How to use a Decision Tree Algorithm for Marketing Analytics
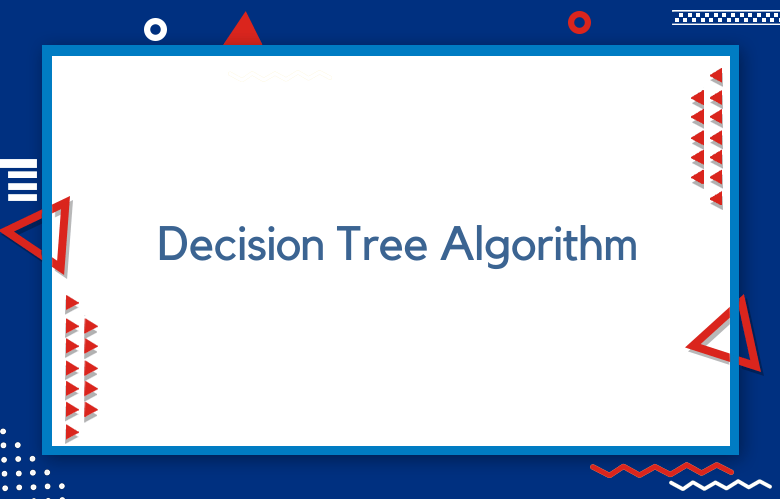
As a marketer, you know that data is essential to your success. But what data are you looking at, and how can you make the most of it? Decision tree algorithms can help you make sense of your marketing data and identify the most critical factors impacting your success. We will explain how to use a decision tree algorithm for marketing analytics and give some examples of how it can be used. Stay tuned – we’ll release a whole series of decision trees for marketing!
Regarding marketing analytics, decision tree algorithms can be handy tools. They allow you to visualize the relationships between different factors and how they impact your desired outcome. In this blog post, we’ll show you how to use a decision tree algorithm for marketing analytics and provide some examples of how it can be used in practice. We’ll also give you a few tips on how to get started with decision trees if you’re new to them. So, let’s get started!
What is a Decision Tree Algorithm?
A decision tree is a method to visualize the possible outcomes of events. It’s used in machine learning and data mining for classification, prediction, estimation, clustering analysis, and association rule learning.
A decision tree algorithm is supervised learning where the outcome is binary. Binary means 0 or 1, and the choice depends on many factors that add up to one value. The result can sometimes be wrong if it’s not weighted right,
A Decision Tree Algorithm is a way to explore decision situations and choose the best alternatives.
A decision tree algorithm is a way to make decisions based on analyzing logical outcomes. It’s called a tree because it creates one main question at each step, like tree branches that grow from an original trunk.
A decision tree algorithm can help you determine the best solution for your business. For example, this method can help you determine the best digital marketing strategy.
A decision tree algorithm is an artificial intelligence approach to solving a problem that contains decisions. It is used in machine learning, pattern recognition, and data mining.
A decision tree algorithm is a technique used to make decisions. It’s built from the top down, and each node can have multiple branches that lead to different answers.
Ways to Use Decision Tree Algorithm for Marketing Analytics
Most marketing will be carried out through personalized, dynamic, real-time marketing-optimization messaging delivered via the most valuable and appropriate communication channel and customized to connect with the particular consumer’s most significant interest and need at that moment.
Decision trees will be used to build models used for predictive analysis. Decision trees are a standard predictive model used in predictive analysis and can develop separate models for different customer segments.
Digital Marketers will use data-driven, algorithmically optimized marketing campaigns to reach buyers and effectively make sales.
Marketing analytics software will provide a user-friendly front-end interface to the powerful decision tree algorithms. It will have additional modules not part of the decision tree approach. Companies will have more computers that will help them make better decisions.
Research in this area will expand beyond the optimal use of past data into new regions of powerful modeling techniques such as genetic algorithms and neural networks. The study also included a dramatic example of how a “good” decision tree can forecast future market changes.”
The decision tree algorithms will make it easier for companies to develop segmentation models, customer lifetime value models, and customer-predicted scores for strategic marketing decisions.
The marketing analytics models will be based on artificial intelligence systems that mimic the human brain’s ability to take in complex data and make sense of it. These systems will help marketers make better decisions faster by providing consumers with what they want, when and how they want it, and how much they are willing to pay.
Wherever your preferences and habits leave a digital trace, they can be incorporated into an algorithm that predicts your next purchase or behavior. For example, a computer program could track your web searches and the websites you visit to determine your interests. It could check your traffic flow between websites to see what captures your attention. It could watch the goods you purchase online and those you take home from the mall. And it could look for clues on social networks to see what your friends are reading or frequenting.
Decision Tree Algorithms are used to make more accurate decisions in diverse fields such as business, politics, medicine, education, and engineering.
Marketing decisions will be dynamic and automated and contain expert intelligence features. Algorithms will continuously track changes in customer behavior and update the strategy constantly, selecting new offers and promotions for customers based on their reactions.
The “tree” will be the new visualization model for the Internet. It will be interactive, allowing users to zoom in and click on any portion of the “tree” to obtain more information. This technology will leverage the Internet’s power to provide visual representations of data and, simultaneously, allow customers to “consume” as much information as they can handle.
Decision trees analyze seemingly unrelated but informative data to make more informed decisions. They could be a tool for improving a company’s business strategy.
Big data technologies and algorithms will continue to influence the marketing landscape. For example, marketers will use data mining tools to uncover insights and other helpful information from their existing databases.
The power of big data will allow the development of even more complex and sophisticated models that will predict consumers’ future behavior based on their cohort’s behavior, indicating how consumers will behave, buy specific products, buy particular services, and so on.
