LLM Optimization: Boost SEO with GAIO for LLM Optimization
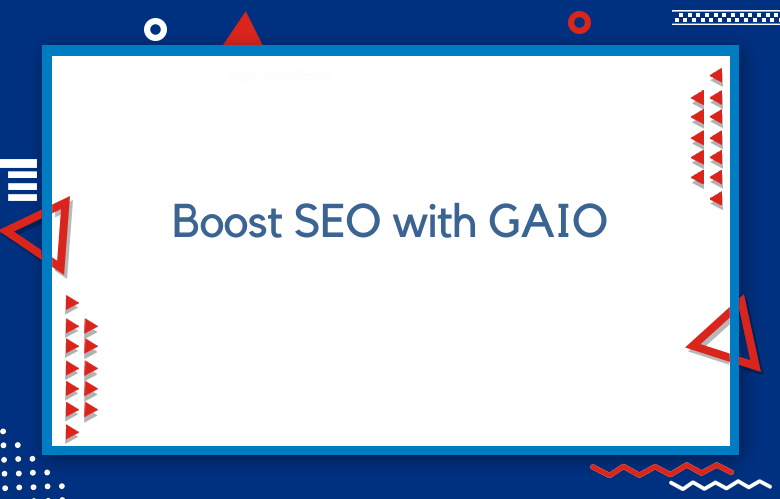
The most popular search engine used today is Google, serving over 5.6 billion searches daily. This number means that Google is the biggest platform businesses can leverage to improve their online presence and attract potential customers.
But, with the high level of competition, every business needs to find a way to stand out in search rankings. One way of doing this is through Local Landing Page (LLP) and Google Analytics SEO insights (GAIO) for LLM optimization. In this article, we’ll review the concepts of LLP and GAIO and how they can help you rank on a local search engine results page.
As an online business owner or a blogger, getting traffic is essential to your business or content success. One of the ways to achieve this is by maximizing your search engine optimization (SEO) efforts. In digital marketing, Local Landing Pages (LLM) are essential to generate traffic to a specific location or region.
To take full advantage of LLM, you need a strategy that includes keyword research and competitor analysis. In this post, we’ll explore how using GAIO can boost your SEO efforts regarding LLM optimization.
Understanding LLM Optimization: Influencing Generative AI Outputs
What is LLM Optimization?
LLM optimization, or Latent Layer Model optimization, is a technique used to influence the output of generative AI models. This process involves training a model to generate more accurate and realistic images by optimizing the latent space in which the images are generated.
By optimizing this space, it is possible to create more complex and detailed illustrations that better reflect real-world data.
How Does LLM Optimization Work?
The process of LLM optimization begins with an initial image being generated by a generative AI model. The model then uses an algorithm to optimize the latent space in which the idea was developed, resulting in a more accurate and realistic representation of the data. This process can be repeated until the desired level of accuracy and realism is achieved.
Benefits of LLM Optimization
LLM optimization has several benefits for generative AI models, including improved accuracy and realism in generated images, faster training times due to fewer parameters needing to be optimized, and increased flexibility when working with different datasets.
LLM optimization can help reduce overfitting by allowing models to focus on specific features rather than attempting to generalize across all datasets.
Challenges of LLM Optimization
While many benefits are associated with LLM optimization, some challenges must be considered when using this technique. For example, if not enough data is available for training or if the dataset is too large for efficient processing times, good results from this type of optimization may not be possible.
As with all machine learning techniques, there is always a risk that overfitting will occur if too much emphasis is placed on specific features or datasets without appropriately generalizing across multiple data sources.
Applications of LLM Optimization
LLM optimization can be used in many applications where generative AI models are employed, such as computer vision tasks such as object recognition or image segmentation, natural language processing tasks such as text summarization or sentiment analysis, and medical imaging tasks such as tumor detection or disease diagnosis.
This technique can also be used in other areas, such as robotics or autonomous vehicle navigation systems, where it can improve accuracy and realism in simulated environments while reducing overfitting risks associated with traditional machine learning techniques like deep neural networks (DNNs).
Limitations of LLM Optimization
Despite its many benefits, some limitations associated with LLM optimization should be considered before implementing this technique for any application scenario.
