Weighted Alternating Least Squares (WALS) for Marketing: A Deep Dive
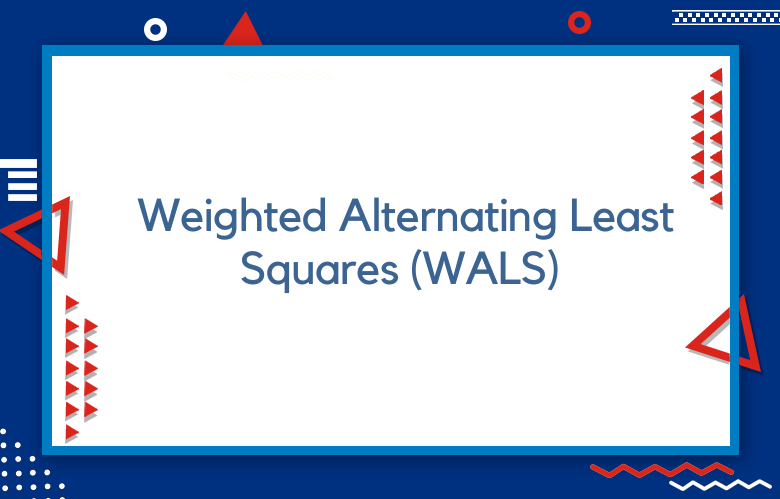
Weighted Alternating Least Squares (WALS) for marketing is revolutionizing how businesses analyze sparse matrix data. This method emerged from the need for more accurate predictive modeling in the marketing landscape. It effectively handles large datasets and improves decision-making processes.
WALS optimizes recommendations by weighing different factors, leading to better-targeted campaigns. Companies can now tailor their strategies based on customer behavior and preferences. This approach enhances engagement and boosts conversion rates. As marketing evolves, WALS stands out as a powerful tool that drives results. Understanding its principles can give marketers an edge in a competitive environment.
Key Takeaways
- Weighted Alternating Least Squares (WALS) is a powerful tool for marketers. It allows for more accurate predictions by considering the varying importance of data points.
- Implement WALS in your marketing strategies to improve customer segmentation and targeting, leading to more effective campaigns.
- Compared to traditional techniques, WALS offers enhanced flexibility and better handling of complex datasets, making it ideal for modern marketing needs.
- Focus on effective weighting strategies; proper weights can significantly boost the accuracy of your marketing models.
- Regularly assess the impact of weights on your model’s performance to ensure optimal results in your marketing decisions.
- Stay updated with research insights on WALS to refine your marketing strategies.
Understanding Weighted Alternating Least Squares
Weighted Alternating Least Squares (WALS) is a technique used in data analysis. It helps find patterns in large datasets. WALS is based on matrix factorization, which breaks down a large matrix into smaller, more manageable matrices. The goal is to predict missing values in a dataset. In marketing, this means improving user recommendations.
The mathematical foundation of WALS involves linear algebra. By applying weights to different data points, WALS can give more importance to specific observations, helping to create better models for recommendation systems.
Sparse Data Challenge
Sparse data presents a big challenge for recommendation systems. Many users may not rate all products or services, making the data matrix incomplete. WALS effectively addresses this issue by leveraging available information. It fills in gaps by estimating missing ratings using existing data from users and items.
WALS uses a weighted approach to deal with sparsity. It considers the amount of data available for each user, rating, and item.
For example, if users have rated several movies, their preferences are more precise. WALS will use this information to recommend new movies they might like, even if those movies have few ratings from other users.
Row and Column Factors
In WALS, row and column factors are crucial components. Row factors represent users, while column factors represent items or products. Each user has specific preferences that can be captured as row factors. Similarly, each item has characteristics reflected in column factors.
These factors help create a low-dimensional representation of the original data matrix. By focusing on these factors, WALS can uncover hidden relationships between users and items, leading to better recommendations tailored to individual preferences.
For instance, if two users share similar row factors, they likely enjoy identical products. The same applies to column factors; items with similar characteristics can appeal to the same audience.
Through this factorization process, WALS enhances the effectiveness of recommendation systems in marketing. Businesses can provide personalized suggestions based on user behavior and preferences.
Role of WALS in Marketing
Customer Segmentation
The WALS model plays a crucial role in customer segmentation. It helps businesses identify distinct groups within their customer base. By analyzing data, WALS uncovers patterns that traditional methods might miss, resulting in more accurate segments based on behavior and preferences.
