Retrieval Augmented Generation (RAG) for Marketing
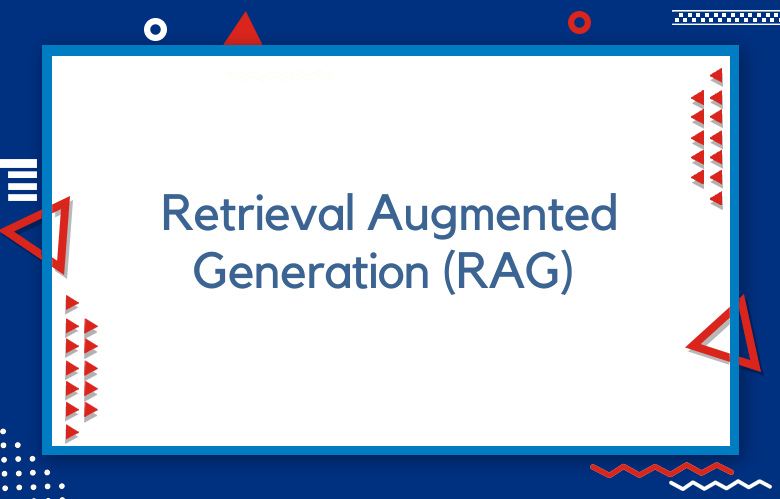
Marketing strategies evolve as technology advances. Today, marketers continuously implement AI to better understand their audience and serve relevant content. Retrieval augmented generation (RAG) is a technology that helps generate content with context.
RAG combines the benefits of traditional retrieval models with the capabilities of language generators to provide marketers with an optimized approach to content marketing. We will explore RAG and how it can transform your marketing strategy.
What is Retrieval Augmented Generation (RAG)?
RAG combines a traditional retrieval model with AI language generators. It uses content from the retrieval model to provide the AI language generator with context, enabling the generator to develop outputs that fit the context.
In RAG, the retrieval model retrieves a prompt relevant to the context from the database, such as a product description or keywords.
The prompt is then fed to the AI language model, which generates a highly relevant response to the context. The process is repeated multiple times until a fully optimized response is obtained.
How does RAG differ from Traditional Content Creation Methods?
Traditional content creation usually involves brainstorming ideas, drafting content, reviewing, and editing to create content. However, RAG takes a different approach.
Artificial intelligence retrieves relevant data from a knowledge pool and leverages that information to generate content based on a given set of keywords. This translates to a more streamlined content creation process, saving marketers time and resources.
How RAG can Help Your Marketing Strategy
RAG can help you create personalized content with ease. You can generate product descriptions, email marketing copy, and social media posts.
RAG can also optimize landing pages, match long-tail keywords with relevant pages, and improve the overall user experience. With RAG, you can get real-time feedback on your content and optimize it as you go.
Understanding Retrieval Augmented Generation (RAG)
Retrieval Augmented Generation (RAG) is an innovative AI tool that combines two separate yet highly advanced machine learning algorithms – BERT (Bidirectional Encoder Representations from Transformers) and GPT-3 (Generative Pre-trained Transformer).
RAG’s ability to draw from these two algorithms provides businesses with a powerful, machine-driven way to improve their marketing strategies.
The Role of RAG in Enhancing Customer Experience
RAG technology can drastically enhance the user experience by providing an effortless customer experience. This advanced technology will make customer queries more accurate and relevant, thus resulting in quick and satisfactory resolutions.
RAG can use previous interactions to suggest relevant products or services to the customer, which helps to enhance the overall customer experience and build brand loyalty.
Use Cases of RAG in Marketing
RAG can be utilized in various contexts, including e-commerce product recommendations, customer reviews and feedback, chatbots, content creation, and website optimizations.
Using RAG for different marketing strategies can help reduce workload and costs while enhancing efficiency and effectiveness. It can be a game-changer for small businesses that need more resources to serve customer queries and generate relevant content.
