Model Classification for Digital Marketing: Exploring Types & Applications
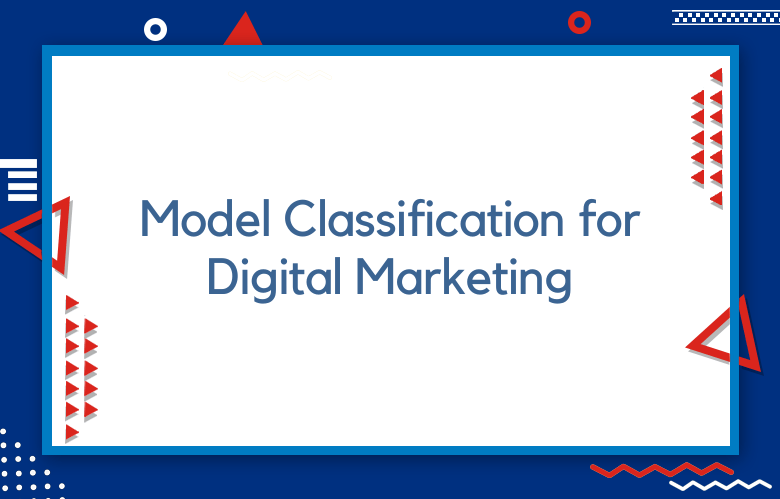
Model classification in digital marketing involves utilizing machine learning models to categorize data into distinct classes, enabling marketers to derive actionable insights and make informed decisions. This technique is pivotal in understanding consumer behaviors, segmenting audiences, personalizing content, and optimizing campaigns across digital channels.
Model Classification for Digital Marketing: Exploring Types & Applications
Classification models can range from simple logistic regression, which might be used for binary decisions like predicting customer churn (yes or no), to more complex algorithms such as decision trees, random forests, and neural networks, which can handle multi-class problems like segmenting customers into various personas based on their behaviors and preferences.
The application of classification models in digital marketing is vast and varied. They can be used for sentiment analysis, categorizing customer feedback into positive, negative, or neutral; for lead scoring, identifying which prospects are most likely to convert; and for recommendation systems, classifying products or content that would be most relevant to a user.
Incorporating classification models into digital marketing strategies allows marketers to automate decision-making processes, deliver more personalized customer experiences, and efficiently allocate resources to areas with the highest return on investment. However, the success of these models depends on the quality of the data, the appropriateness of the chosen model for the task, and ongoing evaluation and refinement to adapt to changing market dynamics and consumer behaviors.
- Key Takeaways
- Importance in Digital Marketing
- Types of Classification Models
- Strengths and Weaknesses
- Decision Trees in Marketing
- Logistic Regression Applications
- Support Vector Machines Explained
- Case Studies in Digital Marketing
- Improving Campaign Optimization
- Future Directions in Model Classification
- Summary
- Frequently Asked Questions
- What is the significance of model classification in digital marketing?
- How do decision trees impact marketing strategies?
- Can you explain the role of logistic regression in digital marketing?
- Why are support vector machines valuable tools in digital marketing?
- How can businesses leverage model classification for campaign optimization?
In the realm of digital marketing, understanding model classification is paramount. This method categorizes data to predict outcomes and enhance decision-making processes. This approach allows businesses to tailor their strategies effectively, optimizing campaigns for better results. In today’s fast-paced digital landscape, mastering model classification empowers marketers to target the right audience precisely and efficiently. This blog post delves into the significance of model classification in digital marketing and explores how it revolutionizes campaign performance. Stay tuned to unravel the intricacies of this powerful tool and elevate your marketing game.
Key Takeaways
- Understanding different classification models is crucial for effective digital marketing strategies.
- Decision trees offer a visual and intuitive approach to decision-making in marketing campaigns.
- Logistic regression is valuable in predicting customer behavior and optimizing marketing efforts.
