Deep Q-Networks(DQN) for Marketing: Deep Reinforcement Learning Algorithm
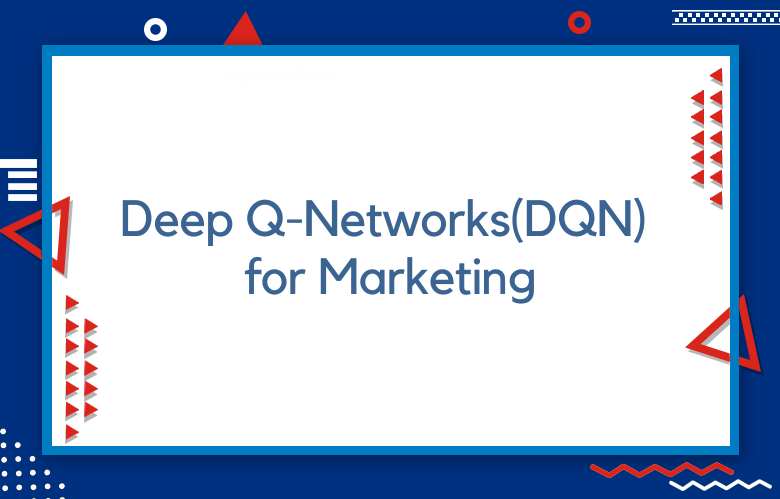
Technology is transforming every industry, and marketing is no exception. With the advent of AI and machine learning, marketers are adopting new digital marketing strategies. Deep Q-Networks(DQN) is a recent deep reinforcement learning algorithm that is making waves.
This AI algorithm has been used in the gaming industry for a while, but its marketing applications are only beginning to be realized. In this blog post, we discuss how Deep Networks (DQN) can be used in marketing to improve customer engagement, sales, and brand awareness.
Artificial intelligence has revolutionized the marketing world, and many companies have adopted innovative marketing techniques to increase their sales. One such method is using Deep Networks (DQN) for marketing campaigns.
DQN is a deep reinforcement learning algorithm that uses neural networks to analyze various inputs and outputs to determine which actions are best suited for achieving specific goals. This blog post will explore the basics of DQN and how it can be leveraged for marketing purposes.
What is Deep Q-Networks(DQN)?
Deep Q-Networks (DQN) is a reinforcement learning algorithm that enables an artificial intelligence agent to learn from its environment and make decisions accordingly.
The algorithm utilizes a combination of deep neural networks and Q-learning to produce an optimal policy for the agent. DQN is considered one of the most impactful breakthroughs in deep reinforcement learning.
What distinguishes DQN from traditional Q-learning is its ability to handle high-dimensional input such as images, sound, and text. This feature enables the algorithm to solve complex tasks that were previously considered impossible.
A key feature of DQN is the use of experience replay, which stores previous experiences of the algorithm and allows for more efficient learning by reducing the impact of correlation in consecutive observations.
What is a Deep Reinforcement Learning Algorithm?
A deep reinforcement learning algorithm is a machine learning technique that combines profound learning principles and reinforcement learning. The primary purpose of this algorithm is to enable an artificial agent to make decisions or take actions in an environment to maximize its rewards.
The artificial agent learns through trial and error in a deep reinforcement learning algorithm. It is trained to interact with an environment, perceive its various states, and receive feedback through rewards or punishments depending on its actions.
This feedback helps the agent to determine which actions are the most beneficial for accomplishing a specific task.
To accomplish this, deep reinforcement learning algorithms use artificial neural networks with multiple layers to identify the most optimal actions.
These networks are designed to learn the complex relationships between the input stimuli from the environment and the agent’s decisions. The neural network gradually learns to make more accurate predictions and actions as more data is processed.
Understanding Reinforcement Learning and Deep Q-Learning for Marketing
Reinforcement learning and deep Q-learning are innovative developments within the field of artificial intelligence that have the potential to drive significant advancements in marketing.
Reinforcement learning is a subset of machine learning that operates on the principle of maximizing rewards through trial and error. This would involve identifying the most effective strategies for optimizing customer engagement and sales outcomes in marketing.
On the other hand, deep Q-learning uses neural networks to approximate the optimal decision-making strategy for a given problem through an iterative feedback and refinement process.
Applying reinforcement learning and deep Q-learning to marketing offers a range of benefits for businesses seeking to improve their bottom line.
By leveraging these advanced techniques, companies can better understand customer behaviors and preferences and develop strategies tailored to their target audience’s specific needs and interests.
Moreover, these technologies can also be used to optimize pricing, promotions, and inventory management, thereby boosting operational efficiency and profitability.
The Concept of Reinforcement Learning and its Impact on Marketing
Reinforcement learning is a type of machine learning based on trial and error. It is a process where an artificial intelligence (AI) system is trained to learn from experience by receiving feedback as punishments or rewards.
This feedback influences the system’s subsequent behavior and improves its decision-making process. In other words, the system learns from its own experiences and corrects its actions based on the feedback it receives.