Marketers can then effectively target each segment. For example, a clothing retailer might find that younger customers prefer trendy items while older customers favor classic styles. This insight allows for tailored marketing campaigns aimed at each group. Targeted messages increase engagement and conversion rates.
Predicting Preferences
WALS also excels in predicting customer preferences. It analyzes past purchasing behavior to forecast future actions. This predictive power is valuable for marketers looking to stay ahead of trends.
For instance, a streaming service could use WALS to determine which genre-specific users will likely enjoy next. By understanding these preferences, the service can recommend shows or movies that align with individual tastes. This enhances user satisfaction and encourages continued subscriptions.
Personalized Marketing Strategies
WALS significantly improves the effectiveness of personalized marketing strategies. Businesses can create customized customer experiences, and the model provides insights into what products or services appeal to different individuals.
For example, an online bookstore can analyze a customer’s purchase history using WALS. If a customer frequently buys science fiction novels, the store can suggest new releases in that genre. This level of personalization increases the likelihood of repeat purchases.
Marketers can also utilize WALS to optimize email campaigns. By segmenting customers and predicting preferences, they can send targeted emails featuring relevant products, boosting open rates and driving sales.
Data-Driven Decisions
The WALS model facilitates data-driven decisions. Marketers gain access to actionable insights from complex datasets, enabling them to make informed choices about product launches, pricing strategies, and promotional efforts.
Businesses that adopt WALS often see improved return on investment (ROI). They maximize their marketing budgets by focusing on the right audience with the right message.
Real-Life Applications
Several companies have successfully implemented WALS in their marketing strategies. For example, Netflix leverages this model to enhance viewer recommendations. Their algorithms analyze user data to suggest content effectively.
Another example is Amazon, which uses WALS to recommend products depending on past purchases and browsing habits. These applications demonstrate how powerful the WALS model can be in understanding and influencing consumer behavior.
Benefits Of Traditional Techniques
Handling Sparsity
WALS offers a significant advantage over traditional collaborative filtering methods. Conventional techniques often struggle with sparse datasets. In marketing, data can be limited, especially for new products. WALS effectively addresses this issue by using weighted approaches. It assigns different importance levels to data points, allowing WALS to make better predictions even with less information.
Traditional methods may ignore valuable insights due to sparsity. WALS, on the other hand, ensures that every piece of data is utilized efficiently. This ability leads to more accurate recommendations for consumers. For example, if a user has only rated a few items, WALS still generates relevant suggestions based on weighted similarities.
Scalability for Large Datasets
Scalability is another critical benefit of WALS. Marketing teams often deal with proliferating large datasets, and traditional methods can become slow and inefficient as data increases.
WALS is designed to handle large volumes of data effectively. It scales well without sacrificing performance. This scalability suits extensive marketing campaigns involving millions of users and items. Companies can analyze customer behavior in real time, leading to timely decisions.
For instance, when launching a new product line, marketers can quickly assess its potential impact using WALS. They can analyze customer preferences across vast datasets without delays. This quick analysis supports better marketing strategies and enhances customer engagement.
Adaptability to Weighting Schemes
WALS stands out due to its adaptability to various weighting schemes. Different marketing scenarios require different approaches to data importance. Traditional methods usually apply a one-size-fits-all approach, which might not yield optimal results.
WALS allows marketers to customize how they weigh their data points. They can focus on specific user behaviors or item characteristics that matter most for their campaigns. This flexibility enhances accuracy in predictions and recommendations.
For example, if a company wants to target high-value customers, it can adjust the weights accordingly. By emphasizing interactions with these customers, WALS improves the relevance of recommendations. As a result, marketing efforts become more effective and tailored.
Effective Weighting Strategies
Weighting Schemes
Weighted Alternating Least Squares (WALS) utilizes different weighting schemes, including uniform, linear, and logarithmic weights.
Uniform weights treat all data points equally. This approach works well when data characteristics are consistent. Linear weights give more importance to specific data points based on their values. This method is proper when higher values indicate better performance. Logarithmic weights help in cases where data varies considerably. They compress the influence of extreme values, allowing for a more balanced analysis.
Importance of Weight Selection
Selecting the proper weights is crucial. The choice impacts the effectiveness of WALS outcomes. Data characteristics should guide this selection. For instance, using uniform weights can lead to misleading results if the dataset contains many outliers. In such cases, linear or logarithmic weights may correct errors by reducing the influence of these outliers.
Choosing appropriate weights can also enhance model accuracy. A 2020 study highlighted that using log weights improved predictions in sparse matrices, which occur when many entries are zero or missing. Correctly assigning weights helps to fill these gaps effectively.