- Support Vector Machines (SVM) provide potent tools for classifying complex data sets in digital marketing.
- Real-world case studies demonstrate model classification’s practical applications and benefits in digital marketing.
- To enhance campaign performance, focus on continuous optimization and leveraging insights from classification models.
Importance in Digital Marketing
Enhanced Strategies
Model classification is crucial in enhancing digital marketing strategies by enabling businesses to categorize and understand their target audience better. Companies can identify consumer behavior patterns by utilizing various models, such as clustering or decision trees.
This insightful data allows marketers to tailor their campaigns more effectively, ensuring that the right message reaches the right audience at the right time. With accurate model classification, businesses can optimize their marketing efforts and allocate resources efficiently.
Personalized Marketing
Accurate classification leads to personalized marketing approaches, where companies can create customized experiences for individual customers. Businesses can deliver targeted content that resonates with each customer segment by segmenting consumers based on their preferences, purchase history, and demographics.
This level of personalization enhances customer engagement and increases conversion rates and customer loyalty. Companies can build long-lasting relationships with their customers through model classification by providing relevant and valuable content.
Customer Segmentation
Model classification significantly impacts customer segmentation and targeting within digital marketing. Businesses can tailor their messaging to each segment’s specific needs and interests by categorizing customers into distinct groups based on common characteristics or behaviors.
This targeted approach ensures that marketing efforts are focused on reaching the most receptive audience, leading to higher engagement rates and improved ROI. Model classification enables companies to refine their targeting strategies continuously based on real-time data analysis.
Types of Classification Models
Consumer Behavior Paradigms
Consumer behavior paradigms play a crucial role in modeling consumer behavior for digital marketing. These paradigms encompass various psychological, sociological, and economic factors influencing how consumers interact with online products and services. By analyzing these paradigms, marketers can tailor their strategies to effectively target specific consumer segments.
Market Situation-Based Vs. Generational Characteristics Models
Market situation-based models focus on understanding consumer behavior in response to specific market conditions or events. They analyze how external factors such as promotions, pricing, or competition impact consumer decision-making. On the other hand, generational characteristics models categorize consumers based on age groups and generational traits. These models help marketers create targeted campaigns that resonate with different age demographics.
Significance of Modern Consumer Behavior Models
In today’s digital marketing landscape, modern consumer behavior models are instrumental in helping businesses stay competitive and relevant. These models leverage advanced data analytics techniques to uncover valuable insights into consumer preferences, motivations, and purchasing patterns. By utilizing sophisticated algorithms and machine learning technologies, marketers can predict future trends, personalize marketing campaigns, and enhance overall customer experiences.
Strengths and Weaknesses
Strengths
Machine learning models, such as decision trees and logistic regression, offer high accuracy in predicting customer behavior, aiding digital marketers in targeted campaigns. These models can analyze vast amounts of data quickly.
Implementing classification models enables marketers to segment their audience effectively, tailoring marketing strategies for different customer groups. This personalized approach often leads to increased engagement and conversion rates.
The flexibility of classification models allows marketers to adapt quickly to changing market trends and consumer preferences. By leveraging these models, businesses can make data-driven decisions, enhancing the overall effectiveness of their marketing efforts.
Weaknesses
One common challenge in using classification models is the interpretability of results. Complex algorithms like neural networks may provide accurate predictions but lack transparency in explaining how decisions are made.
Another limitation is the need for quality data input. Classification models heavily rely on the quality and relevance of the data used for training, making it crucial for marketers to ensure data cleanliness and accuracy.
Moreover, overfitting poses a significant risk when implementing classification models. If a model is overly complex or trained on limited data, it may not generalize to new scenarios, leading to poor performance in real-world applications.
Leveraging Strengths to Mitigate Weaknesses
To address the interpretability issue, marketers can combine different types of classification models with simpler algorithms like decision trees. This hybrid approach provides both accuracy and transparency in results.
