Model Clustering for Digital Marketing: Advanced Strategies
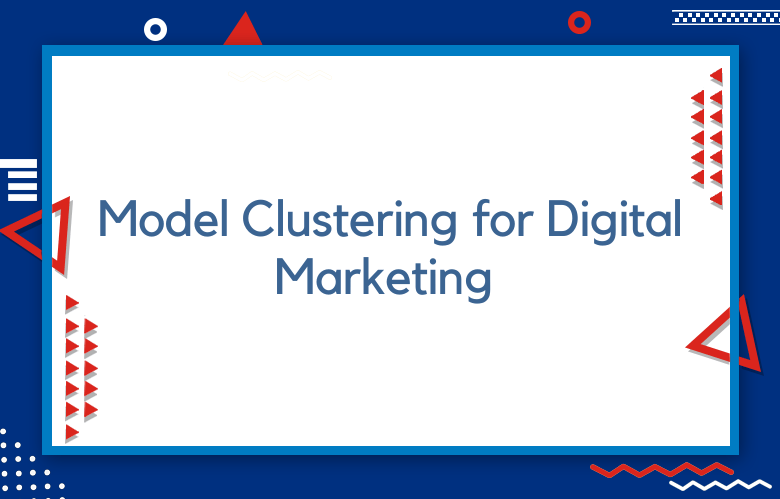
Model clustering in digital marketing leverages advanced machine-learning techniques to segment data into distinct groups based on shared characteristics without relying on predefined categories. This unsupervised learning approach is invaluable for uncovering natural patterns and segments within customer data, enabling marketers to tailor strategies and allocate resources more efficiently.
Model Clustering for Digital Marketing: Advanced Strategies
In digital marketing, clustering can revolutionize how organizations understand their audience. For example, it can identify distinct customer personas based on purchasing behavior, engagement levels, and preferences, revealing insights that might not be apparent through traditional segmentation methods. This allows for highly personalized marketing campaigns, targeted product recommendations, and optimized customer journeys, enhancing the overall customer experience and driving conversions.
Advanced clustering techniques, such as K-means, hierarchical clustering, and DBSCAN, offer different approaches to grouping data, each with advantages and suited for specific data types and marketing objectives. For instance, K-means is famous for its simplicity and efficiency in segmenting large datasets, while hierarchical clustering provides a detailed dendrogram, offering insights into the relationships between clusters.
Implementing clustering in digital marketing strategies involves careful consideration of data selection, feature engineering, and the choice of clustering algorithm. It also requires an iterative approach to refine clusters and interpret them in an actionable way for marketing strategies.
By effectively utilizing model clustering, digital marketers can better understand their audience, uncover new market opportunities, and deliver more engaging content, ultimately leading to increased customer loyalty and higher ROI. This data-driven approach aligns with the dynamic nature of digital marketing, where understanding subtle shifts in customer behavior can provide a competitive edge.
- Key Takeaways
- Understanding Model Clustering
- Cluster Analysis Basics
- Advanced Customer Segmentation
- Clustering Algorithms Overview
- Practical Clustering Applications
- Advantages of Cluster Analysis
- Marketing Analytics with Clusters
- Enhancing ROI through Clustering
- Case Studies in Digital Marketing
- Summary
- Frequently Asked Questions
- What is Model Clustering in Digital Marketing?
- How can Cluster Analysis Benefit Digital Marketing Strategies?
- Which Clustering Algorithms Are Commonly Used in Digital Marketing?
- Why are Case Studies Important in Understanding Model Clustering for Digital Marketing?
- How Does Model Clustering Enhance Return on Investment (ROI) in Digital Marketing?
Are you struggling to optimize your digital marketing strategies, including search engines, content audit, metrics, and keyword research, for better results? Model clustering for digital marketing could be the game-changer you’ve been searching for. By leveraging this advanced technique, businesses can unlock valuable insights, enhance customer targeting, and boost overall campaign performance. Stay tuned to discover how this innovative approach can advance your digital marketing initiatives.
Key Takeaways
- Implement model clustering to segment customers and target them with tailored marketing strategies effectively.
