How to use a Naive Bayes for Marketing Analytics
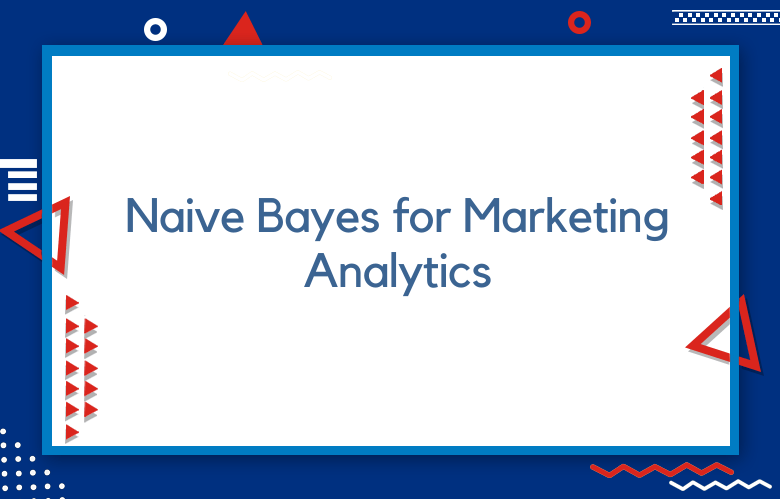
Naive Bayes is a popular classification algorithm widely used in data mining and machine learning. In marketing analytics, this powerful tool can help businesses gain valuable insights from their data, enabling them to make data-driven decisions and optimize their marketing strategies.
This article will explore how to utilize Naive Bayes for marketing analytics, discuss its critical applications, and offer practical tips on incorporating this method into your data analysis workflow.
How to use a Naive Bayes for Marketing Analytics
Using Naive Bayes for Marketing Analytics involves leveraging this simple yet powerful algorithm to understand and predict customer behaviors based on historical data. Here’s a basic introduction to get you started:
Understanding Naive Bayes
Naive Bayes is a probabilistic machine learning algorithm based on Bayes’ Theorem, which calculates the probability of an event based on prior knowledge of conditions that might be related to the event. It’s called “naive” because it assumes that all predictors (or features) are independent of each other, a condition rarely met in real life, but simplifies the calculations.
Application in Marketing Analytics
In marketing analytics, Naive Bayes can perform customer segmentation, targeting, churn prediction, and personalized marketing tasks. By analyzing customer data, Naive Bayes can help predict how likely a customer is to respond to a specific marketing campaign, buy a product, or churn.
Steps to Use Naive Bayes in Marketing Analytics
Data Collection: Gather data relevant to your marketing question. This could include customer demographic information, past purchase behavior, interaction with marketing campaigns, and other relevant metrics.
Data Preprocessing: Clean and preprocess the data. This involves handling missing values, encoding categorical variables, and possibly normalizing or standardizing numerical values.
Feature Selection: Select relevant features that could influence the outcome you’re interested in. For example, features might include age, subscription length, usage frequency, and responses to past marketing campaigns to predict customer churn.
Model Training: Split your data into training and test sets. Use the training set to train your Naive Bayes model. The model will learn the probabilities of different features associated with various outcomes (e.g., purchasing).
Evaluation: Test the model on your test set to evaluate its performance. Standard metrics for evaluation include accuracy, precision, recall, and the F1 score. Adjust your model or preprocessing steps based on these metrics to improve performance.
Deployment and Monitoring: Once the model’s performance is satisfied, deploy it in a real-world setting, where it can start predicting customer behavior based on new data. Continuous monitoring is crucial to ensure the model remains accurate over time.
Benefits and Limitations
Benefits: Naive Bayes is easy to implement, fast, and efficient with large datasets. It works well with categorical data and is effective even with small training data.
Limitations: The assumption of independent predictors is often unrealistic in marketing data, which can affect the accuracy. Additionally, it may not perform well with numerical features compared to other algorithms.
Unleashing the Power of Naive Bayes in Marketing Analytics
Naive Bayes, a robust yet straightforward classification algorithm, has found extensive application in marketing analytics due to its effectiveness in handling large datasets and its ability to make quick predictions. Based on Bayes’ Theorem, this algorithm is particularly adept at categorizing data into different groups, making it an invaluable tool for marketers seeking to understand and predict consumer behavior.