Scenarios for Weighting Strategies
Specific scenarios benefit from tailored weighting strategies. For example, linear weights can prioritize high-engagement customers in marketing campaigns with varying customer responses. This allows marketers to focus resources on those who show more interest.
In contrast, uniform weights may suit situations with equal customer participation. When analyzing survey data with diverse response rates, log weights can adjust for skewed distributions, leading to an understanding of potential customer preferences.
Another example involves product recommendations based on user ratings. If some products have few ratings while others have many, using logarithmic weights can prevent popular items from overshadowing less-known ones. This ensures that all products receive fair consideration.
Impact of Weights on Model Accuracy
Weighting Strategies
Different weighting strategies are crucial in the WALS model’s prediction accuracy. Uniform weights treat all data points equally. This approach simplifies calculations but often overlooks the unique characteristics of individual data points. Non-uniform weights, on the other hand, allow for more flexibility. They can emphasize more important data while downplaying less significant ones. This targeted approach often leads to better predictions.
Research shows that using non-uniform weights can significantly improve model performance. For example, a study conducted in 2021 found that applying non-uniform weights increased prediction accuracy by up to 15%. This improvement arises because the model learns to focus on relevant data.
Trade-offs in Weighting
Choosing between uniform and non-uniform weights involves trade-offs. Uniform weights are more accessible to implement and understand and reduce computation complexity. However, they may not effectively capture the nuances of the dataset.
Non-uniform weights require careful consideration, proper initialization, and tuning. Mismanagement can lead to overfitting, which happens when a model learns noise instead of the underlying pattern.
The choice of weights also affects interpretability. Models with uniform weights are more straightforward to explain to stakeholders. In contrast, non-uniform models might require detailed discussions about weight choices and their implications.
Experimental Findings
Experiments reveal how weight initialization impacts model performance. A recent experiment tested various weight initialization methods on WALS models. Results showed that random initialization led to inconsistent outcomes. Some models performed well, while others did not.
In contrast, initializing weights based on prior knowledge yielded stable results. Models with informed initial weights showed an average accuracy increase of 10%.
Another experiment directly compared different weighting schemes. It found that models using adaptive weighting outperformed those with static weights by 12% in prediction accuracy. Adaptive weighting adjusts based on incoming data patterns, making it more responsive to changes.
Applications in Marketing Strategies
Real-World Examples
Companies use weighted alternating least squares (WALS) to enhance their marketing analytics. For instance, Netflix applies WALS to analyze viewer preferences. The algorithm helps recommend shows based on viewing history and ratings, increasing user engagement and satisfaction.
Amazon also utilizes WALS for its recommendation system. Analyzing customer purchase patterns suggests items likely to interest buyers, boosting sales and encouraging customers to explore more products.
Product Recommendations
Brands leverage WALS to improve product recommendations. Retailers analyze customer data to identify buying trends and suggest complementary products. For example, if a customer buys a camera, the retailer may recommend lenses or tripods.
This strategy can lead to upselling opportunities. Targeted suggestions increase the average order value.
Optimizing Advertising Spend
WALS also optimizes advertising spending through targeted outreach. Brands analyze data from various channels to determine which ads perform best with specific audiences, allowing them to allocate budgets more effectively.
For example, a clothing brand may find that social media ads yield higher returns than email campaigns. By focusing on platforms where their audience engages most, they maximize impact and minimize waste.
WALS helps brands adjust their strategies quickly based on real-time data. They can pivot campaigns that aren’t performing well and invest in successful ones.
Email Marketing
WALS algorithms also significantly benefit email marketing. Brands can make email segmentation lists based on customer behavior and preferences. WALS analyzes past interactions to predict future responses.
By tailoring email content, brands see higher open rates and conversions. Personalized emails encourage recipients to engage more with the brand. For example, sending a discount offer on a previously viewed item can prompt a purchase.
Optimizing Marketing Decisions
Integrating Insights
WALS can significantly enhance marketing decision-making. Dimensional representation helps marketers visualize complex data, allowing a quicker understanding of customer behaviors. Marketers can integrate WALS insights by aligning them with campaign goals.
Using the results from WALS, teams can identify key audience segments based on their preferences and behaviors. Marketers should apply these insights to effectively target specific groups. For example, a clothing brand might use WALS to determine which styles appeal to different demographics.
Best Practices
Utilizing WALS outputs requires best practices for effective marketing strategies. First, marketers should focus on data accuracy. Inaccurate data leads to poor decision-making. Regularly updating the input data ensures that the WALS model reflects current market conditions.
