Federated Machine Learning for Marketing: Insights & Hurdles
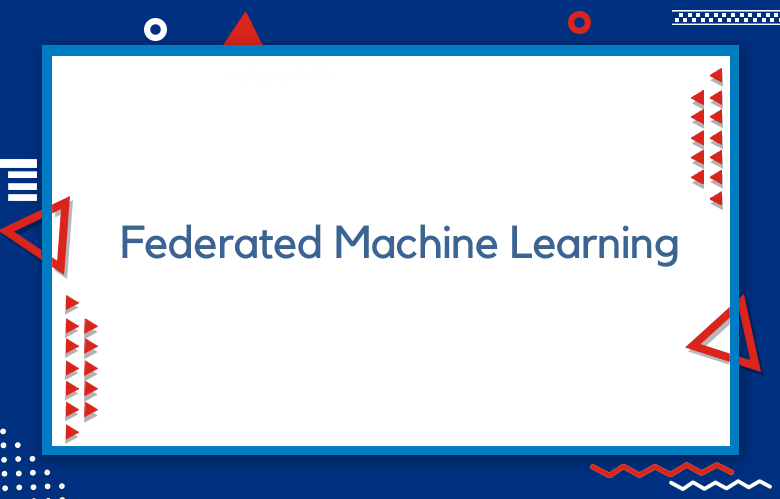
Have you ever wondered how businesses can harness data without compromising privacy? Federated machine learning for marketing is revolutionizing the way companies analyze customer behavior. This innovative approach allows brands to train algorithms on decentralized data, ensuring user information stays secure.
Marketers can now gain insights while respecting privacy laws and ethical standards. By using federated learning, businesses can improve their targeting strategies and boost engagement without the risk of data breaches. This method not only enhances personalization but also builds trust with customers.
Key Takeaways
- Federated machine learning allows marketers to use data from multiple sources without compromising privacy, making it essential for modern marketing strategies.
- Understanding your data is crucial; identify what data you have and how it can be used effectively in federated learning.
- This technology is not limited to one industry; it explores how different sectors apply federated learning to enhance their marketing efforts.
- Ensure you have enough complementary data; the success of federated learning relies on combining diverse datasets for better insights.
- When implementing federated learning in your marketing strategy, be prepared to tackle data security and model accuracy challenges.
- Stay informed about the latest developments in federated machine learning to leverage its full potential for your marketing campaigns.
Understanding Federated Machine Learning
Basics of Federated Learning
Federated learning is a method where data stays on the user’s device. It allows for decentralized data processing, which means models are trained across many devices without sharing the actual data. Traditional machine learning relies on central servers to collect and process data, which can lead to privacy risks.
Encryption plays a key role in federated learning. It protects sensitive information during the learning process. Data is never sent to a central server, reducing the risk of breaches. Instead, only model updates are shared. This keeps personal data safe while still improving models.
Benefits for Marketing
Federated learning can significantly enhance customer segmentation. Companies can analyze user behavior without compromising privacy, leading to more targeted marketing strategies. For example, businesses can tailor ads based on local trends without accessing individual data.
Federated learning also improves predictive analytics. Marketers can forecast customer behavior more accurately. They gain insights from diverse datasets while respecting user privacy, resulting in better marketing campaigns that resonate with audiences.
Another advantage of federated learning is cost-effectiveness. Traditional methods often require expensive infrastructure for data storage and processing. With federated learning, companies save on these costs and avoid the need for extensive data-sharing agreements and compliance issues.
Data Privacy Importance
Protecting customer data is crucial today. Many users are concerned about how their information is used. Federated learning effectively addresses these privacy concerns. It allows marketers to analyze data without directly accessing it.
Companies can gather insights without risking customer trust, and it builds stronger relationships between brands and consumers.
Another benefit of federated learning is regulatory compliance. Laws like GDPR require strict rules around data usage. By using federated learning, companies can stay compliant with these regulations, leverage valuable insights, and adhere to legal standards.