- Utilize cluster analysis to group customers based on similarities in behavior, preferences, or demographics for personalized marketing campaigns.
- Explore various clustering algorithms such as K-means or hierarchical clustering to identify distinct customer segments efficiently.
- Leverage cluster analysis in marketing analytics to gain insights into customer behavior, improve targeting, and enhance campaign performance.
- Enhance return on investment (ROI) in digital marketing by using clustering to optimize resource allocation and increase campaign effectiveness.
- Refer to case studies in digital marketing to see real-world examples of how model clustering has been successfully applied to improve marketing outcomes.
Understanding Model Clustering
Definition
Model clustering in digital marketing involves grouping customers based on similarities in behavior, preferences, or demographics. This technique helps marketers tailor strategies to specific customer segments effectively.
Importance
Model clustering is crucial for personalized marketing, allowing businesses to target customers with relevant content and offers. By understanding distinct customer groups, companies can create more engaging campaigns.
Enhanced Customer Segmentation Accuracy
Implementing model clustering enhances customer segmentation accuracy by identifying patterns that may not be apparent through traditional methods. It enables marketers to categorize customers into precise clusters for targeted marketing efforts.
Cluster Analysis Basics
Mathematical Model
Cluster analysis is a mathematical model employed in digital marketing to group customers with similar characteristics. This model allows businesses to target their audience based on shared traits and behaviors.
K-Means Role
K-means cluster analysis plays a crucial role in customer segmentation by dividing the customer base into distinct clusters. This method helps marketers tailor their strategies to meet each segment’s specific needs and preferences.
Dynamic Definitions
In cluster analysis, it’s essential to recognize the dynamic nature of cluster definitions and market strategies. As customer behavior evolves, these definitions must adapt accordingly to ensure accurate targeting and personalized marketing approaches.
Advanced Customer Segmentation
Benefits
Cluster analysis offers more precise segmentation compared to traditional threshold-based methods. Companies can tailor their marketing strategies more effectively by identifying distinct customer groups based on various dimensions.
Real-time Personalization
Daily cluster-based segmentation allows for dynamic adjustments in marketing campaigns based on real-time consumer behavior. This enables businesses to deliver personalized content and offers promptly, increasing customer engagement.
Automated Systems
Using automated systems in customer segmentation enhances the accuracy and efficiency of the process. Companies can make informed decisions regarding product development and marketing strategies by analyzing vast amounts of data quickly.
Clustering Algorithms Overview
K-Means Clustering
K-means clustering is a popular algorithm in digital marketing for segmenting customers based on similarities in their behavior or characteristics. It divides data points into clusters by minimizing the sum of squared distances within each cluster. This efficient algorithm works well with large datasets, making it ideal for grouping customers with similar purchase histories.
Hierarchical Clustering
Hierarchical clustering organizes data points into a tree-like structure, forming clusters based on the distance between data points. Unlike K-means, this method does not require the number of clusters to be defined beforehand. In digital marketing, hierarchical clustering is beneficial for identifying clusters at different levels of similarity among customers.
DBSCAN Clustering
DBSCAN (Density-Based Spatial Clustering of Applications with Noise) effectively identifies clusters of varying shapes and sizes. It works by grouping together closely packed data points while marking outliers as noise. In digital marketing, DBSCAN helps detect irregular patterns in customer behavior that may not fit traditional cluster shapes.
Gaussian Mixture Model (GMM)
The Gaussian Mixture Model assumes that the data points are generated from a mixture of several Gaussian distributions. This model assigns probabilities to each point belonging to a particular cluster rather than definitively assigning them. GMM is suitable for identifying complex patterns in customer behavior that simpler algorithms cannot capture.
Role of Clustering Algorithms in Predictive Analytics
Clustering algorithms are crucial in predictive analytics to understand customer behavior and preferences. Marketers can tailor their strategies to target each group effectively by segmenting customers into distinct groups based on similarities. For instance, if a company wants to launch a new product, it can use clustering algorithms to identify which customer segments are most likely to be interested.
