Models Transfer Learning for Digital Marketing
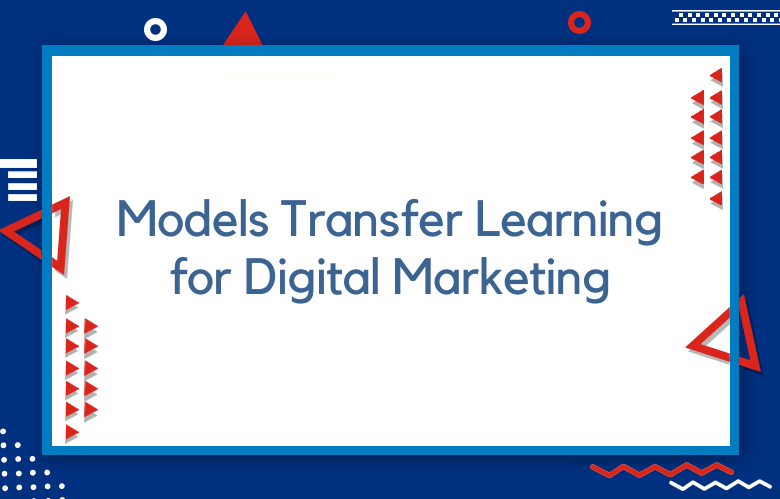
Transfer learning is a powerful technique in machine learning that involves taking a pre-trained model developed for one task and repurposing it for a similar, yet distinct, problem. In digital marketing, transfer learning offers a highly efficient way to leverage existing knowledge and models, accelerating the development of predictive models and AI-driven strategies without building models from scratch or requiring extensive datasets.
Models Transfer Learning for Digital Marketing
For digital marketers, transfer learning can be a game-changer. It enables the application of sophisticated AI models, previously trained on vast datasets, to specific marketing tasks such as customer segmentation, sentiment analysis, content recommendation, and more. This approach significantly reduces the time and resources needed for model training, making advanced AI capabilities more accessible.
Transfer learning is particularly beneficial when data may be scarce or building a highly accurate model from the ground up would be resource-intensive. By adapting models that have already learned relevant features from large, diverse datasets, marketers can achieve high levels of accuracy and performance in their predictive analytics and personalization efforts.
Incorporating transfer learning into digital marketing strategies allows for more agile adaptation to market trends, more profound insights into customer behavior, and the creation of more engaging and personalized customer experiences. As AI evolves, transfer learning in digital marketing is poised to become a standard practice, offering a competitive edge to those who adopt it effectively.
- Key Takeaways
- Understanding Transfer Learning
- Transfer Learning vs. Traditional SEO
- Optimizing Content with Transfer Learning
- Enhancing SEO with Deep Learning
- Analyzing User Behavior
- Addressing Technical SEO Issues
- Optimizing Multimedia for SEO
- Best Practices in SEO Transfer Learning
- Challenges and Solutions in SEO Transfer
- Closing Thoughts
- Frequently Asked Questions
- What is Transfer Learning in Digital Marketing?
- How Does Transfer Learning Benefit SEO Strategies?
- Why Should Digital Marketers Consider Deep Learning for SEO?
- What are the Best Practices for Implementing Transfer Learning in SEO?
- How Can Digital Marketers Overcome Challenges in Implementing Transfer Learning for SEO?
Are you ready to revolutionize your digital marketing strategy with cutting-edge models, transfer learning techniques, and powerful tools, questioning the industry’s ability? Dive into the world of advanced marketing strategies as we explore how models, transfer learning, industry examples, and tutorials can elevate your digital presence. Discover the powerful tool of leveraging data-driven insights, optimizing campaigns, and enhancing customer engagement like never before. Stay ahead of the curve in the dynamic landscape of digital marketing by harnessing the potential of these innovative approaches. Get ready to unlock new possibilities and drive unprecedented growth for your brand in the digital sphere.
Key Takeaways
Implement transfer learning in digital marketing.
Use transfer learning techniques to enhance your digital marketing strategies effectively.
Optimize content with transfer learning.
Leverage transfer learning to improve the quality and relevance of your content for better SEO performance.
Incorporate deep learning for SEO enhancement.
Explore the benefits of deep learning in boosting SEO efforts and gaining insights into user behavior.
Analyze user behavior for targeted marketing.
Utilize data analysis from user behavior to tailor your marketing strategies and enhance user engagement.
Address technical SEO issues promptly.
Identify and resolve technical SEO issues to ensure optimal website performance and visibility.
Apply best practices in SEO transfer learning.
Follow industry best practices to maximize the impact of transfer learning on your SEO initiatives.
Understanding Transfer Learning
Benefits
Transfer learning in machine learning allows for efficiently adapting pre-trained models to new tasks. It saves time and computational resources by leveraging knowledge from existing models through transfer learning.
Implementation
For digital marketing, transfer learning is crucial in optimizing website content and analyzing user behavior by transferring information from one model to another.