The impact of reinforcement learning on marketing has been immense. Online businesses, for instance, can use reinforcement learning to optimize their pricing strategies. Pricing is one of the most critical aspects of e-commerce, as it directly affects a customer’s buying decisions.
Reinforcement learning algorithms can help a business determine the optimal price point by analyzing large amounts of data, such as customer behavior, market trends, and historical sales data.
Exploring Q-Learning Algorithm and its Efficiency for Marketing
Marketing is an ever-evolving field that requires constant innovation to stay ahead of competitors.
With the rapid advancement of technology and the rise of digital marketing, it has become imperative for marketers to find new and effective ways to reach their target audience. One such method that has gained popularity in recent years is the Q-learning algorithm.
Q-learning is a machine-learning technique used to make decisions in an uncertain environment. It is a form of reinforcement learning where an agent learns to achieve a goal through trial and error.
Q-learning involves incorporating reward signals into an iterative process that allows it to learn from its past actions to make better decisions in the future.
Overcoming Limitations with Deep Q-Learning Algorithm for Marketing
The marketing industry constantly evolves and demands constant innovation and adaptation to new techniques. One of the challenges marketers face is the limitation that traditional marketing methods pose. However, this challenge is being addressed with the emergence of deep learning algorithms.
Deep Q-learning, a reinforcement algorithm, is making waves in the marketing industry as it can make decisions and recommendations with higher accuracy than traditional methods.
This algorithm has become a sought-after tool as it can analyze vast amounts of data and consider multiple variables to provide more precise recommendations.
One of the significant benefits of Deep Q-learning is that it can adapt and evolve through experience. This means that the more data it analyzes, the better its performance.
As a result, there is an increased likelihood of identifying potential consumers, customers, or clients and providing more personalized recommendations.
Implementing DQN for Marketing: Benefits and Considerations
Improved Decision Making
Implementing a deep Q-learning network (DQN) for marketing can help to improve decision-making. DQN is a type of artificial intelligence that uses reinforcement learning to make decisions based on past experiences.
By using DQN, marketers can learn from the data they collect and use it to make better decisions about their campaigns. This can lead to more effective campaigns that are better targeted and have higher ROI.
Increased Efficiency
Using DQN for marketing can also increase efficiency. By automating specific tasks, such as analyzing customer data or optimizing campaigns, marketers can save time and focus on other aspects of their job. This can help them be more productive and get done in less time.
Better Customer Experiences
Using DQN for marketing can also lead to better customer experiences. By understanding customer behavior and preferences, marketers can create more personalized campaigns tailored to each customer’s needs. This leads to a better overall experience for the customer, which can result in increased loyalty and higher conversion rates.
More Accurate Predictions
Using DQN for marketing also allows marketers to make more accurate predictions about future trends and behaviors. By analyzing past data, marketers can gain insights into how customers will respond to specific campaigns or offers in the future, allowing them to plan accordingly and maximize their results.
Cost Savings
Using DQN for marketing can also lead to cost savings by reducing the need for manual labor or outsourcing specific tasks such as analytics or optimization processes.
This helps businesses save money by eliminating unnecessary costs associated with manual labor or outsourcing services while accessing top-notch AI technology that provides valuable insights into customer’s behavior and preferences.
Data Security
When implementing a DQN for marketing, it is vital to consider data security risks and the potential benefits of using AI technology for marketing purposes.
Businesses must take steps such as encrypting customer data or using secure cloud storage solutions when storing customer information so that it remains safe from malicious actors who may try to access it without permission or misuse it in some way.
Enhancing Marketing Strategies with Deep Q-Networks (DQN)
Improving marketing strategies is critical for businesses in today’s highly competitive marketplace. As such, researchers and marketers are exploring various approaches to optimize marketing techniques, from social media campaigns to email marketing tactics.
One such strategy that has gained considerable momentum is using Deep Networks (DQN).
DQNs employ artificial intelligence (AI) to improve marketing strategies by evaluating the effectiveness of various marketing techniques. This neural network model utilizes reinforcement learning to enhance decision-making capabilities, thereby increasing the efficiency of marketing efforts.
Conclusion:
Deep Q-Networks(DQN) represents a game-changing tool for marketers. By combining deep learning and reinforcement learning, marketers can improve customer engagement, optimize sales, and enhance brand awareness.
As this technology evolves, the possibilities for DQN are limitless, and marketers should actively explore how this cutting-edge technology can benefit their businesses today. With DQN, the prospects for marketing are endless.
Deep Q-Networks is an innovative marketing technique that can help companies optimize their marketing activities and maximize investment returns.
By analyzing large amounts of data, DQNs can create personalized campaigns tailored to individual customers’ preferences, identify the best channels and timing to reach customers, and provide recommendations for future marketing actions.
As companies adopt new marketing techniques to stay competitive, keeping an eye on the latest trends and tools that can drive growth is essential. If you want to enhance your marketing efforts and gain a competitive advantage in your industry, adopting DQN technology may be worth considering.
Call: +91 9848321284
Email: [email protected]