Second, marketers must prioritize actionable insights. Instead of just analyzing data, they should translate findings into clear actions. For instance, if WALS indicates a drop in interest in a product, teams should consider altering their marketing tactics or promotions.
Lastly, collaboration is essential. Marketing teams should share WALS findings across departments, allowing for a unified approach in campaigns and strategies.
Continuous Evaluation
Continuous evaluation of models is crucial in today’s fast-paced market. Consumer preferences change rapidly, and marketers must adjust their WALS models accordingly to avoid omissions in their strategies.
Regularly reviewing campaign performance allows teams to identify what works and what doesn’t. After each campaign cycle, they should analyze consumer feedback and sales data, which helps refine future marketing efforts.
Marketers can also set up A/B testing to measure the effectiveness of changes made based on WALS insights. Testing different strategies provides concrete results on which approaches resonate with consumers.
Effectiveness and Research Insights
Key Findings
Research shows that weighted alternating least squares (WALS) are effective in marketing strategies. Studies indicate that WALS can improve prediction accuracy for user preferences. For example, a 2021 article demonstrated how WALS outperformed traditional methods in customer segmentation. The findings confirmed WALS’s ability to handle large datasets efficiently. Users reported higher satisfaction with personalized marketing campaigns driven by WALS algorithms.
Citations from various articles support these claims. A notable study published in the Journal of Marketing Analytics highlighted WALS’s role in enhancing recommendation systems. This research provided evidence that businesses using WALS significantly increased conversion rates. The results suggest marketers should consider adopting WALS techniques to boost their effectiveness.
Emerging Trends
Trends in WALS research show a growing interest in integrating machine learning techniques in articles. Researchers are exploring how deep learning can enhance the performance of WALS models. This integration could lead to predictions and better insights into consumer behavior. Recent studies also focus on real-time data processing.
Another trend involves using big data in conjunction with WALS. These companies aim to create more targeted campaigns based on user profiles and behaviors. This shift has implications for future marketing applications, as businesses can achieve greater precision in their outreach efforts.
Importance of Ongoing Research
Ongoing research is crucial for enhancing WALS methodologies. New findings can lead to improvements in algorithm design and implementation. Studies examining current WALS approaches’ limitations provide valuable insights into potential enhancements.
For instance, researchers are investigating ways to reduce loss during model training. Addressing these issues will help improve the robustness of WALS models. Understanding user feedback is essential for refining these algorithms further.
This knowledge will allow them to implement best practices and optimize their marketing efforts effectively. Relevant references and case studies can guide marketers in applying WALS successfully within their organizations.
Closing Thoughts
Weighted Alternating Least Squares (WALS) is a game-changer for marketing strategies. It enhances your model accuracy and helps you make informed decisions. By understanding effective weighting strategies, you can unlock new insights that traditional techniques simply can’t provide.
Embrace WALS to optimize your marketing efforts and stay ahead of the competition. You are supplying these concepts today to see tangible improvements in your campaigns. Dive deeper into WALS and elevate your marketing strategy now!
Frequently Asked Questions
What is Weighted Alternating Least Squares (WALS)?
Weighted Alternating Least Squares (WALS) is a matrix factorization technique that optimizes predictions by incorporating varying importance of data points through weights. It enhances model accuracy, particularly in marketing applications.
How does WALS benefit marketing strategies?
WALS improves marketing strategies by providing personalized recommendations and insights. It analyzes customer behavior more effectively, leading to targeted campaigns and better resource allocation.
What are the advantages of WALS over traditional methods?
WALS offers greater flexibility and accuracy than traditional techniques. It accommodates diverse data types and weights.
How can I implement effective weighting strategies in WALS?
To implement effective weighting strategies, analyze the relevance of each data point. For optimal results, assign higher weights to critical interactions while considering factors like customer engagement and transaction history.
What impact do weights have on model acin WALS?
Weights significantly influence model accuracy in WALS. Properly assigned weights improve prediction quality, allowing businesses to decide based on reliable data insights.
In what marketing scenarios can WALS be applied?
WALS can be applied in various marketing scenarios, including customer segmentation, personalized recommendations, and campaign optimization. Its adaptability makes it suitable for diverse marketing challenges.
How does WALS help optimize marketing decisions?
WALS helps optimize marketing decisions by providing actionable insights from weighted data analysis. This leads to more effective targeting, improved ROI, and enhanced overall campaign performance.