How to Manage Marketing ML Workflows
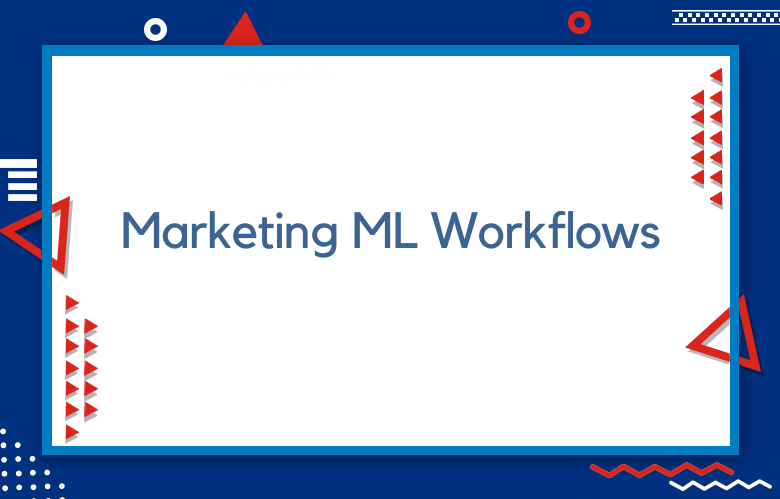
Machine Learning (ML) transforms marketing operations and presents new possibilities for businesses to understand their customers better and make data-driven decisions.
However, marketing ML workflows can be complex, time-consuming, and resource-intensive.
Managing the various stages and stakeholders involved in ML model development and deployment requires an intelligent strategy and efficient tools. This blog post will explore essential tips and tools for managing marketing ML workflows.
What is Marketing ML Workflows?
Marketing ML workflows are a series of steps used to train machine learning algorithms to process data related to marketing campaigns.
They are automated workflows that allow data to be transferred between different software applications and algorithms and for information to be analyzed in real-time.
ML workflows are used in marketing to analyze consumer behavior, predict future trends, and optimize marketing campaigns.
How do Marketing ML Workflows Work?
Marketing ML workflows analyze data on a company’s marketing initiatives from different sources.
These sources include customer relationship management (CRM) software, email marketing tools, and social media platforms.
The algorithms used in ML workflows are trained to process data related to customer behavior, such as purchase history and online interactions.
These algorithms then use this data to predict what a customer or group of customers is likely to do in the future, which can inform marketing campaigns and increase the chances of success.
Simplifying Your Marketing Workflow with Machine Learning.
In this technological era, machine learning (ML) has proven to be a revolutionary tool for simplifying workloads and increasing productivity.
As businesses grow, the need for optimization and scale grows, making machine learning a much-needed solution.
For marketing, machine learning maximizes the chances of reaching the right audience, streamlines workflows and decision-making processes, and provides the insights needed to fuel growth. This blog post will delve into how you.
Can manage marketing workflows using ML to achieve business objectives.
Streamlining Your Marketing ML Workflows for Better Results.
Are you looking to put your marketing machine learning (ML) workflows in order? With the ever-rising complexity of today’s marketing campaigns, streamlining your ML workflows can be daunting.
However, managing your marketing ML workflows can be a challenge! In this blog post, we will share some insights into managing your marketing ML workflows and making them work better, faster, and more brilliantly for your business.
Understanding Marketing ML Workflows: Automating your Marketing Strategy.
As businesses strive towards digital transformation, machine learning (ML) presents a revolutionary opportunity in automating, scaling, and optimizing marketing operations.
In this digitally competitive era, marketing ML workflows have become vital to any company’s growth strategy. Today, we will discuss marketing ML workflows, how they work, and how businesses can leverage them to enhance their marketing campaigns.
Marketing ML workflows are automated sequences that use machine learning algorithms to tailor marketing campaigns, personalize customer experiences, and optimize marketing performance.
These workflows enable marketers to make data-driven marketing decisions and improve customer experience.
Best Practices for Implementing Marketing ML Workflows.
To successfully implement marketing ML workflows, businesses should clearly understand their goals and how ML workflows can help achieve them.
They should also be aware of the importance of data quality and invest in solutions that ensure data accuracy and cleanliness.
Marketing teams should involve data scientists and IT professionals in the implementation process to ensure that algorithms are correctly set up and operating effectively.
Additionally, it is essential to continue to monitor and refine ML workflows as they are used to ensure that they continue to provide value and meet the needs of the business.
Define Your Goals and Metrics:
Before starting any ML project, you must clarify your objectives and performance indicators. What do you want to achieve with the ML model? What metrics will you use to measure success?
Defining clear goals and KPIs will help you avoid common pitfalls, such as building models that don’t address business needs or chasing irrelevant metrics. It’s also crucial to involve all relevant stakeholders in defining the goals and metrics, including the marketing team, IT department, and data scientists.
Keep Your Data Clean and Secure:
The quality and security of your data are fundamental for building accurate and trustworthy ML models. If required, you must ensure that your data is adequately relevant, complete, and anonymized.
You must also protect your data from unauthorized access and leverage encryption and other security measures. An efficient way to manage your data is to use a dedicated data platform to store, visualize, and manipulate your data in a secure and scalable environment.
Automate Your Model Training and Deployment:
Training an ML model can be time-consuming and error-prone, especially if done manually. Automation can significantly speed up model training and deployment and reduce human errors.
You can use various tools and platforms to automate the entire ML workflow, from data preprocessing to model hyperparameter tuning and deployment. Some famous examples are Google Cloud ML Engine, Amazon SageMaker, and Azure Machine Learning.
Monitor Your Model’s Performance and Explainability:
Once your ML models are deployed, you must monitor their performance and explainability. Monitoring will enable you to detect and fix bugs or anomalies in real-time and optimize your model’s accuracy.
On the other hand, explainability will help you understand how your model makes predictions and enable you to explain its decisions to your stakeholders. You can use various tools and techniques for monitoring and explainability, such as TensorBoard, Kibana, and SHAP.
Stay Updated and Collaborate:
ML technology rapidly evolves, and new tools and frameworks are emerging daily. To stay on top of the latest trends and best practices, you need to keep learning and collaborating with other experts in the field.
You can attend conferences, meetups, and webinars, join online communities or forums, and read blogs and papers on ML and marketing topics. Collaborating with other teams and companies can help you tackle common challenges and share valuable resources and insights.
Conclusion:
ML can bring significant benefits to your marketing operations, but it requires a thoughtful and systematic approach to managing the various stages and stakeholders involved.
Following the tips and using the tools discussed in this blog post can ensure a successful and efficient ML workflow that delivers actionable insights and enhances customer experiences.
Remember that ML is not a magic bullet; to succeed, you must align your ML strategies with your business goals and values.
Call: +91 9848321284
Email: [email protected]