In marketing analytics, Naive Bayes helps segment customers based on their purchasing history, demographic data, and interaction with marketing campaigns. It can efficiently predict the likelihood of a customer buying a product, subscribing to a service, or responding to a particular advertising campaign. This predictive capability enables marketers to tailor their strategies to different customer segments, optimizing their efforts for higher engagement and conversion rates.
Moreover, Naive Bayes is straightforward to implement and doesn’t require as much computational power as some of the more complex machine learning models, making it an accessible option for marketers who may not have extensive technical backgrounds. Its assumption of feature independence, while a simplification, often proves to be effective in practical applications, particularly in text classification tasks such as sentiment analysis and spam detection in customer feedback and emails.
Step-by-Step Guide: Implementing Naive Bayes for Marketing Insights
Implementing Naive Bayes for marketing insights involves a series of steps, from data preparation to model evaluation and interpretation. Here’s a step-by-step guide to get you started:
Step 1: Define Your Objective
Clearly define what marketing question you’re trying to answer with Naive Bayes. Are you predicting customer churn, segmenting customers, personalizing offers, or something else?
Step 2: Collect and Prepare Your Data
Data Collection: Gather historical marketing and customer data. This may include demographics, purchase history, campaign interactions, website engagement metrics, etc.
Data Cleaning: Clean your data to handle missing values, remove duplicates, and correct errors.
Feature Engineering: Create new features that might be relevant to your prediction. For example, a ‘days since last purchase’ feature could be helpful for churn prediction.
Data Preprocessing: Convert categorical variables into a format that can be provided to the model (e.g., one-hot encoding). Normalize or standardize numerical features if necessary.
Step 3: Choose the Right Naive Bayes Model
Depending on your data type, choose the appropriate Naive Bayes classifier. Common types include:
Gaussian Naive Bayes: Suitable for continuous data where the features are assumed to be normally distributed.
Multinomial Naive Bayes: Good for discrete data. It is often used in text classification, where features are word counts or frequencies.
Bernoulli Naive Bayes: Used for binary/boolean features.
Step 4: Split Your Data
Split your dataset into a training set and a test set. A typical split ratio is 80% for training and 20% for testing.
Step 5: Train Your Model
Train your Naive Bayes model using the training dataset. This involves the model learning the probabilities of different features associated with various outcomes.
Step 6: Model Evaluation
Evaluate your model’s performance using the test set. Standard metrics include accuracy, precision, recall, and F1 score.
Confusion matrix: Provides insights into the types of errors made by your model.
Step 7: Model Tuning (If Necessary)
Based on the evaluation, you may need to adjust your feature selection and data preprocessing or try a different Naive Bayes model type.
Step 8: Gain Insights and Make Predictions
Use the trained model to gain insights into customer behavior or predict future outcomes. For instance, understand which features most influence the likelihood of a customer purchasing or churning.
Apply the model to new customer data to make predictions or segment customers.
Step 9: Deployment and Monitoring
Deploy your model in a live environment to start making real-time predictions or insights.
Continuously monitor its performance and update the model when new data becomes available, or its performance degrades.
Leveraging Naive Bayes for Precise Targeting in Marketing Campaigns
Leveraging Naive Bayes for precise targeting in marketing campaigns allows marketers to harness the power of predictive analytics to enhance the relevance and effectiveness of their efforts. Applying this probabilistic model will enable the analysis of vast amounts of customer data to predict behaviors and preferences, enabling more personalized and targeted marketing strategies.
Understanding Customer Segments
Naive Bayes excels in classifying customers into segments based on their past interactions, demographic information, and purchasing behaviors. This segmentation helps marketers understand different groups’ specific needs and preferences, allowing for more tailored marketing messages and offers.
Predictive Personalization
The algorithm’s predictive capabilities extend to forecasting customer responses to various marketing stimuli. For instance, it can predict the likelihood of a customer clicking on a particular ad, making a purchase, or engaging with a specific type of content. This level of personalization ensures that marketing efforts resonate more deeply with potential customers, significantly enhancing the chances of conversion.
Enhancing Campaign Efficiency
By focusing on segments and individuals most likely to respond positively, Naive Bayes helps marketers allocate resources more efficiently. Instead of a broad, “one-size-fits-all” approach, marketing campaigns can be strategically directed toward those with the highest propensity to engage, optimizing budget expenditure and maximizing ROI.
Real-time Adaptability
The relative simplicity and speed of the Naive Bayes algorithm make it well-suited for real-time marketing decisions. Marketers can quickly adjust campaigns based on ongoing customer interactions and feedback, ensuring marketing messages remain relevant and timely.
