Bayesian Optimization Algorithm for Marketing: Key Concepts
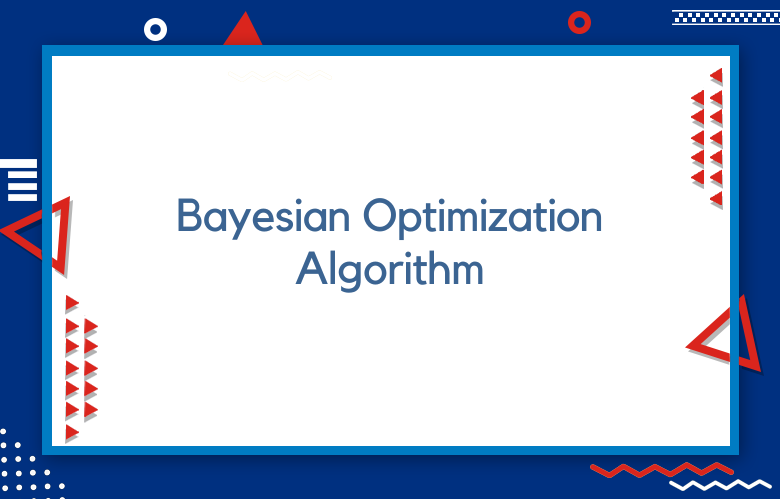
The marketing world is evolving quickly. Traditional methods often need to be revised in today’s fast-paced environment. Enter the Bayesian optimization algorithm for marketing. This tool helps businesses make data-driven decisions and optimize their campaigns effectively. It contrasts with old-school techniques that rely on guesswork and intuition.
With Bayesian Optimization, marketers can identify the best strategies and allocate resources wisely. This approach not only saves time but also boosts ROI. By leveraging past data, it makes accurate and actionable predictions. Embracing this algorithm can transform your marketing efforts and set you apart from competitors stuck in the past.
Key Takeaways
- Bayesian Optimization can significantly improve marketing strategies by helping you make data-driven decisions based on prior information and likelihood analysis.
- Understand the fundamental concepts of Bayesian analysis to effectively apply them in your marketing efforts, ensuring you grasp how prior information influences outcomes.
- Follow a structured approach, using the Bayesian process, to systematically refine your marketing tactics and enhance their effectiveness over time.
- Leverage prior information about customer behavior and market trends to inform your marketing campaigns, making them more targeted and successful.
- Use likelihood exploration to analyze different marketing scenarios. This can provide better insights and improved click-through rates (CTR).
- Implement practical applications of Bayesian methods in your marketing strategy to optimize resources and maximize returns on investment.
Understanding Bayesian Optimization
Definition
Bayesian Optimization is a method for optimizing complex functions. These functions can be expensive to evaluate. For example, testing different marketing strategies can take time and resources. Instead of assessing every option, this method uses past data to make intelligent decisions.
Relevance in Marketing
This optimization technique is crucial in marketing. It significantly improves decision-making processes. Companies can use it to find the best marketing strategies quickly. By focusing on the most promising options, businesses save time and money.
For instance, a company launching a new product can test various advertising channels. They may consider social media, TV ads, or email campaigns. Using Bayesian Optimization, they analyze previous campaign data.
Probabilistic Models
Bayesian Optimization relies on probabilistic models that predict how well different strategies might perform. It then gathers data from evaluations to update its predictions.
The process involves two main steps: exploration and exploitation. Exploration means trying out new options that may yield better results. Exploitation focuses on refining the best-known options. This balance allows marketers to discover optimal solutions efficiently.
For example, a business may want to optimize its email marketing campaign. They start by sending emails with different subject lines and content styles. The algorithm collects data on open rates and conversions. Based on this data, it adjusts future emails to improve performance.
Benefits of Bayesian Optimization
- Cost-effective: Reduces unnecessary evaluations.
- Time-saving: Quickly identifies optimal solutions.
- Data-driven: Uses existing data for better predictions.
- Flexible: Adapts to various marketing challenges.
Marketers can focus on strategies that show promise based on historical data and trends.
Real-World Examples
Many companies have adopted Bayesian Optimization in their marketing strategies. Google, for instance, uses this method for ad placement optimization.
Another example is Airbnb, which employs Bayesian methods to optimize pricing strategies for hosts. They adjust prices based on demand forecasts and market conditions using previous booking data.
