Ensemble Learning for Digital Marketing Pro Strategies
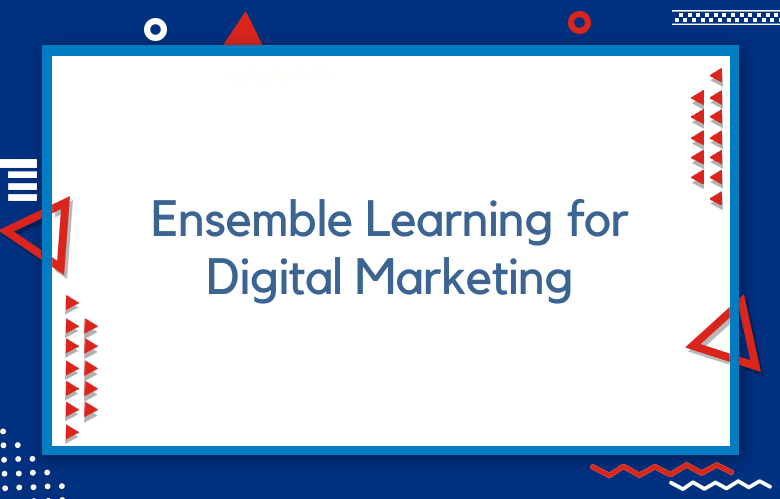
Ensemble learning, a powerful machine learning technique that combines multiple models to improve prediction accuracy, is making significant inroads into digital marketing strategies. By leveraging various algorithms’ strengths and mitigating their weaknesses, ensemble learning offers a robust approach to tackling complex marketing challenges, from customer segmentation and churn prediction to content personalization and ad targeting.
Ensemble Learning for Digital Marketing: Pro Strategies
Ensemble learning provides a flexible and adaptive framework in the dynamic and data-rich digital marketing environment, where consumer behaviors and market trends are constantly evolving. It integrates diverse models like decision trees, neural networks, and regression models, each contributing different perspectives and insights, resulting in more accurate and reliable predictions.
Adopting ensemble learning in digital marketing strategies allows for a more nuanced understanding of customer data, leading to enhanced customer experiences, optimized marketing campaigns, and improved ROI. Techniques such as bagging, boosting, and stacking are commonly used in ensemble models, each with its unique way of combining predictions from multiple models to achieve better performance than any single model could on its own.
For digital marketers looking to harness the power of ensemble learning, key considerations include selecting the right combination of base models, balancing model complexity with interpretability, and continuously evaluating and refining ensemble models in response to changing data and market conditions. By integrating ensemble learning into their analytical toolkit, digital marketers can unlock deeper insights, drive innovation, and maintain a competitive edge in the fast-paced digital landscape.
- Key Takeaways
- Basics of Ensemble Learning
- Ensemble Learning in Digital Marketing
- Enhancing Ad Targeting Accuracy
- Optimizing Website Content
- Customer Segmentation Strategies
- Sales Forecasting Techniques
- Benefits and Challenges of Ensemble Learning
- Implementing Ensemble Learning Strategies
- Summary
- Frequently Asked Questions
- What is ensemble learning, and how does it apply to digital marketing?
- How does ensemble learning benefit digital marketers?
- What are the challenges associated with implementing ensemble learning in digital marketing?
- Can ensemble learning help improve customer segmentation strategies?
- How does ensemble learning contribute to optimizing website content for digital marketing purposes?
Did you know ensemble learning in digital marketing can boost predictive accuracy by up to 3% compared to individual models? Harnessing the power of multiple algorithms working together, ensemble learning is revolutionizing how marketers optimize campaigns and drive results using marketing automation, analytics, and digital marketing strategies. Discover how combining diverse models can enhance decision-making processes, increase ROI, and outperform traditional single-model approaches. Stay ahead of the curve in the competitive digital landscape with the analytics, social media, marketing campaigns, and recommendations shared here.
Key Takeaways
- Utilize ensemble learning techniques in digital marketing to improve decision-making processes.