For example, because this technique requires a large amount of data for training purposes, it may not always be feasible for specific applications where limited amounts of data are available; due to its complexity, it may require additional computing resources, which could increase costs significantly depending on the application scenario at hand.
The Future of SEO: Influence Generative AI Outputs with LLM Optimization
Improved Natural Language Processing
Natural language processing (NLP) is the ability of a computer to understand and interpret human language. With advances in machine learning, natural language processing is becoming more accurate and efficient. This will significantly impact SEO, allowing search engines to understand user intent better and deliver more relevant results.
Automation of Keyword Research
Keyword research is essential to SEO, as it helps you identify the most effective keywords for your website. With AI-driven automation, keyword research can be done quickly and accurately, allowing you to focus more on other aspects of SEO, such as content creation and link building.
Enhanced Content Optimization
Content optimization is one of the most important aspects of SEO, as it helps search engines determine which pages are most relevant to a particular query. AI-driven tools can help optimize content by suggesting changes that improve relevance and ranking potential.
Increased Use of Structured Data Markup
Structured data markup is code that can be added to web pages to provide additional information about the page’s content to search engines. This can help search engines better understand the content on a webpage and improve its ranking potential.
AI-driven tools can automate the process of adding structured data markup, making it easier for website owners to use this powerful SEO technique.
Improved Image Recognition Technology
Image recognition technology allows computers to recognize objects in images and videos, which has numerous applications for SEO. For example, image recognition technology can generate alt text for images, helping them rank higher in image search results. It can also be used for video transcription or captioning, improving their discoverability in video search results.
Voice Search Optimization
Voice search is becoming increasingly popular due to its convenience and accuracy. To ensure your website ranks well in voice searches, you need to optimize your content for conversational queries rather than traditional keyword-based ones. AI-driven tools can analyze voice queries and suggest changes to make your content more relevant for voice searches.
Contextual Link Analysis
Link analysis is an integral part of SEO, as links are one of the primary signals search engines use when ranking websites in organic search results. AI-driven tools can analyze links on your website or from external sources and provide insights into how they affect ranking potential so that you can make informed decisions about link-building strategies moving forward.
Exploring the Intersection of SEO and Generative AI: LLM Optimization
The intersection of Search Engine Optimization (SEO) and Generative Artificial Intelligence (AI) is a fascinating area of study that offers numerous opportunities for businesses and researchers alike. One key area of interest in this field is LLM or Language Model Optimization.
LLM Optimization involves fine-tuning the algorithms used in natural language processing (NLP) to improve AI models’ ability to generate high-quality, relevant content optimized for search engines. This can be achieved through various techniques, including incorporating SEO keywords and metadata, developing specific training data sets, and using advanced machine learning algorithms.
Unlocking the Potential of Generative AI: Influence with LLM Optimization
Generative Adversarial Networks (GANs) are among the most powerful and versatile tools in modern artificial intelligence research, and they are used for various applications, including image synthesis, data augmentation, and domain adaptation. However, training GANs is challenging due to several issues, including mode collapse and instability.
Recent research has focused on utilizing learned latent spaces to manipulate the output of GANs. In the literature, this is known as latent space interpolation. However, more than interpolation is required to achieve the desired results, and it is limited to linear variations between two points in the latent space.
Influence functions have been proposed to improve the robustness and versatility of GANs. Influence functions allow more precise manipulation of the generated data distribution by maximizing or minimizing specific objectives. However, influence functions require access to the gradients of the generator network, which can be computationally expensive.
Strategies and Challenges in LLM Optimization for Generative AI
Developing an Optimal Training Process
An effective training process for generative AI is essential to optimize the system’s long-term memory. This process should involve supervised and unsupervised learning techniques, reinforcement learning, and transfer learning. It is necessary to ensure that the data used for training is diverse and representative of the real-world environment.
Reducing Overfitting
Overfitting occurs when a model memorizes the training data instead of generalizing it. Regularization techniques such as dropout and weight decay during training are essential to reduce overfitting. Monitoring the model’s performance on training and test sets throughout training is necessary to ensure that overfitting does not occur.