Transfer Learning Vs. Traditional SEO
Key Differences
Transfer learning in digital marketing involves leveraging pre-trained models to improve performance, while traditional SEO relies on manual optimization techniques. Transfer learning adapts existing knowledge to new tasks; traditional SEO requires starting from scratch.
Advantages of Transfer Learning
Efficiency
Transfer learning reduces the time and resources needed for training models compared to traditional SEO methods.
Accuracy
Transfer learning can achieve higher accuracy levels in predicting search engine behavior by utilizing pre-trained models.
Flexibility
Transfer learning allows for quick adaptation to changing algorithms and trends in the digital marketing landscape.
Enhancing SEO Algorithms with Transfer Learning
Implementing transfer learning in SEO algorithms enhances their capabilities by incorporating knowledge from related tasks. This integration enables algorithms to adapt to new data patterns quickly without extensive retraining.
Optimizing Content with Transfer Learning
Leveraging Transfer Learning
Transfer learning in digital marketing involves utilizing pre-trained models to enhance various aspects of content optimization. By leveraging transfer learning from one industry to another, marketers can significantly improve the efficiency of their strategies.
Enhancing Search Engine Optimization
One significant application of transfer learning is optimizing website content for search engines. Through this technique, marketers can boost their website’s visibility and ranking on search engine result pages.
Improving Search Result Accuracy
Thanks to transfer learning, pre-trained language models play a crucial role in enhancing the accuracy of search results. By incorporating these models into the optimization process, marketers can ensure that users receive more relevant and precise information.
Fine-Tuning for User Behavior Analysis
Fine-tuning models allow marketers to analyze and understand user behavior accurately. This process enables them to tailor their content based on user preferences, leading to higher engagement and conversion rates.
Enhancing SEO with Deep Learning
Analyzing Content
Deep learning techniques revolutionize content analysis for SEO, enabling precise identification of relevant keywords and topics. By leveraging neural networks, marketers can extract valuable insights from vast data.
Deep learning models like BERT allow for a more nuanced understanding of search intent and user behavior. This leads to creating highly targeted and engaging content that resonates with the audience.
Improving Search Rankings
Deep learning enhances search engine ranking factors, such as relevance, authority, and user experience. Algorithms like RankBrain use machine learning to interpret complex queries and deliver accurate search results.
By incorporating deep learning algorithms into SEO strategies, businesses can effectively adapt to evolving search algorithms. This ensures their content remains visible and competitive in the ever-changing digital landscape.
Optimizing Website Performance
Deep learning models offer advanced capabilities in website content optimization by analyzing user engagement metrics and feedback. This data-driven approach enables marketers to tailor their content for improved visibility and conversion rates.
Implementing deep learning techniques for on-page SEO optimization enhances website performance across various devices and platforms. Marketers can effectively deliver personalized experiences that cater to individual user preferences.
Analyzing User Behavior
Understanding Intent
User behavior analysis is crucial in digital marketing. Transfer learning can revolutionize SEO by understanding search intent, user interactions, and models. By leveraging transfer learning models, marketers can gain insights into user behavior patterns for optimal SEO strategies.
Identifying Patterns
Transfer learning’s ability to adapt pre-trained models to new scenarios is invaluable. SEO means recognizing and classifying user behavior based on specific characteristics using a model. These models excel at identifying patterns that lead to accurate results in predicting user actions, preferences, and transfer learning.
Enhancing Optimization
The main benefit of using transfer learning to analyze user behavior lies in its efficiency in making sense of complex data. By fine-tuning preexisting models, marketers can uncover valuable insights that drive SEO optimization strategies. This process not only saves time but also ensures more effective decision-making.
Addressing Technical SEO Issues
Common Problems
Broken links and slow loading times are common technical SEO issues that significantly impact a website’s performance. These issues can lead to poor user experience and lower search engine rankings.
Transfer learning offers a solution by leveraging pre-trained models to detect and resolve these problems efficiently. Transfer learning streamlines the process of identifying and fixing technical SEO issues by utilizing existing knowledge from one task to improve another.
Detecting Broken Links
Transfer learning can help detect broken links by training models on a large dataset of websites with known link structures. This enables the model to identify anomalies in link patterns, such as missing or non-functional links, leading to quicker detection and resolution of broken links.
Resolving Slow Loading Times
In the case of slow loading times, transfer learning is crucial in optimizing website performance. By training models on datasets containing information about page load speeds and factors affecting them, transfer learning helps identify areas for improvement and implement strategies to enhance loading times.
Improving SEO Performance
Transfer learning enhances the technical aspects of SEO performance by providing insights into optimizing website structure, content, and user experience through models. By fine-tuning models based on specific SEO metrics and criteria, transfer learning enables marketers to make data-driven decisions for improving overall search visibility.
Pros
- Efficient detection and resolution of technical SEO issues.
- Optimization of website performance for better user experience.
Cons
- Requires expertise in machine learning for effective implementation.
- Initial setup and training may involve time and resource investment.
- Transfer learning enhances the efficiency of detecting broken links.
- It aids in resolving slow loading times by optimizing website performance.