Critical Concepts in Bayesian Analysis
Gaussian Processes
Gaussian Processes (GPs) serve as a foundational element of Bayesian Optimization. They model the unknown function being optimized. GPs provide a flexible way to predict outcomes based on observed data. Each prediction comes with an estimate of uncertainty. This is crucial because it helps marketers understand how confident they can be about their decisions.
Marketers use GPs to explore different strategies. For instance, if a company tests an advertisement’s effectiveness, GPs can help predict its performance across various audiences. The model learns from the results and adjusts predictions accordingly. This iterative process leads to improved marketing strategies over time.
Acquisition Functions
Acquisition functions play a vital role in guiding the optimization process. They determine which points in the search space should be evaluated next. By balancing exploration and exploitation, acquisition functions help find better solutions efficiently.
Standard acquisition functions include Expected Improvement (EI) and Upper Confidence Bound (UCB). EI focuses on points that could improve the current best outcome. UCB emphasizes areas with high uncertainty, allowing for exploring less-tested strategies.
Using these functions, marketers can effectively prioritize efforts. For example, acquisition functions will guide further testing in the uncertain area if a campaign shows potential in one area but lacks data in another. This targeted approach maximizes resource efficiency.
Uncertainty Quantification
Quantification of uncertainty is essential for making informed marketing decisions. It allows marketers to assess risks associated with various strategies, and understanding uncertainty helps teams avoid costly mistakes.
For instance, marketers often face unknowns about customer reactions when launching a new product. Bayesian Optimization provides insights into these uncertainties by using GPs. Marketers can visualize potential outcomes along with their probabilities, aiding decision-making.
Moreover, quantifying uncertainty helps set realistic expectations. If a marketing strategy has high uncertainty, teams can prepare for varying outcomes. This preparation minimizes surprises and fosters resilience.
Steps in the Bayesian Process
Define Objective
The first step in Bayesian Optimization is to define the objective function. This function measures what you want to optimize. For example, if a company wants to increase sales, the objective might be total revenue.
This function needs clear parameters and should reflect the marketing strategy’s goals. Determining this function helps focus on what truly matters for success.
Update Model
After defining the objective, the next step involves updating the model with new data. This process is iterative. Each time new information comes in, predictions are refined.
For instance, if a marketing campaign runs, data on customer responses becomes available. This data allows marketers to adjust strategies based on actual performance. The model learns from past actions and adapts accordingly.
Using statistical methods, the algorithm updates its understanding of the objective function. It evaluates how changes impact results. This continuous learning enhances future predictions.
Evaluate Acquisition Function
Evaluating the acquisition function is crucial in Bayesian Optimization. This function determines where to sample next for evaluation. It guides decision-making by identifying points that could yield better results.
Marketers use the acquisition function to find optimal areas for testing. For example, they might explore different advertising channels or target audiences. The goal is to discover which options perform best.
The acquisition function balances exploration and exploitation. It encourages trying new strategies while also refining known successful ones. By evaluating this function regularly, marketers can stay ahead of trends.
Iterate Process
The process is not linear; it requires multiple iterations. After each evaluation, insights are gathered and incorporated into the model, leading to more informed decisions.
Every iteration helps narrow down effective strategies. Marketers can tweak their approaches based on fresh data and insights gained from previous rounds.
This iterative nature makes Bayesian Optimization powerful in marketing contexts. It allows for a responsive approach that adjusts as conditions change.
Role of Prior Information
Importance of Prior Beliefs
Prior beliefs play a significant role in the Bayesian optimization algorithm. They provide a starting point for the analysis based on previous data or expert insights. By incorporating this information, marketers can guide the optimization process more effectively and make informed decisions rather than relying solely on new data.
Bayesian methods treat prior information as a probability distribution, which helps in modeling uncertainty. As new data comes in, these prior beliefs are updated. This updating process is essential for refining marketing strategies.
Influence on the Optimization Process
Prior information significantly influences the optimization outcomes. If the previous is strong, it can lead to faster convergence towards optimal solutions. For instance, if a company knows that a particular advertising channel performs well, it can prioritize it early. This focus can save time and resources during campaigns.
However, over-relying on prior beliefs can be risky. If the previous is accurate, it may lead to better decisions. Marketers must regularly assess their prior assumptions against actual performance data. Balancing prior knowledge with new evidence is vital for success.
Balancing Knowledge and Evidence
Finding the right balance between prior knowledge and new evidence shapes effective marketing strategies. Marketers should view their prior beliefs as flexible guidelines rather than fixed rules. When new data emerges, they should adjust their strategy accordingly.