Know Your Data
Data Types in Marketing
Marketing relies on various data types. Transactional data comes from customer purchases. This data shows what products customers buy and how much they spend. Behavioral data tracks customer interactions online. It includes clicks, page views, and time spent on a website.
Understanding these data types is crucial for federated learning applications. Different data types provide unique insights into customer behavior. For example, transactional data informs inventory decisions, while behavioral data helps tailor marketing messages. Each type of data influences marketing strategies and outcomes differently.
Data Quality and Relevance
High-quality data significantly impacts federated learning models. Clean, accurate data leads to better model performance. The model’s predictions will be better if the data is correct or outdated. Regular evaluation of data relevance ensures it aligns with marketing goals.
Marketers should implement methods to improve data quality. They can conduct audits to identify inaccuracies and streamline this process using automated tools. These steps help ensure that only valuable information enters federated learning processes.
Leveraging Diverse Data Sources
Integrating diverse data sources enhances model training. Combining internal datasets with external ones creates a fuller picture of customer behavior. For instance, social media data can complement sales records, leading to richer insights.
Using external data has distinct advantages. It provides context that internal data may lack. By analyzing broader trends, marketers can adapt their strategies effectively. Diverse sources lead to more effective marketing strategies overall.
Federated Learning Across Industries
Cross-Industry Collaborations
Partnerships between companies in different sectors can enhance federated learning. These collaborations allow firms to share insights without exposing sensitive data. For example, a retail company might partner with a financial institution. By combining customer behavior data, they can create more personalized marketing strategies.
Innovative marketing solutions often arise from these partnerships. Companies can leverage each other’s strengths, leading to better targeting and improved customer experiences. A notable example is the collaboration between healthcare and tech companies. They used federated learning to predict patient needs while protecting personal health information.
Industry-Specific Applications
Federated learning finds unique applications in various industries. In retail, businesses analyze shopping patterns without compromising customer privacy. This helps them tailor promotions effectively. Similarly, the finance sector uses federated learning to detect fraud across multiple banks. Each bank contributes data insights while keeping customer details secure.
These industry-specific applications drive targeted marketing efforts. Retailers can offer personalized discounts based on shopping history, and financial institutions can instantly alert customers about unusual transactions. However, challenges exist. Different industries have varying regulations about data sharing. Adapting federated learning models to meet these regulations is crucial.
Success Stories and Case Studies
Several businesses have successfully implemented federated learning in their operations. For instance, a significant e-commerce platform partnered with local stores. They used federated learning to analyze buying trends across regions, resulting in a substantial sales increase due to targeted advertising.
Another case study involves a bank that collaborated with insurance companies. The companies shared insights on customer behavior while maintaining privacy standards, which led to improved product offerings tailored to customers’ needs.
Innovative approaches can help businesses improve customer engagement and increase revenue. Readers should consider similar methods for their marketing strategies.
Data Complementarity and Volume
Importance of Data Volume
Sufficient data volume is crucial for effective federated learning. With enough data, models can learn patterns accurately. Larger datasets improve model accuracy and performance significantly. For example, a study in 2020 showed that more data led to a 15% increase in predictive accuracy for marketing campaigns.
Organizations can increase their data volume through collaboration and partnerships. Partnering with other companies allows access to larger datasets without compromising privacy. Sharing data among trusted partners can help build more muscular models. This approach fosters innovation while ensuring compliance with regulations.
Complementary Data Sets
Complementary data sets play a vital role in enhancing federated learning outcomes. When combined, these datasets provide richer insights than individual datasets alone. Organizations can identify complementary datasets by analyzing their data gaps. For instance, a retail company might partner with a logistics firm to gain insights into delivery patterns.
Utilizing complementary datasets can lead to better decision-making for marketing strategy. By sharing this data while maintaining privacy, organizations can benefit without exposing sensitive information. The key is establishing clear guidelines for using and sharing data.