Suitability for Different Data Types
When choosing a clustering algorithm for digital marketing, it’s essential to consider the nature of the analyzed data. For categorical data, algorithms like K-modes or hierarchical clustering may be more suitable due to their ability to handle non-numeric attributes. On the other hand, for continuous numerical data, algorithms like K-means or GMM are preferred for their effectiveness in finding patterns within numeric features.
Practical Clustering Applications
Consumer Clusters
Consumer clusters are pivotal in digital marketing, allowing targeted and personalized campaigns. Businesses can tailor their marketing strategies effectively by segmenting customers based on similarities.
Utilizing machine learning algorithms, companies can analyze vast amounts of data to identify distinct consumer groups through cluster marketing. For instance, a retail company can use clustering to group customers based on purchasing behavior, demographics, or preferences.
Targeted Marketing
Marketers, including cluster marketing, can gain valuable insights into consumer behavior and preferences through visualization techniques. By understanding these patterns, businesses can create tailored marketing campaigns that resonate with specific consumer groups.
One practical application is clustering to identify high-value customers more likely to make repeat purchases. By targeting these groups with personalized offers or promotions, businesses can enhance customer loyalty and increase sales.
Customer Engagement
Utilizing cluster analysis in digital marketing significantly impacts customer engagement. Businesses can improve customer satisfaction and loyalty by delivering relevant content and offers to specific consumer segments.
For example, an e-commerce platform can use clustering to categorize customers based on their browsing history and purchase patterns. This allows them to send targeted product recommendations or promotions, leading to higher conversion rates.
Advantages of Cluster Analysis
Practicality
Cluster analysis offers practical benefits by grouping similar data points and simplifying complex datasets for marketers. This method allows for efficient segmentation based on shared characteristics, streamlining marketing strategies.
Homogeneity
By emphasizing homogeneity, cluster analysis ensures that each group contains individuals with similar traits. This enhances targeting precision, enabling marketers to effectively tailor campaigns to specific customer segments.
Dynamic Clustering
Dynamic clustering in cluster analysis adapts to changes in consumer behavior, ensuring marketing efforts remain relevant over time. This flexibility allows for real-time adjustments, optimizing campaign performance continuously.
Superiority Over Rule-Based Segmentation
Compared to rule-based segmentation approaches, cluster analysis offers a more flexible and data-driven solution. Instead of rigid rules, cluster analysis leverages statistical algorithms to accurately identify natural groupings within data.
Pros of Cluster Analysis
- Enables precise targeting.
- Adapts to evolving consumer trends.
- Simplifies complex datasets for actionable insights.
Cons of Rule-Based Segmentation
- Limited adaptability to changing market dynamics.
- May overlook subtle variations within customer segments.
Personalized Marketing Efforts
Cluster analysis empowers marketers to create personalized marketing campaigns by understanding different customer groups’ unique preferences and behaviors. By segmenting customers based on similarities, marketers can deliver tailored messages that resonate with each segment’s needs.
Marketing Analytics with Clusters
Enhanced Insights
Clusters derived from cluster analysis play a crucial role in enhancing marketing analytics. By segmenting customers based on similarities in behavior or preferences, companies can gain deeper insights into their target audience through cluster marketing. This segmentation allows businesses to tailor their marketing strategies more effectively, leading to higher engagement and conversion rates.
Improved Customer Modeling Accuracy
The utilization of cluster analysis significantly improves customer modeling accuracy in marketing analytics. Businesses can create more precise customer profiles by grouping customers with similar characteristics into clusters. This accurate customer modeling lets companies personalize their marketing efforts, resulting in better customer relationships and increased loyalty.
Targeted Marketing Campaigns
Marketing analytics with clusters pave the way for more targeted marketing campaigns. By analyzing data through the lens of topic and content clusters, companies can identify specific trends and patterns within their customer base. This detailed understanding allows businesses to create highly targeted campaigns that resonate with different customer segments, ultimately driving higher ROI and brand engagement.
Enhancing ROI through Clustering
Cost-Effective Strategy
Content cluster strategy is pivotal in enhancing marketing ROI by optimizing content organization. Marketers can effectively target specific keywords and improve search engine rankings by strategically grouping related content into content clusters.