- Enhance ad targeting accuracy by combining multiple models for more precise audience segmentation.
- Optimize website content by leveraging ensemble learning to analyze user behavior and preferences effectively.
- Implement customer segmentation strategies using ensemble methods to tailor marketing campaigns for specific audience segments.
- Improve sales forecasting accuracy by employing ensemble learning techniques that combine various forecasting models.
- Be aware of the benefits, such as increased prediction accuracy, and challenges, like model complexity, associated with implementing ensemble learning in digital marketing.
Basics of Ensemble Learning
Definition
Ensemble learning in machine learning involves combining multiple models to improve predictive performance. It leverages the collective intelligence of diverse models.
Bagging
Bagging, or Bootstrap Aggregating, is a technique where multiple instances of the same model are trained on different subsets of the training data. These models then vote to make predictions.
Boosting
Boosting is another ensemble method where models are trained sequentially, with each new model correcting errors made by its predecessors. This iterative process focuses on examples that previous machine learning algorithms struggled with.
Ensemble learning offers several advantages.
Improved accuracy
By combining multiple models, ensemble learning can often outperform individual models.
Robustness
Ensemble methods are less susceptible to overfitting compared to single models.
Versatility
Different ensemble techniques cater to various types of data and problem domains.
Ensemble Learning in Digital Marketing
Application: Food Delivery Business
Ensemble learning in digital marketing is crucial in optimizing strategies for the food delivery business. By combining various machine learning models, digital marketers can improve the effectiveness of email marketing campaigns and social media advertising. This approach enables enterprises to reach their target audience more efficiently.
Utilizing ensemble techniques, such as bagging and boosting, along with machine learning algorithms and models, allows digital marketers to analyze vast amounts of data from different sources. For instance, they can leverage ensemble learning and machine data to help make decisions and enhance social media advertising campaigns by creating personalized content based on customer preferences. This method significantly increases engagement rates and boosts overall campaign performance.
Customer Data Analysis and Behavior Prediction
Implementing ensemble learning techniques empowers digital marketers to gain deeper insights into customer behavior. By utilizing diverse machine learning algorithms, businesses can accurately analyze big data sets to understand customer preferences and trends and make decisions. This detailed analysis enables precise customer segmentation, leading to more targeted marketing efforts.
Digital marketers can more accurately predict customer behavior through ensemble learning and machine data. Combining multiple machine learning models, they can forecast customer actions based on past interactions and purchasing patterns. This predictive capability, driven by machine learning algorithms and data, allows businesses to tailor their marketing strategies to individual customer needs, enhancing the overall customer experience.
Benefits: Personalized Recommendations
One significant benefit of using ensemble learning in digital marketing is the ability to provide personalized recommendations. By leveraging ensemble techniques and machine learning algorithms, businesses can offer tailored product suggestions based on individual customer preferences, data, and browsing history. This level of personalization enhances the overall shopping experience for customers.
Moreover, ensemble learning enables digital marketers to optimize their marketing spending by more effectively targeting specific customer segments. By analyzing data through ensemble methods and machine learning algorithms, businesses can allocate resources efficiently toward campaigns that are most likely to generate high returns. This targeted approach improves ROI and enhances customer satisfaction through relevant and engaging content.
Enhancing Ad Targeting Accuracy
Combining Algorithms
Ensemble learning in digital marketing leverages different algorithms, machines, and data to enhance ad targeting accuracy. Blending machine learning algorithm models, such as decision trees, neural networks, and logistic regression, creates a more robust prediction mechanism. This approach allows for a more comprehensive analysis of user behavior and preferences.
Optimizing Ad Campaigns
Through ensemble learning using machine data, marketers can significantly improve the effectiveness of their ad campaigns. By leveraging diverse algorithms, machine learning, and techniques, ad targeting accuracy is enhanced, leading to better engagement with the target audience. This optimization results in higher conversion rates and improved advertising services’ return on investment (ROI).