Enhancing Insights with More Data
Data accumulation is essential for refining marketing strategies. More data leads to deeper insights into customer behavior and preferences. A company that tracks customer interactions across multiple platforms gains a comprehensive view of its audience.
Data diversity enriches the analytical process. Different data types, such as demographic, transactional, and behavioral, provide varied perspectives. This diversity helps marketers understand trends better and tailor their campaigns effectively.
Organizations should prioritize collecting diverse data types and respect privacy laws. Companies enhance their marketing effectiveness by focusing on both the quantity and quality of data.
Overcoming Federated Learning Challenges
Technical Hurdles and Solutions
Federated learning faces several technical challenges. One major issue is data heterogeneity. Different devices may have varying data distributions, which can lead to model bias. Another challenge is communication efficiency. Transferring model updates can be slow and consume bandwidth.
Unions exist for these hurdles. Improved algorithms can help manage data diversity. Techniques like federated averaging allow models to learn from different data sources effectively. Organizations should also invest in training for their teams. Ongoing education ensures that staff understands the latest advancements in federated learning technology.
Ensuring Data Security
Data security is crucial in federated learning environments. Organizations must implement robust security measures. This protects sensitive information while models train on decentralized data.
Best practices include using strong encryption methods. Encryption secures data during transmission and storage. Secure communication protocols also play a vital role. They ensure that only authorized parties access the data.
Organizations should regularly assess their security measures. This helps identify vulnerabilities and update protocols as needed.
Legal and Ethical Considerations
The legal implications of using federated learning in marketing are significant. Companies must comply with regulations like GDPR in Europe or CCPA in California, which govern how personal data is collected and used.
Ethical considerations also arise, especially regarding transparency and consent. Users should know how their data contributes to model training, and organizations must obtain explicit consent before using any personal information.
Establishing clear policies is essential. These policies should address both legal and ethical aspects of federated learning. Companies can build trust by being transparent about their practices.
Closing Thoughts
Federated machine learning is transforming marketing strategies. By understanding your data and leveraging federated learning, you can gain insights while keeping customer information private. This approach allows you to harness the power of data from various sources without compromising security.
Embrace this innovative method to stay ahead in the competitive landscape. Tackle the challenges head-on and explore how federated learning can enhance your marketing efforts. Don’t wait—start integrating federated machine learning into your strategy today for better results and a stronger connection with your audience.
Frequently Asked Questions
What is federated machine learning?
Federated machine learning is a decentralized approach that allows multiple devices to train a model collaboratively without sharing raw data. This enhances privacy and security while enabling organizations to utilize valuable insights from distributed data sources.
How does federated learning benefit marketers?
Marketers can leverage federated learning to gain insights from customer data across various platforms without compromising privacy. This enables personalized campaigns while adhering to data protection regulations, enhancing customer trust and engagement.
What types of data can be used in federated learning?
Federated learning can utilize diverse data types, including customer interactions, purchase histories, and behavioral patterns. The key is that the data remains on local devices, ensuring compliance with privacy standards.
What industries benefit most from federated learning?
Industries like finance, healthcare, and retail significantly benefit from federated learning. They can analyze sensitive data while maintaining compliance with regulations and improving decision-making and customer experiences.
What are the main challenges of implementing federated learning?
Challenges include managing data heterogeneity, ensuring model accuracy, and addressing communication efficiency. Organizations must invest in robust infrastructure and strategies to overcome these hurdles for successful implementation.
How does data complementarity enhance federated learning?
Data complementarity refers to the unique insights gained from combining different datasets. In federated learning, this concept allows organizations to create more accurate models by leveraging diverse information while preserving data privacy.
Is federated learning compliant with data protection laws?
Yes, federated learning aligns with data protection laws such as GDPR. Keeping data localized and only sharing model updates minimizes risks associated with personal data exposure, fostering trust among users.