Optimizing Multimedia for SEO
Image Recognition
Transfer learning, involving a model, offers a powerful approach to optimizing multimedia content for marketing purposes. By leveraging pre-trained image recognition models and transfer learning, marketers can enhance their SEO strategies. Fine-tuning and transfer learning of these models allows for improved image indexing and better visibility in search results.
UtiliTransferning in image recognition involves retraining existing models on various images related to the specific marketing context. This process enables the model to learn domain-specific features that are crucial for SEO success through transfer learning. As a result, marketers can boost their website’s ranking by ensuring search engines accurately understand images.
Video Recognition
Transfer learning plays a significant role in enhancing marketing efforts for video content. Marketers can fine-tune video recognition models using transfer learning to improve the discoverability of their multimedia content online. Businesses can increase their online presence by training these models with relevant data, such as product videos or promotional clips.
The benefits of using transfer learning for video recognition extend beyond SEO optimization. By delivering tailored video content, marketers can create more engaging and personalized experiences for their target audience. This improves user engagement, drives conversions, and boosts overall marketing performance.
Best Practices in SEO Transfer Learning
Selecting Models
When implementing transfer learning in SEO, selecting suitable pre-trained models is crucial. Look for models trained on vast datasets and transfer learning to capture diverse patterns. Consider factors like model size, training time, compatibility with your data, and transfer learning.
Fine-Tuning Process
To fine-tune pre-trained models for SEO using transfer learning, start by freezing the initial layers to retain general features. Then, the later layers of the model are adapted to learn domain-specific patterns using transfer learning. Experiment with different learning rates and batch sizes for optimal performance.
Monitoring Performance
Monitoring model performance is essential in transfer learning for SEO. Regularly evaluate accuracy, precision, and recall metrics to ensure the model aligns with SEO objectives. Implement tools like TensorBoard to visualize performance metrics over time.
Updating Models
Regularly updating the transfer learning model is critical for sustained SEO success. Retraining the model with fresh data ensures relevance as algorithms evolve and search engine updates occur. Set up a schedule to retrain the model periodically to maintain competitiveness.
Challenges and Solutions in SEO Transfer
Data Scarcity
Due to data scarcity and models, many companies face challenges in SEO transfer learning. Limited data availability can hinder the effectiveness of models, leading to subpar performance. Companies can leverage techniques like data augmentation, transfer learning, and synthetic data generation to address this issue and enhance the training dataset.
Lack of Customization
A significant challenge in SEO transfer learning is the lack of customization. Generic models may not cater to the specific nuances of each business, impacting the accuracy of predictions. One solution is to fine-tune pre-trained models using transfer learning on domain-specific data to tailor them to the unique requirements of a company’s SEO strategy.
Overfitting
Overfitting poses a common obstacle in implementing a transfer learning model for SEO. When a model performs well on training data but fails to generalize to new instances, it indicates overfitting. To mitigate this issue, regularization techniques such as dropout layers and early stopping can help prevent overfitting and improve model generalization.
Closing Thoughts
Incorporating transfer learning into your digital marketing strategy can revolutionize your SEO efforts. By leveraging pre-existing models and data, including transfer learning, you can enhance content optimization, delve deeper into user behavior analysis, and tackle technical SEO challenges more effectively. Embracing transfer learning and advanced model techniques will undoubtedly set you apart in the competitive digital landscape.
As you navigate the realm of SEO transfer learning, remember to stay updated on best practices, remain adaptable to evolving algorithms, and continuously refine your strategies. The road ahead may present challenges, but with perseverance and a commitment to innovation, you can position yourself for sustained success in the dynamic world of digital marketing.
Frequently Asked Questions
What is Transfer Learning in Digital Marketing?
Transfer learning is a technique where knowledge gained from solving one problem is applied to a different but related problem using a model. Digital marketing involves leveraging pre-trained models using transfer learning to enhance tasks like content optimization and user behavior analysis.
How Does Transfer Learning Benefit SEO Strategies?
Transfer learning can significantly improve SEO strategies by providing insights into user behavior, optimizing multimedia content, addressing technical issues efficiently, enhancing overall content quality for better search engine visibility, and using a model.
Why should Digital Marketers Consider Deep Learning for SEO?
Deep learning algorithms can analyze complex patterns in user data, leading to more accurate user intent and behavior predictions. This enables marketers to create highly targeted and engaging content that resonates with their audience, boosting SEO performance.
What are the Best Practices for Implementing Transfer Learning in SEO?
To successfully implement transfer learning in SEO, digital marketers should focus on understanding the fundamentals of transfer learning, selecting relevant pre-trained models, optimizing content based on insights from these models, and continuously monitoring and refining their strategies for optimal results.
How can Digital Marketers Overcome Challenges in Implementing Transfer Learning for SEO?
Digital marketers can overcome challenges in implementing transfer learning by staying updated on the latest trends and technologies in the field, collaborating with experts or agencies specializing in AI-driven marketing solutions, conducting thorough testing and experimentation, and adapting their strategies based on performance metrics.