Ensuring data quality involves thorough preprocessing steps such as cleaning and feature engineering. By investing time and resources into data preparation, marketers can improve their classification models’ overall performance and reliability.
Techniques like cross-validation and regularization can be applied to combat overfitting during model training. These methods help prevent the model from memorizing the training data too closely, promoting better generalization to unseen data scenarios.
Decision Trees in Marketing
Understanding Decision Trees
Decision trees in digital marketing are powerful tools for classifying and predicting customer behavior based on historical data. These trees consist of nodes representing decisions and branches representing outcomes or potential actions. Marketers can gain valuable insights into customer preferences and behaviors by analyzing these structures.
Application in Digital Marketing
Decision trees are crucial in segmenting customers based on demographics, browsing history, purchase patterns, and more. Marketers utilize decision trees to tailor personalized marketing strategies for different customer segments. For instance, businesses can optimize their advertising efforts for better results by identifying specific characteristics that lead to high conversion rates.
Advantages of Decision Trees
Scalability
Decision trees are highly scalable and can handle large datasets efficiently.
Interpretability
They clearly visualize decision-making processes, making it easier for marketers to understand and interpret the results.
Flexibility
Decision trees can accommodate both numerical and categorical data without the need for extensive data preprocessing.
Logistic Regression Applications
Customer Behavior
Logistic regression plays a crucial role in predicting customer behavior in digital marketing. By analyzing historical data on customer interactions, this model can forecast the likelihood of specific behaviors, such as making a purchase or clicking on an ad. This predictive capability enables marketers to tailor their strategies effectively.
Marketing Campaign Analysis
Logistic regression is important because of its ability to assess marketing campaign effectiveness. Marketers can utilize this model to evaluate the impact of various factors on campaign outcomes, such as email open rates or website conversions. Through detailed analysis, they can optimize their campaigns for better performance.
Real-World Examples
Logistic regression is widely employed in digital marketing in real-world scenarios. For instance, e-commerce platforms use this model to predict the probability of a customer making a purchase based on their browsing history and demographics. Social media platforms likewise utilize logistic regression to analyze user engagement with different types of content.
Support Vector Machines Explained
Definition
Support Vector Machines (SVM) are potent algorithms used in model classification for digital marketing. They excel in separating data points into different classes based on features.
SVMs work by finding the optimal hyperplane that best divides the dataset into distinct categories. This makes them highly effective in classifying customer data accurately.
Optimization of Marketing Strategies
By leveraging SVMs, marketers can gain valuable insights from large datasets—these insights aid in understanding customer behavior and preferences, enabling data-driven decisions.
The ability of SVMs to handle high-dimensional data efficiently allows marketers to identify patterns and trends that might be challenging to detect manually. This leads to optimized marketing strategies tailored to specific target audiences.
Efficiency in Handling Complex Datasets
One of the critical strengths of SVMs is their capability to efficiently handle complex marketing datasets. They can effectively deal with noise and outliers while maintaining high accuracy levels.
SVMs are particularly beneficial when dealing with non-linear data, where traditional methods like logistic regression may struggle. Their versatility in handling various data structures makes them a preferred choice for sophisticated marketing analysis.
Case Studies in Digital Marketing
Successful Implementation
Implementing model classification in digital marketing has shown significant success. For instance, a leading e-commerce company utilized random forest algorithms to predict customer preferences accurately. This allowed them to tailor their marketing strategies, resulting in a 30% increase in sales.
Outcomes and Impacts
Using neural networks by a social media platform revolutionized their ad targeting approach. By analyzing user behavior patterns, they achieved a 40% boost in click-through rates. This demonstrates the profound impact of advanced model classification techniques on marketing effectiveness.
Business Success
A global tech firm leveraged logistic regression to optimize its email marketing campaigns. They witnessed a doubling of their conversion rates through precise customer segmentation based on purchasing behavior. This showcases how strategic model classification can drive tangible business growth.
