How to use a Linear Regression for Marketing Analytics
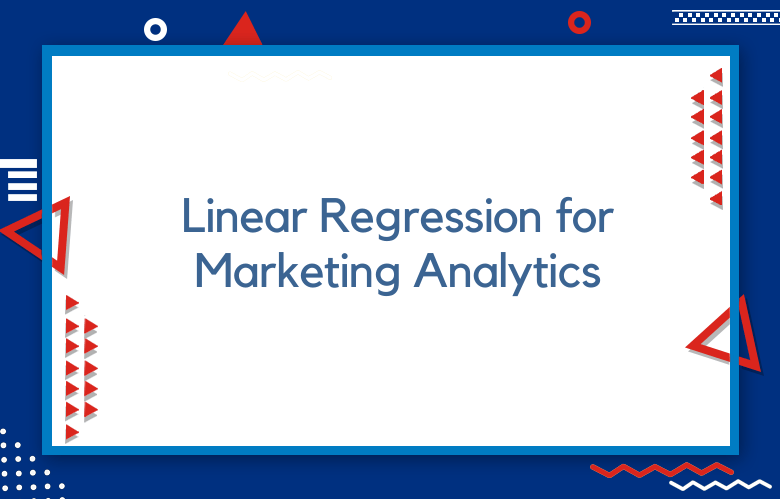
Linear Regression is a statistical analysis that can identify relationships between two or more variables. In marketing, this tool can evaluate the impact of various marketing campaigns on specific outcomes, such as website visits or revenue. By understanding the relationship between these factors, businesses can optimize their marketing efforts for the greatest return on investment. Here’s how to get started using linear Regression for your marketing analytics.
Linear Regression is a powerful statistical tool used for marketing analytics. We will use linear Regression to analyze customer data and improve your marketing tactics. We will also provide examples of how linear Regression can examine customer behavior and identify trends.
What is Linear Regression?
Linear Regression is a statistical method for modeling the relationship between variables when a linear association exists. The results of this model are estimates of the coefficients in a linear equation that describes the relationship between two variables.
Linear Regression is a method for finding a set’s best-fit line of data points.
In statistics and econometrics, linear Regression is a linear approach to modeling the relationship between a scalar dependent variable (the outcome) and one or more explanatory variables (predictors).
A straight line is the most straightforward possible curve, which will probably be helpful.
Linear Regression is a method for predicting the relationship between two variables. It’s usually represented by an equation of “y = ax + b.”
Linear Regression extends simple linear models and is a part of statistics. It helps us understand the relationship between independent variables (controlled ones) and dependent variables (the ones we want to predict).
Linear Regression is a powerful tool for analyzing data and predicting trends. Data scientists use linear Regression to determine how well their models perform, as it’s very accurate in predicting future events.
Linear Regression is a powerful tool that uses statistics to find an equation to predict your output given input. Linear Regression can be used for many applications, including finding sales data trends or determining how two variables vary.
How to use a Linear Regression for Marketing Analytics
- Linear Regression is a simple yet effective tool for predicting responses in marketing analytics.
- The British mathematician and statistician Sir Karl Pearson developed linear Regression. It is a method of finding a line that best fits a set of data points – in other words, it provides an equation to define the relationship between two variables.
- One of the most popular methods for predicting future trends is linear Regression. It’s a reliable and straightforward way to determine how changes in one variable affect another.
- Linear Regression is a straightforward statistical process that can be concluded. It’s also an easy marketing tool for predictive analysis.
- Using linear Regression to measure your marketing’s effectiveness is one of the best ways to determine if you’re in the right direction.
- Linear Regression is a formula that uses historical data to predict unknown values, even if they have complex relationships.
- Marketing analytics can help you make decisions that optimize efficiency and increase profits.
- Linear Regression is a statistical method for estimating the relationship between two variables in their predicted values.
Mastering Linear Regression for Precise Marketing Insights
Mastering linear Regression can give marketers valuable insights into the relationship between marketing efforts and business outcomes. This statistical technique allows marketers to analyze how changes in independent variables, such as advertising spend or social media engagement, impact dependent variables, such as sales or customer acquisition.
By understanding different variables’ coefficients and significance levels, marketers can identify which factors significantly influence their desired outcomes. Linear Regression enables marketers to make predictions and forecast future performance based on historical data, empowering them to make informed decisions and optimize their marketing strategies for maximum effectiveness. Overall, mastering linear Regression equips marketers with a powerful tool for gaining precise insights and driving data-driven decision-making in their marketing efforts.
Optimizing Marketing Strategies with Linear Regression Analysis
Optimizing marketing strategies with linear regression analysis involves using statistical techniques to understand and predict the relationships between marketing inputs (such as advertising spending, promotional activities, etc.) and outcomes (such as sales, customer acquisition, etc.). Here’s how it can be done:
Data Collection: Collect relevant data on marketing inputs and outcomes. This could include advertising spend, promotional activities, sales figures, customer demographics, market trends, etc. Ensure the data is accurate, complete, and properly formatted.
Identify Variables: Determine which variables are potential predictors of the outcome variable (e.g., sales). These could include advertising expenditure, social media engagement, email marketing campaigns, seasonality, competitor actions, etc.
Data Preprocessing: Clean the data by handling missing values, removing outliers, and transforming variables if necessary (e.g., log transformations for skewed distributions). Normalize or standardize variables to ensure they are on the same scale.
Build Linear Regression Models: Linear regression analysis is used to build models that predict the outcome variable based on the marketing inputs. Start with simple models and gradually add more variables to assess their impact on the outcome.
Assess Model Fit: Evaluate the model’s goodness-of-fit using metrics like R-squared, adjusted R-squared, and p-values. These metrics indicate how well the model explains the variability in the outcome variable and whether the predictors are statistically significant.
Interpret Results: Interpret the regression model coefficients to understand the relationship between marketing inputs and the outcome variable. Positive coefficients indicate a positive relationship, while negative coefficients indicate a negative relationship.
Validate the Model: Validate the model using techniques such as cross-validation or splitting the data into training and testing sets. This helps ensure the model generalizes well to new data and is not overfitting.
Optimization and Prediction: Once a satisfactory model is developed and validated, use it to optimize marketing strategies. For example, you can simulate the impact of different marketing scenarios (e.g., increasing ad spend, changing promotional strategy) on the predicted outcome variable (e.g., sales). This lets you identify the most effective marketing strategies for achieving your objectives.
Monitor and Refine: Continuously monitor the performance of your marketing strategies and refine your regression model as new data becomes available. This ensures that your marketing efforts align with your business goals and market dynamics.
By leveraging linear regression analysis, marketers can gain valuable insights into the effectiveness of their marketing strategies and make data-driven decisions to optimize their campaigns for maximum impact and ROI.
Achieving Marketing Success through Data-Driven Linear Regression Analysis
Conclusion
Linear ReRegressions a powerful marketing analytics tool but can also be intimidating. If you’re not sure how to use linear ReRegressionr what type of model would work best for your business needs, contact us! We have an expert team to help with any aspect of data analysis- from determining which metric will provide more accurate insights into customer behavior patterns to designing an A/B test. Click here if you want someone who has experience in Marketing Analytics Consulting.
Call: +91 9848321284
Email: [email protected]