Tree-based (decision tree) processing will be the platform for modeling and decision-making in complex problems as a marketer.
Big data sets will be analyzed using natural language programming logic to find segmented, targeted marketing opportunities that maximize return on marketing expenditures.
Decision-tree techniques will enable machine learning (ML) systems to incorporate domain-specific knowledge by automatically learning which variables affect the outcome under analysis and organizing the decision tree accordingly.
Machines and artificial intelligence could automate the gathering and analysis of marketing information. For example, a state-of-the-art neural network might scan Facebook news feeds and update its strategic plan based on what it sees.
The ubiquity of data and opportunities to analyze it with various data mining algorithms will make it harder to predict the next breakthrough business application.
Organizations and individuals will use these embodiments of decision trees to make automated marketing analytics decisions – such as who to market to, what to call and when to sell, who to try to recruit, what to say to whom, and how to combine new marketing data with existing data to make intelligent, optimized marketing analytics decisions.
Data mining and data-driven marketing will evolve to include interactive and immersive approaches to predicting customer responses.
Decision-tree algorithms and neural networks will be superimposed on surveys, focus groups, and customer loyalty cards. Customer information will be collected in the short term for immediate decisions about their experiences with goods or services. This will also provide marketers with a wealth of information stored long-term and added to information databases.
Companies will be able to understand their key customers, prospects, and their needs (Prospect’s Profile) and make better predictions about their decisions using new tools for better modeling (Decision Tree Algorithm) with different data (Deductive, Inductive) and view the results of those models simply with better visualization tools. New prediction tools will allow better marketing decisions based on data analysis.
Advertisers will use decision tree algorithms, market researchers, and retailers to target consumers better and maximize their products’ marketability and popularity.
Decision tree-based models might be more widely used for decision-making and gaining insightful information. These models may also be used more creatively to develop effective online marketing campaigns and better understand customer behavior.
The algorithm will be used to answer questions and make predictions. Instead of just saying, “People who watched this commercial are more likely to buy my product,” the tree may provide a little background on why it arrived at that conclusion. This could lead to a decision tree asking, “Do you want to watch a commercial, read a review, or see a friend’s post about a product before you buy it?”
The basic concept of the Marketing Analytics Decision Tree Algorithm will be the foundation of a new department in significant companies worldwide. Marketing Analysts on this team will use the flexibility of this algorithm to find the best approach for each situation. These marketing analytics will also be able to use Decision Tree Algorithms to find relationships among marketing variables. These relationships will help to optimize marketing strategies. Also, these marketing analytics will apply Decision Tree algorithms to see patterns and trends associated with the performance of marketing campaigns.
The analytics community is believed to focus on more advanced branches of decision trees. Beyond regression and classification, other natural extensions to decision trees include survival analysis and even graphical models such as Bayesian networks.
We will be able to identify patterns in purchasing behavior. This algorithmic pattern recognition can help determine if a particular customer will be interested in a product or service.
More efficient ways to target customers will make marketing more innovative, efficient, and cost-effective, resulting in a growing number of multichannel communication campaigns.
No enterprise will survive without intelligent algorithms that help make strategic and tactical decisions in natural language, whether spoken or written.
The decision-tree algorithm will be used even further than it is now. It will be indispensable to marketing managers, who will use it to answer essential questions about their products. Furthermore, it will be used to predict customers’ future behavior. Marketing managers will ask customers’ opinions about the products, understand customers’ needs, match specific customers’ tastes to certain products, and much more.
Top-level executives will have a personalized response to any infrastructure failure because the computer models will have been constructed from the top down to be analytically correct.
Computers will use the Decision Tree Algorithm to make predictions and recommendations. By learning from experience, they will become increasingly better at targeted selling. Expert systems and expert neural networking algorithms will help make predictions.
Decision Tree Algorithms will understand how different market variables interact and how a customer will interact with a brand. Decision Tree Algorithms will allow companies to make suggestions and recommendations to customers to increase their gratification due to higher brand-customer interaction.
Decision tree algorithms will be used to make timely marketing decisions in real time, such as when and where to market products or services. They will drive intelligent agent-driven electronic markets that aggregate buyers and sellers to facilitate economic exchange efficiently.
The decision tree algorithm will surpass its current uses of predicting credit risk, churn, and revenue. It will better predict users’ likelihood of buying products and responding to advertising. Companies will provide more relevant ads to users and possibly offer buyers a better experience using anonymized data and real-time information.
Data mining models have to know their limitations. For example, a decision tree has the inherent end of a monotonically decreasing reduction in error. Optimizing split criteria can get caught in a downward spiral, resulting in the tree trying to reduce the error by shrinking its leaf size.
Decisions may be automated with data processing algorithms such as decision trees, artificial neural networks, support vector machines, and evolutionary programming.
The analytical capability of Decision-Tree-based systems can be critical for identifying your firm’s most profitable customers and managing the risk associated with extending credit to those customers.
Customer data will influence decisions that affect the customer experience, and the findings will be massive.
Users of Decision Tree Algorithms will utilize a more advanced logic model of the processes, relying heavily on events and prescriptive decision-making to optimize the methods of these decisions.
Conclusion
Data-driven marketing is a valuable way to increase product sales and improve customer satisfaction. It takes the guesswork out of your decision-making process by using data from past campaigns to make better decisions for future ones.
If you’re interested in this type of engagement but don’t know where to start, we can help! Our expert team will provide valuable insights into how best to use the Decision Tree Algorithm for Marketing Analytics as part of an overall campaign plan that drives business growth and generates more revenue.
Contact us today if you want someone on your team who knows what they’re doing regarding data analysis!
Call: +91 9848321284
Email: [email protected]