Reducing Ad Spend Wastage
One key advantage of ensemble learning in digital marketing is its ability to reduce ad spend wastage. By utilizing multiple algorithms simultaneously, precise targeting becomes achievable, minimizing the chances of displaying ads to irrelevant audiences. This approach ensures that advertising budgets are allocated efficiently to individuals more likely to convert using machine learning algorithms.
Optimizing Website Content
User Behavior Analysis
Ensemble learning leverages user behavior data to optimize website content effectively. Analyzing user data from various sources using machine learning algorithms provides valuable insights for content optimization. This approach saves time by offering recommendations based on search history, preferences, and machine learning algorithms.
Improved User Experience
Ensemble learning plays a crucial role in enhancing user experience on websites. By understanding user preferences and behaviors and using machine learning algorithms, it tailors content to meet their needs. This personalized approach ensures users find the most relevant and engaging content, increasing satisfaction.
A/B Testing Optimization
Ensemble learning is a powerful tool for conducting A/B testing to optimize website content. It helps compare different versions of content by analyzing quality, engagement metrics, and conversion rates. Marketers can make data-driven decisions to improve content performance by utilizing ensemble learning algorithms.
Customer Segmentation Strategies
Enhanced Identification
Ensemble learning, a machine learning technique, is crucial in enhancing customer segmentation strategies by leveraging its ability to analyze vast amounts of data. By utilizing various algorithms and machine learning simultaneously, it can more accurately identify distinct customer segments.
By employing ensemble learning, marketers can pinpoint specific customer behavior patterns, allowing for the creation of highly targeted marketing campaigns. This approach enables businesses to tailor their strategies using machine learning based on precise insights derived from complex data sets.
Personalized Marketing Campaigns
Ensemble learning for customer segmentation leads to the development of personalized marketing campaigns. This method allows marketers to create customized content and offerings that resonate with individual customer segments.
- Improved targeting for marketing efforts
- Increased efficiency in reaching potential customers
- Enhanced customer experience through tailored interactions
Benefits of Ensemble Learning
Ensemble learning in customer segmentation strategies offers several critical benefits for digital marketers. One significant advantage is optimizing marketing campaigns based on accurate segmentations.
- Enhanced accuracy in identifying customer segments
- Improved ROI on marketing campaigns
- Streamlined marketing attribution and tracking
Sales Forecasting Techniques
Predictive Analytics
Ensemble learning leverages predictive analytics to analyze sales data and make accurate forecasts. By combining multiple models, it improves the accuracy of predictions.
Ensemble learning techniques such as random forests and gradient boosting use historical data to identify patterns in sales trends. These machine learning models consider various factors like purchase history and conversion rates.
Enhanced Sales Forecasts
By integrating ensemble learning, digital marketers can generate more precise sales forecasts. This approach utilizes a combination of diverse machine-learning models to predict future sales based on past behavior.
Ensemble learning helps identify subtle nuances in data that individual models might miss. This leads to more reliable predictions and assists marketers in making informed decisions.
Strategic Marketing Adjustments
One key advantage of ensemble learning in sales forecasting is its ability to adapt marketing strategies based on accurate predictions. Marketers can adjust their campaigns according to the forecasts generated by ensemble models using machine learning.
Ensemble learning’s flexibility allows for real-time adjustments, ensuring that marketing efforts are aligned with predicted retention rates and customer behaviors.
Benefits and Challenges of Ensemble Learning
Improved Predictive Accuracy
Ensemble learning in digital marketing offers enhanced predictive accuracy by combining multiple models to generate more reliable predictions. By aggregating the outputs of diverse algorithms, it minimizes errors and provides more precise forecasts.
Implementing ensemble techniques such as bagging or boosting allows marketers to capitalize on the strengths of various models, leading to more accurate predictions than individual models alone. This results in better decision-making processes and improved campaign performance.
Model Robustness
One key benefit of ensemble learning for digital marketing is the enhanced model robustness it provides. By leveraging a combination of machine learning models, marketers can create a more resilient system less susceptible to outliers and noise in the data.