For example, if initial marketing efforts yield unexpected results, it’s essential to re-evaluate both the prior information and the new evidence. Adjustments based on fresh insights can improve future campaigns. This adaptability is what makes Bayesian Optimization powerful.
The dynamic nature of markets requires constant learning and adaptation. Marketers should embrace changes in consumer behavior or market conditions. They enhance their decision-making processes by integrating new findings into their existing frameworks.
Exploring Likelihood in Analysis
Definition of Likelihood
Likelihood measures how well a model explains observed data. In Bayesian analysis, it represents the probability of the data given specific parameters. This concept is crucial because it helps determine how plausible different parameter values are based on the evidence.
For example, if a marketing campaign generates specific sales figures, the likelihood function assesses how well various models predict those results. Higher likelihood values indicate that a model better fits the observed data.
Updating Beliefs
Likelihood functions play a crucial role in updating beliefs about parameters. Initially, analysts start with prior beliefs or assumptions about these parameters. After observing data, they can use the likelihood to refine their beliefs.
This process involves multiplying the prior distribution by the likelihood function. The result is called the posterior distribution. It reflects updated beliefs after considering new evidence. For instance, if initial assumptions about customer preferences change after analyzing sales data, the likelihood helps adjust those views accordingly.
Impact on Optimization
The impact of likelihood on Optimization is significant. It directly influences decision-making in marketing strategies. As marketers gather more data, they can continually update their models using likelihood functions. This leads to more accurate predictions and better resource allocation.
In Bayesian Optimization, maximizing the likelihood can guide marketers toward optimal decisions. Businesses can target their efforts effectively by focusing on areas where the possibility is highest. For example, marketers can invest more resources if a particular advertisement shows high engagement rates.
Decision-Making Process
Incorporating likelihood into analysis benefits the decision-making process. It gives a systematic approach to evaluating options based on data-driven insights. Marketers can prioritize strategies that show higher probabilities of success based on past performance.
Using likelihood also reduces uncertainty in decisions. Companies can make informed choices by relying on statistical evidence rather than guesswork. This leads to improved outcomes and higher returns on investment.
Combining Prior and Likelihood
Prior Information
Prior information is essential in Bayesian Optimization. It represents what is already known before analyzing new data. For example, a company might know that specific marketing strategies have worked well. This knowledge helps shape initial beliefs about potential outcomes.
This prior belief is combined with new evidence from marketing campaigns. The evidence comes from data such as customer responses or sales figures. Combining prior information and this new evidence leads to a more informed decision-making process.
Likelihood Function
The likelihood function measures how likely the observed data is given a specific model. For example, if a company runs an ad campaign, it can assess how possible it is to achieve particular results based on the chosen strategy. This function provides a way to evaluate different marketing approaches.
When you combine the likelihood with the prior information, you create a framework for understanding uncertainty. This method allows marketers to update their beliefs based on actual performance data.
Posterior Distributions
The result of combining prior information and likelihood is called the posterior distribution. This distribution reflects updated beliefs after considering new evidence. For example, if a campaign performs better than expected, the posterior distribution shifts to indicate increased confidence in that strategy.
Posterior distributions are significant for refining marketing strategies. They help marketers identify which tactics yield better results. By analyzing these distributions, companies can allocate resources more effectively, leading to improved targeting and higher return on investment.
Iterative Process
Bayesian Optimization is an iterative process. Marketers continuously update their priors as new data becomes available. Each round of analysis refines the posterior distribution further. This cycle allows businesses to adapt quickly to changing market conditions.
For instance, if a company’s ads initially target young adults but later show success with older demographics, it can adjust its strategies accordingly. This iterative nature ensures that marketing efforts remain relevant and effective over time.
Continuous Improvement
The combination of prior and likelihood supports continuous improvement in marketing outcomes. As campaigns progress, businesses learn from their successes and failures. They adjust their strategies based on updated insights from posterior distributions.
This approach fosters a culture of experimentation within organizations. Teams can test new ideas while relying on previous knowledge and current data. Over time, this leads to smarter marketing decisions and better overall performance.
Enhancing CTR with Bayesian Methods
Strategy Development
Bayesian Optimization helps marketers improve click-through rates (CTR) in digital advertising. This method uses prior knowledge and data to make better decisions. Marketers can identify which ad elements attract users the most. For example, they can test headlines, images, and call-to-action buttons. By analyzing past performance, they can predict which combination will yield the best results.