Improving Campaign Optimization
Data-Driven Decisions
Effective campaign optimization hinges on leveraging data-driven decision-making processes. By utilizing advanced model classification techniques, marketers can tap into valuable insights derived from data analysis. These insights enable a deeper understanding of consumer behavior and preferences, leading to more targeted and personalized marketing strategies.
Harnessing the power of data allows marketers to make informed decisions based on concrete evidence rather than assumptions. This approach enhances the accuracy of campaign optimization and increases the overall effectiveness of marketing initiatives. By analyzing historical data trends and patterns, marketers can identify key performance indicators (KPIs) and adjust their strategies in real time to maximize results.
Personalized Targeting
One key strategy for improving campaign optimization is personalized targeting using model classification. By segmenting audiences based on demographics, behavior, and preferences, marketers can tailor their campaigns to resonate with specific customer segments. This level of personalization enhances engagement rates and drives higher conversion rates.
Marketers can identify distinct audience segments with unique characteristics and behaviors through model classification techniques such as clustering and predictive modeling. This granular understanding enables them to create targeted content that speaks directly to each segment’s needs and interests. As a result, marketing campaigns become more relevant and impactful, leading to increased ROI and customer satisfaction.
Continuous Testing and Optimization
Optimizing marketing campaigns requires a commitment to continuous testing and optimization. Marketers can iterate on their strategies in real-time by implementing A/B testing methodologies and monitoring campaign performance metrics. Model classification plays a crucial role in this process by providing insights into which variables drive success and which need adjustment.
Marketers can leverage model classification algorithms to predict outcomes based on different scenarios and variables. This predictive capability allows them to proactively adjust their campaigns before issues arise. By constantly refining their targeting criteria, messaging, and channels based on data-driven insights, marketers can ensure that their campaigns are continually optimized for maximum impact.
Future Directions in Model Classification
AI Integration
AI and machine learning will revolutionize model classification in digital marketing, enhancing precision and efficiency. These technologies can accurately predict consumer behavior by analyzing vast datasets.
AI algorithms’ ability to adapt and learn from data trends will lead to more personalized marketing campaigns. This tailored approach can significantly increase conversion rates and customer engagement.
Emerging Technologies
Advancements in emerging technologies like blockchain and the Internet of Things (IoT) will play a crucial role in refining model classification strategies in the future. These innovations will give marketers deeper insights into consumer preferences and behavior patterns.
Summary
You’ve now explored the significance of model classification in digital marketing, delved into various types of models, assessed their strengths and weaknesses, and examined real-world applications like decision trees, logistic regression, and support vector machines. The case studies provided a practical understanding, emphasizing the role of these models in improving campaign optimization. The future promises exciting advancements in model classification that could revolutionize digital marketing strategies.
Frequently Asked Questions
What is the significance of model classification in digital marketing?
Model classification in digital marketing helps businesses predict customer behavior, optimize campaigns, and allocate resources effectively. Companies can use various models to enhance targeting strategies, improve ROI, and make data-driven decisions to stay ahead in the competitive landscape.
How do decision trees impact marketing strategies?
Decision trees simplify complex decision-making processes in marketing by visually representing possible outcomes. Marketers can use decision trees to identify critical factors influencing customer behavior, tailor messaging for different segments, and optimize campaign performance based on predictive insights.
Can you explain the role of logistic regression in digital marketing?
Logistic regression is crucial in digital marketing for predicting binary outcomes like click-through rates or conversion probabilities. It helps marketers understand relationships between variables, segment audiences effectively, and determine the likelihood of specific actions or responses from customers.
Why are support vector machines valuable tools in digital marketing?
Support vector machines excel in handling complex datasets and identifying patterns that might be challenging for other models. In digital marketing, SVMs enable precise customer segmentation, personalized recommendations, and accurate prediction of customer preferences for targeted campaigns.