Efficient Data Organization
Implementing keyword research within the context of clustering allows for better data organization. Marketers can identify relevant keywords and create targeted content, leading to higher engagement and conversion rates.
Budget Optimization
Clustering enhances ROI by ensuring that marketing efforts focus on high-performing content clusters. By analyzing data trends and performance metrics, marketers can allocate their marketing budget more efficiently toward strategies that yield the best results.
Streamlined Campaigns
Through effective clustering, marketers can streamline their campaigns by focusing on specific topics or themes within content clusters. This approach improves content relevance and enhances user experience, leading to higher click-through rates and improved conversion rates.
Targeted Audience Engagement
Clustering enables marketers to tailor their messaging to specific audience segments based on interactions with different content clusters. By understanding audience preferences and behaviors, marketers can create personalized campaigns that resonate with their target audience, resulting in higher engagement and brand loyalty.
Case Studies in Digital Marketing
Successful Implementations
Cluster analysis has revolutionized digital marketing strategies for various businesses. For instance, a leading e-commerce company utilized clustering to segment its customer base based on purchasing behavior. By identifying distinct clusters, they tailored marketing efforts and personalized promotions, significantly increasing sales.
Another example involves a global fashion brand that applied cluster analysis to analyze customer preferences across different regions. By understanding regional variations in tastes and trends, they optimized their content marketing campaigns, leading to higher engagement rates and improved brand loyalty.
Outcomes of Cluster Analysis
The outcomes of implementing cluster analysis in digital marketing have been remarkable. Businesses have reported enhanced targeting precision, increased conversion rates, and improved customer satisfaction. By leveraging cluster insights, companies can deliver more relevant content and offer to specific audience segments, maximizing their marketing strategy effectiveness.
Moreover, cluster analysis has enabled marketers to uncover hidden patterns within data sets, allowing them to refine their marketing tactics for better results. Businesses can tailor their messaging and promotions to resonate with each group by analyzing clusters of customers with similar characteristics or behaviors.
Lessons Learned
From these real-world case studies, several key lessons emerge. Firstly, marketers must prioritize data-driven decision-making by leveraging cluster analysis tools to gain valuable consumer behavior and preferences insights. Understanding customer segments empowers businesses to create targeted campaigns that yield higher returns on investment.
Secondly, the successful implementation of cluster analysis requires continuous monitoring and adjustment. Companies can stay agile in responding to changing market dynamics and consumer trends by regularly evaluating cluster performance and adapting marketing strategies.
Summary
You’ve delved into the world of model clustering for digital marketing, understanding its basics, algorithms, and practical applications. By segmenting customers effectively, you can enhance your marketing strategies, leading to improved ROI. Leveraging clustering in your marketing analytics can provide valuable insights and help tailor your campaigns to specific audience segments. Real-world case studies showcase the power of clustering in optimizing digital marketing efforts. Embrace model clustering to revolutionize your approach and stay ahead in the competitive digital landscape.
Take action now. Implement model clustering in your digital marketing endeavors and witness firsthand the transformative impact it can have on your campaigns. Stay informed, adapt continuously, and watch your marketing efforts yield unprecedented results.
Frequently Asked Questions
What is Model Clustering in Digital Marketing?
Model clustering in digital marketing refers to grouping similar data points or customers based on specific characteristics for targeted marketing strategies.
How can Cluster Analysis Benefit Digital Marketing Strategies?
Cluster analysis helps identify distinct customer segments, allowing marketers to tailor personalized campaigns, improve customer engagement, and optimize marketing efforts for higher ROI.
Which Clustering Algorithms Are Commonly Used in Digital Marketing?
Standard clustering algorithms used in digital marketing include K-means, Hierarchical clustering, DBSCAN, and Gaussian Mixture Models (GMM), each with its unique approach to grouping data points.
Why are Case Studies Important in Understanding Model Clustering for Digital Marketing?
Case studies provide real-world examples of how businesses have successfully implemented model clustering techniques to enhance their marketing strategies, showcasing practical applications and results for better comprehension.