Multichannel Optimization
Naive Bayes can analyze customer data across various channels, from social media interactions to email responses. This comprehensive view enables marketers to optimize their strategies across all touchpoints, ensuring a cohesive and personalized customer journey.
Challenges and Considerations
While Naive Bayes offers numerous advantages, marketers should be mindful of its limitations, such as the assumption of feature independence, which may not always hold in complex datasets. Additionally, the quality and relevance of the input data significantly impact the model’s predictions, necessitating thorough data cleaning and preparation.
Maximize Your Marketing ROI with Naive Bayes Algorithm
Maximizing your marketing ROI (Return on Investment) involves making informed decisions about where to allocate your resources for the best possible returns. The Naive Bayes algorithm, with its simplicity and effectiveness, can play a pivotal role in achieving this by providing data-driven insights into customer behavior and preferences. Here’s an introduction to how Naive Bayes can help you boost your marketing ROI:
Understanding the Basics
The Naive Bayes algorithm is a probabilistic machine learning model that applies Bayes’ Theorem with the “naive” assumption of conditional independence between every pair of features. Despite its simplicity, Naive Bayes can yield highly accurate predictions, making it a valuable tool for marketing analytics.
Key Applications in Marketing
Customer Segmentation: Naive Bayes can analyze customer data to identify distinct segments based on purchasing behavior, preferences, and demographics. By understanding these segments, marketers can tailor their strategies to target each group.
Predictive Analytics: It predicts customer churn, response to marketing campaigns, and potential purchases. These predictions enable marketers to focus their efforts where they are most likely to succeed, enhancing campaign effectiveness and ROI.
Personalization: By understanding individual customer behaviors and preferences, Naive Bayes helps personalize marketing messages and offers, leading to higher engagement rates and customer satisfaction.
Content Optimization: In digital marketing, Naive Bayes optimizes content and recommendations, ensuring users see the most relevant ads and product recommendations, increasing conversion rates.
Advantages for Marketing ROI
Cost Efficiency: Naive Bayes is computationally inexpensive, making it accessible even for small businesses with limited resources.
Speed: It can quickly process large datasets, providing timely insights crucial for fast-paced marketing decisions.
Versatility: It suits various data types and marketing scenarios, from email campaigns to social media analytics.
Implementing Naive Bayes for Enhanced ROI
Data Collection and Preparation: Gather comprehensive customer and campaign data. Clean and preprocess this data to ensure it is ready for analysis.
Model Training and Testing: Choose the appropriate type of Naive Bayes model based on your data, train it with historical data, and validate its performance using real-world test cases.
Insight Generation: Use the model to generate actionable insights. This could involve identifying high-value customer segments, predicting the success of various marketing strategies, or personalizing customer interactions.
Action and Optimization: Apply these insights to refine your marketing strategies. This could mean reallocating the budget to high-performing channels, personalizing communication, or adjusting your product recommendations.
Continuous Learning: Marketing environments are dynamic. Continuously feed new data into your model to refine its predictions and adapt to changing trends and customer behaviors.
Understanding the Basics: Naive Bayes for Marketing Professionals
For marketing professionals venturing into data analytics, understanding the basics of Naive Bayes is akin to adding a powerful tool to their arsenal. This probabilistic algorithm, grounded in the principles of Bayes’ Theorem, offers a straightforward yet practical approach to classifying and predicting consumer behavior, making it an invaluable asset in the data-driven landscape of modern marketing.
At its core, Naive Bayes operates on the premise that each feature (or piece of data) about a customer or potential customer contributes independently to the outcome. For instance, in the context of email marketing campaigns, it might consider factors like the time an email is opened, the types of products previously purchased, and the frequency of engagement with past emails, all as separate influencing factors on the likelihood of a future purchase.
Conclusion:
Naive Bayes is a powerful tool that offers businesses valuable insights to drive strategic decision-making and improve overall performance when applied to marketing analytics. Its simplicity, effectiveness, and ability to handle large datasets make it an attractive choice for marketers seeking to harness the potential of data in their campaigns.
By understanding the principles behind Naive Bayes and its applications in marketing analytics, businesses can gain a competitive edge and propel their marketing efforts to new heights of success. As the marketing landscape evolves, embracing data-driven strategies like Naive Bayes will be crucial for staying ahead of the curve and achieving sustainable growth.
Call: +91 9848321284
Email: [email protected]