imputation or k-nearest neighbors can be useful here. Another option is to use algorithms designed to handle missing data directly.
Alternative Algorithms
While NMF is powerful, there are better fits for some situations. In some cases, other algorithms may work better. For instance, Principal Component Analysis (PCA) can be a good alternative. PCA reduces dimensionality and helps visualize complex datasets. It works well when data independence is less of a concern.
Another option is Latent Dirichlet Allocation (LDA). LDA focuses on topic modeling and can reveal hidden themes within marketing texts or reviews.
Using these alternatives alongside NMF can enhance marketing strategies. Combining methods allows marketers to gain a more comprehensive understanding of their audience.
Future Trends in NMF for Marketing
Machine Learning Impact
Advancements in machine learning will change how non-negative matrix factorization (NMF) is used in marketing. As algorithms improve, NMF can analyze data faster and more accurately. This speed allows marketers to gain insights in real time.
ML models can learn from new data patterns and adapt and refine their predictions. This adaptability enhances the effectiveness of NMF applications. For example, marketers can use NMF to segment customers based on behavior. Improved algorithms will provide deeper insights into customer preferences.
New Marketing Areas
NMF could enter new marketing areas as it evolves. One area is personalized advertising, where marketers want to create ads tailored to individual needs. NMF can help identify customer segments for targeted campaigns.
Another area is social media analytics. With the rise of social media, brands need to understand audience engagement. NMF can analyze interactions and sentiments effectively. It can help brands discover trends and optimize content strategies.
NMF may also find its place in product recommendation systems. Analyzing user data can suggest products that fit individual tastes. This application increases customer satisfaction and sales.
Big Data and AI Implications
Big data plays a crucial role in the future of NMF techniques. The amount of data generated daily is enormous, and marketers need tools to process this data efficiently. NMF helps manage large datasets by breaking them down into smaller parts.
Artificial intelligence (AI) will further enhance NMF capabilities. AI can automate the analysis of complex datasets, saving marketers time and resources.
The combination of big data and AI will lead to better decision-making processes. Marketers will rely on accurate insights derived from NMF analysis to guide strategies like pricing, promotions, and inventory management.
As companies embrace these technologies, they will stay competitive in the market.
Summary
Non-negative matrix factorization (NMF) opens doors for innovative marketing strategies. You’ve learned how NMF can enhance segmentation, improve recommendation systems, and optimize marketing projects. This powerful technique helps you make data-driven decisions that resonate with your audience.
Embrace NMF to tackle challenges and stay ahead of future marketing trends. You can refine your strategies by leveraging its capabilities and drive better results. Dive deeper into NMF and transform your marketing approach today. Your audience is waiting for insights that only you can provide.
Frequently Asked Questions
What is Non-Negative Matrix Factorization (NMF)?
NMF is a mathematical technique that decomposes a nonnegative matrix into two lower-dimensional nonnegative matrices. It helps discover hidden patterns in data, making it useful for various applications, including marketing.
How is NMF used in marketing?
In marketing, NMF identifies customer segments, analyzes purchasing behavior, and enhances recommendation systems. It allows businesses to tailor strategies based on insights derived from consumer data.
What are the benefits of using NMF for segmentation?
NMF provides clear and interpretable segments by revealing underlying factors driving customer behavior. This clarity aids marketers in creating targeted campaigns that resonate with specific audience groups.
Can NMF improve recommendation systems?
Yes, NMF enhances recommendation systems by uncovering latent features in user-item interactions. This leads to more personalized recommendations, increasing customer satisfaction and engagement.
What challenges are associated with implementing NMF?
Common challenges include selecting the right number of components and dealing with data noise. However, these can be addressed through careful preprocessing and validation techniques.
How can I optimize NMF for better results?
To optimize NMF, experiment with different initialization methods, regularization techniques, and convergence criteria. Fine-tuning these parameters can enhance the quality of the factorization.
What are future trends in NMF for marketing?
Future trends include integrating NMF with machine learning models and leveraging big data analytics. These advancements will enable more profound insights into consumer behavior and effective marketing strategies.