Introducing Retrieval Augmented Generation (RAG) for Marketing
As marketers strive to reach and engage with audiences, content creation has never been more foundational and fundamental.
The approach, therefore, becomes critical in ensuring that marketers can address this need. Still, with the evolving search algorithms and user-specific queries, finding a system that addresses these trends remains challenging.
Retrieval augmented generation, or RAG, is fast becoming a game-changer in the marketing industry. It is reshaping how businesses create, strategize, and deploy content. This article breaks down retrieval augmented generation and how it’s shaking up the marketing realm.
RAG is an innovative approach that combines language models with retrieval techniques to produce generated outputs that are grounded and informative. Oriental Trading, a company that primarily stocked party favors, used RAG to create content that answered customers’ questions.
Building a RAG Model for Your Marketing Strategy
The first step in building an RAG model is to choose the retrieval model, such as Elasticsearch, Solr, or Google Search. The selection should be based on the type of data being retrieved.
Next, the AI language model, such as GPT-3, should be chosen, and the training data for the model should be prepared. RAG model developers should carefully consider the context being fed to the model, which is critical to obtaining relevant content.
Retrieval Augmented Generation (RAG) for Marketing: A Deep Dive
As an industry, marketing is all about creating the right message and delivering it at the right time to the right people.
With the advancements in artificial intelligence and machine learning, marketers can now create optimized messaging with a personalized touch that resonates with their audience. One such approach gaining immense popularity recently is Retrieval-Augmented Generation (RAG).
We’ll dive deep into what RAG is, how it differs from traditional content creation methods, and the benefits it brings to the table. So, let’s jump right into it!
Challenges and Future of RAG in Marketing
Interpretation
One of the primary challenges with RAG reports is the potential for misinterpretation of data. These reports are meant to simplify complex data sets, but too much focus on the visual representation and less on the data itself can lead to incorrect conclusions.
Developing a standardized method of interpreting information while considering each campaign’s context is crucial.
This can be done by establishing protocols for measurement, defining the color coding and what each code represents, and ensuring everyone who uses the RAG reports has a clear understanding of them.
Repetition
Another concern is the repetition of data. When used excessively, RAG reports can become dull and repetitive, leading to a lack of engagement from marketers.
Overusing RAG reports can lead to unresponsive marketing teams indifferent to the data presented. Hence, RAG reports must be used strategically where they can have the most significant impact.
This can be done by setting clear objectives and tailoring your reports accordingly, emphasizing parts of the campaign data most critical to achieving those objectives.
Real-time Data
Real-time data is necessary to make informed decisions in today’s fast-paced marketing environment. Traditional RAG reports provide a snapshot of data at a particular time and do not offer real-time data tracking.
To overcome this challenge, consider implementing real-time reporting dashboards that provide a real-time data view. These dashboards can be linked to measurement metrics that trigger alerts whenever a critical KPI has been hit, allowing marketers to respond quickly and make informed decisions.
Automation Bias
Automation has allowed us to move towards a data-driven approach. While RAG reports offer us valuable insight, there is a danger of becoming reliant on automation.
It is crucial to avoid this “automation bias” and ensure our data is balanced and holistic. The future of RAG reports in marketing should not be limited to data automation but a dynamic human-centric approach to data interpretation wherever necessary.
Inclusion
Ensuring RAG reports are inclusive by engaging all stakeholders in a campaign is necessary.
Metrics and measures vary across different departments, and it is beneficial for all teams involved to have their perspectives reflected in reporting and used to diagnose potential issues or gaps.
A transparent approach to RAG reporting can ensure that all participants know the campaign’s status.
Conclusion
Retrieval augmented generation (RAG) is a new technology transforming how marketers create content. RAG models combine the best of traditional retrieval models and AI language generators to generate content that is highly relevant to the context provided.
With RAG, marketers can easily create personalized and optimized content, reducing the resources needed for manual content creation and staying ahead of the competition. Consider implementing RAG in your marketing strategy to stay ahead of the game!
Call: +91 9848321284
Email: [email protected]