Marketers should focus on A/B testing various ad elements. For instance, one ad may use a bold headline while the other uses a softer approach. The results show which version leads to higher CTR. This process allows for continuous improvement of advertising strategies.
Dynamic Adjustments
Real-time data is crucial for optimizing campaigns. Marketers can track user interactions as they happen, allowing them to adjust ads quickly based on performance metrics. If an ad is underperforming, marketers can change its elements immediately. They might swap out images or modify the text to see if it boosts engagement.
Using real-time data also helps in understanding audience behavior. Marketers can identify trends and preferences among different demographics. For example, if younger users prefer video ads over static images, campaigns can be adjusted accordingly. Adapting to these insights can significantly enhance CTR.
Combination Testing
Testing combinations of ad elements is vital for finding optimal configurations. Bayesian Optimization allows marketers to explore various setups without exhaustive testing. They can analyze how different factors interact with each other. For example, combining a specific image with a particular headline may produce better results than either element alone.
Marketers should prioritize testing combinations likely to resonate with their target audience. Using insights from previous campaigns provides a solid foundation for these tests. The goal is to find the perfect mix that maximizes engagement and conversion rates.
Practical Applications in Marketing
Case Studies
Many brands have used Bayesian Optimization to improve their marketing results. One notable example is a leading e-commerce company. They utilized Bayesian methods to personalize email campaigns. By analyzing customer behavior, they optimized send times and content, leading to a 20% increase in open rates.
Another case involved a major software firm. They ran multiple A/B tests for their landing pages. Using Bayesian Optimization, they could quickly identify the most effective design. As a result, they experienced a 15% boost in conversion rates.
A/B Testing Benefits
Bayesian methods provide clear advantages for A/B testing. Traditional approaches often require large sample sizes and long testing periods. In contrast, Bayesian Optimization can effectively work with smaller samples. It updates probabilities as new data comes in.
Marketers can make quicker decisions based on real-time insights. This flexibility allows for faster adjustments during campaigns. For instance, if one ad performs better than another, marketers can shift resources immediately. This leads to better allocation of budgets and improved overall performance.
Resource Allocation
Efficient resource allocation is crucial in marketing. Bayesian Optimization helps marketers decide where to invest their budgets. It considers various factors, such as audience engagement and campaign performance.
Using these methods, companies can prioritize high-performing channels. For example, if social media ads yield better results than email marketing, funds can be reallocated accordingly. This ensures that every dollar spent maximizes return on investment.
Future Trends
The future of marketing analytics looks promising with Bayesian Optimization. As data collection improves, more businesses adopt these techniques for deeper insights, and predictive analytics will become more common.
As AI continues to evolve, its integration with Bayesian methods will enhance decision-making processes.
Moreover, personalization will reach new heights. Brands will use Bayesian models to tailor messages even further, which can increase customer loyalty and engagement.
Final Remarks
Bayesian Optimization is a game changer for your marketing strategies. By harnessing the power of prior information and likelihood, you can make smarter decisions that enhance your campaign’s effectiveness. This method allows you to predict outcomes and improve click-through rates, making your marketing efforts more efficient and impactful.
Now’s the time to dive into Bayesian Optimization. Implement these techniques in your next campaign and watch your results soar. Your marketing success is just a decision away.
Frequently Asked Questions
What is Bayesian Optimization?
Bayesian Optimization is a statistical method for optimizing complex functions. It uses probabilistic models to make informed decisions, improving efficiency in finding optimal solutions.
How does Bayesian Optimization benefit marketing?
It enhances decision-making by predicting outcomes based on prior data. This leads to better targeting, improved customer engagement, and higher conversion rates.
What role does prior information play in Bayesian Optimization?
Prior information helps establish initial beliefs about the parameters being optimized. It guides the algorithm in making more informed predictions and adjustments.
Can Bayesian methods improve click-through rates (CTR)?
Yes, Bayesian methods can significantly enhance CTR by analyzing user behavior patterns and optimizing ad placements based on predictive insights.
What are the practical applications of Bayesian Optimization in marketing?
Applications include A/B testing, personalized marketing strategies, pricing optimization, and campaign performance analysis. These lead to more effective marketing efforts and better ROI.
Is Bayesian Optimization suitable for all types of marketing campaigns?
While highly effective for many campaigns, it works best with data-rich environments where historical data can inform future strategies.
How can I implement Bayesian Optimization in my marketing strategy?
Start by identifying key metrics to optimize. Use existing data to establish priors, then apply Bayesian algorithms to test and refine your marketing approaches continuously.