Marketing Using Overfitting in Machine Learning
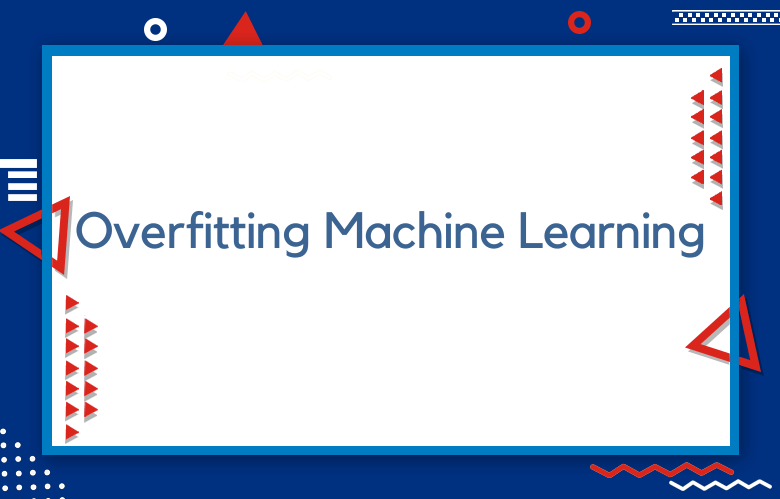
Traditional marketing strategies no longer work effectively in today’s world. Digital marketing has taken the forefront with technological advancements, with new techniques emerging daily.
With Machine Learning (ML), you can help identify crucial trends in your data that can change the course of your marketing campaigns. One such method that has taken the marketing world by storm is overfitting.
We will explore how overfitting in machine learning can help you create personalized marketing campaigns and increase the efficiency of your marketing strategy.
What is Overfitting?
Overfitting is a common phenomenon in machine learning where an algorithm learns too much from the data it is trained on.
It becomes so good at predicting outcomes based on the training data that it performs poorly on new data.
The algorithm becomes too specialized for the training data and cannot generalize the learning to new data sets. Overfitting can be problematic, leading to inaccurate predictions and erroneous decision-making.
How can Overfitting be Used in Marketing?
Despite the potential drawbacks, overfitting is a valuable tool in marketing.
By fitting a statistical model very close to the data, machine learning algorithms can identify patterns and relationships between variables that might not be apparent in a broader analysis.
These patterns can create highly targeted marketing campaigns that are more likely to resonate with specific audiences.
Understanding Overfitting in Machine Learning.
Machine learning trains the model on your dataset to predict the output. Overfitting occurs when the model becomes too complex, which causes the accuracy of the training data to increase but the accuracy of the test data to decrease.
In simpler terms, the model becomes too specific to the training data and fails to predict accurately when applied to real-life scenarios. Overfitting can adversely impact machine learning models, and the same effect is observed in marketing.
Overfitting in Lead Generation.
Incorporating overfitting into lead generation, you can create highly targeted campaigns customized according to the users’ interests in real-time. You can use past user data to create the ideal customer profile, which can be used to nurture new leads.
By analyzing the user’s online behavior in real time, you can deliver personalized messages that speak directly to the user’s needs and preferences, ultimately leading to more conversions.
Overfitting in Customer Retention.
Using overfitting in customer retention, you can create personalized experiences that satisfy your customers’ needs.
With the ability to predict what customers might need next, you can keep customers engaged with personalized experiences that meet their evolving preferences.
Understanding the lifetime value of a customer and refining marketing campaigns through overfitting ensures their continued business.
Overfitting in Improving ROI.
Overfitting in marketing campaigns helps save time and resources. By channeling your marketing resources into creating personalized experiences that closely align with the user’s preferences, you can ensure a higher return on your investment in engagement, conversions, and customer satisfaction.
Using Overfitting in Machine Learning for Marketing Success.
Machine learning has revolutionized various industries, and marketing is one of them. With the help of machine learning, marketers can now predict consumer behavior, analyze their preferences and offer personalized marketing.
But machine learning is not a magic wand that will solve all your marketing problems. Like every strategy, it comes with its own set of challenges. One of them is overfitting in machine learning.
While overfitting is usually a problem in machine learning, it can help marketers gain valuable insights and boost their marketing efforts.
We will explore how marketers can use overfitting in machine learning and leverage it to their advantage.
Marketing Strategy: Using Overfitting in Machine Learning for Better Results.
Regarding marketing strategies, businesses must be savvy in using data to inform their decisions. Machine learning has become an effective tool for analyzing and predicting consumer behavior, and overfitting can take this one step further.
But what is overfitting, and how can it be used to improve your marketing strategy? We will explore the basics of overfitting and its potential benefits for businesses.
Overfitting occurs when a machine learning model is trained too precisely on the given data. This can be problematic because the model needs to generalize to new data points, leading to inaccurate predictions.
However, in marketing, overfitting can be helpful. For example, if a business analyzes customer data from one region, it may tailor its marketing efforts to that region.
Overfitting in Machine Learning and Its Benefits in Marketing.
Overfitting in machine learning is often seen as a negative aspect of data analysis. Still, it can also be viewed as an opportunity for marketers to get more out of their data.
Identifying new target markets:
Overfitting can be a powerful tool for marketers identifying new target markets. By fitting a model too closely to their existing customer data, marketers may uncover new segments they had yet to consider.
These new segments could have different needs, preferences, and purchasing habits than the marketer’s existing customer base, allowing for more effective and targeted marketing efforts.
Uncovering optimal messaging:
Overfitting can also be used to uncover optimal messaging for specific target markets. By fitting a model too closely to the data, marketers can identify which messages are most effective for particular customer segments.
This information can then be used to craft marketing campaigns that are laser-focused on the needs and preferences of the target audience.
Improving the accuracy of marketing strategies:
Overfitting can lead to higher overall accuracy for a marketing strategy. By fitting a model too closely to existing customer data, marketers can ensure that their marketing efforts are focused squarely on the target audience.
This can lead to higher conversion rates, increased customer retention, and, ultimately, increased revenue.
Measuring the effectiveness of marketing campaigns:
Overfitting can also be used to measure the effectiveness of marketing campaigns more accurately.
By fitting a model too closely to existing customer data, marketers can accurately predict the outcomes of their marketing campaigns.
This can allow for more accurate measurement of key performance indicators (KPIs), including conversion rates, clickthrough rates, and customer retention.
Avoiding overfitting pitfalls:
While overfitting can be a powerful tool for marketers, it’s essential to avoid the pitfalls of overfitting.
Specifically, marketers must be careful not to rely too heavily on a model that has been overfitted to a specific dataset. Instead, they should seek to validate the results of their models on new, unseen data to ensure that their models are robust and effective.
Conclusion:
In conclusion, overfitting in machine learning has the potential to boost your marketing campaigns by creating highly personalized experiences that increase engagement and conversions while reducing the need for additional resources.
Using overfitting for lead generation, customer retention, and improving ROI is just the beginning.
As you continue to learn about the technology and analyze your data, you will gain even better insights and learn new ways to leverage overfitting for your benefit. By embracing this technology, you can set your marketing apart and take your campaigns to the next level.
Call: +91 9848321284
Email: [email protected]