Ensemble methods like random forests or gradient boosting help mitigate the impact of outliers and errors, resulting in more stable predictions even when dealing with complex datasets. This robustness ensures that marketing strategies are based on solid insights and reduces the risk of making decisions based on unreliable data.
Challenges in Implementation
Despite its advantages, implementing ensemble learning in digital marketing has several challenges. One common obstacle is the increased complexity involved in managing multiple models simultaneously. Marketers must deeply understand each algorithm’s intricacies to utilize ensemble techniques effectively.
Another challenge is the computational resources required for training and running ensemble models. The process can be resource-intensive, especially when dealing with large datasets or sophisticated algorithms. Marketers must ensure they have access to sufficient computing power to leverage ensemble learning effectively.
Risks of Overfitting
A critical consideration when using ensemble learning techniques in digital marketing is the risk of overfitting. Overfitting occurs when a model performs exceptionally well on training data but fails to accurately generalize to new, unseen data. This can lead to misleading predictions and inaccurate insights.
To mitigate the risk of overfitting in machine learning, marketers must carefully tune their ensemble models, select appropriate algorithms, and utilize techniques like cross-validation to evaluate model performance accurately. Balancing model complexity with generalizability is crucial to avoid falling into the trap of overfitting.
Implementing Ensemble Learning Strategies
Integration Steps
To implement ensemble learning in digital marketing, select diverse machine learning algorithms. Combine supervised learning with unsupervised learning techniques to enhance model accuracy. Utilize a mix of decision trees, neighbor algorithms, logistic regression, and machine learning for varied perspectives.
Next, blend these models into an ensemble using techniques like bagging or boosting. Ensure the models complement each other’s weaknesses, leading to more robust predictions. Combining different machine learning algorithms can capture broader patterns and improve decision-making processes.
Monitoring and Optimization
Continuous monitoring is crucial in ensuring the effectiveness of ensemble learning in digital marketing campaigns. Regularly assess model performance, identify discrepancies, and adjust parameters accordingly—real-time feedback loops help refine predictions and optimize strategies promptly.
Moreover, A/B testing will compare the ensemble model’s performance against individual models. This allows for measuring the incremental value added by ensemble techniques. Marketers can stay ahead of trends and competitors by continuously fine-tuning the algorithms based on real-world data.
Summary
You’ve now grasped the power of ensemble learning in revolutionizing digital marketing. By leveraging this technique, you can enhance ad targeting accuracy, optimize website content, fine-tune customer segmentation strategies, and refine sales forecasting techniques. Understanding the benefits and challenges of ensemble learning equips you to implement these strategies effectively. Take charge of your digital marketing efforts by embracing ensemble learning to stay ahead of the competition and drive better results for your business.
Frequently Asked Questions
What is ensemble learning, and how does it apply to digital marketing?
Ensemble learning combines multiple machine learning models to improve predictive performance. In digital marketing, machine learning enhances accuracy in ad targeting, customer segmentation, and sales forecasting by leveraging diverse algorithms for more robust results.
How does ensemble learning benefit digital marketers?
Ensemble learning boosts accuracy in predicting customer behavior, optimizing ad campaigns, and enhancing website content. It helps marketers make data-driven decisions, leading to improved ROI, better customer engagement, and increased conversion rates.
What are the challenges associated with implementing ensemble learning in digital marketing?
Challenges include the need for quality data sources, computational resources for training multiple machine learning models, interpreting complex ensemble outputs, and ensuring seamless integration with existing marketing strategies. Overcoming these hurdles requires expertise in machine learning and a strategic approach to implementation.
Can ensemble learning help improve customer segmentation strategies?
Yes, ensemble learning can enhance customer segmentation by combining various models to accurately identify distinct customer groups. This leads to more personalized marketing campaigns, better targeting of specific demographics or behaviors, and increased relevance in messaging for improved engagement.
How does ensemble learning contribute to optimizing website content for digital marketing purposes?
Ensemble learning analyzes user behavior patterns to recommend personalized content based on preferences and interactions. By leveraging this insight, marketers can tailor website content effectively, improve user experience, increase conversions, and drive higher engagement.