Utilizing Memory Augmentation Techniques
Memory augmentation techniques can help generative AI systems remember more information over time. These techniques include using external memory modules, such as hard disks or flash drives, and recurrent neural networks (RNNs) or long short-term memory (LSTM) networks to store information in a structured form over time.
Exploring New Architectures
Exploring new architectures for generative AI systems can improve their long-term memory optimization capabilities. For example, Generative Adversarial Networks (GANs) are effective at generating high-quality images from limited data sets and can also be used in LLM optimization tasks.
Transformer models have been used successfully for language modeling tasks and could be applied to other LLM optimization tasks.
Utilizing Transfer Learning Techniques
Transfer learning involves transferring knowledge from one domain to another to improve performance on a specific task without training a model from scratch on that task’s data set.
This technique has been successfully used in various areas, such as computer vision and natural language processing (NLP). It could also be applied in LLM optimization tasks to improve performance without requiring large data or extensive training timescales.
Incorporating Human Knowledge
Incorporating human knowledge into generative AI systems can help them better understand complex concepts and make more accurate predictions over time.
For example, incorporating expert knowledge into decision trees or rule-based systems can help them make better decisions when faced with unfamiliar situations or contexts that they have not encountered before during their training phase.
Leveraging Existing Datasets
Using existing datasets can provide valuable insights into how generative AI systems should behave when presented with scenarios or contexts outside their usual scope of understanding.
By leveraging existing datasets, organizations can quickly build a library of knowledge to use when creating their custom models for LLM optimization tasks. This approach allows organizations to utilize existing research while controlling their models’ training and deployment.
Optimizing Computational Resources
Optimizing computational resources is vital in optimizing LLM for generative AI systems. Organizations can significantly reduce training times by utilizing hardware accelerators such as GPUs, FPGAs, TPUs, etc., while still producing highly accurate results.
Distributed computing frameworks such as Apache Spark allow organizations to leverage multiple machines in parallel, allowing them to take advantage of larger datasets than would otherwise be possible with traditional computing architectures.
What is LLM Optimization or GAIO, and How Does It Work?
LLM optimization or GAIO (Generalized Adaptive Input-Output) is a robust optimization methodology that combines physics-based modeling with machine-learning techniques to optimize complex systems and improve their performance.
The approach is well-suited for designing and optimizing mechanical, electrical, chemical, and thermal systems. GAIO can be applied to various design problems, including product design, manufacturing optimization, and system integration.
The GAIO methodology’s core lies in optimizing a system’s input-output maps. These maps describe how a system responds to changes in its input parameters, such as operating conditions, material properties, or boundary conditions.
By optimizing these maps, engineers can achieve optimal performance for a given system while improving reliability, efficiency, and safety.
The Role of Training Data in LLM Optimization and Generative AI
Training data is crucial in optimizing machine learning models and generative AI. The quality and quantity of training data directly affect the accuracy and effectiveness of these models. Training data is a data set that teaches machine learning algorithms how to make predictions. It is the foundation upon which these models are built.
In supervised learning, the most common type of machine learning, training data is labeled, meaning each data point is associated with a specific output or response variable. The algorithm aims to learn how to predict this output based on the input data.
Therefore, the quality of the training data determines how well the algorithm can generalize and make accurate predictions on new, unseen data. If the training data is incomplete or biased, the algorithm might struggle to make accurate predictions on new data points.
Semantic Understanding and Entity Extraction in LLM Optimization
In the field of legal document processing, understanding the context and extracting specific entities from legal documents has become increasingly important. Legal Language Modeling (LLM) is a particular area of research that aims to build models that can accurately understand and interpret legal language.
One critical challenge in LLM optimization is developing effective techniques for semantic understanding and entity extraction in legal documents.
Semantic understanding involves analyzing the syntax and structure of legal language to identify the relationships between different words and phrases. This requires a deep understanding of legal terminology and concepts and knowledge of the specific legal domain for which the model is being developed.
Influencing SEO and Search Engine Positioning with LLM Optimization
LLM optimization is a strategic technique to help businesses improve their SEO and search engine positioning. Specifically, LLM optimization involves targeting long-tail keywords, creating high-quality content, and optimizing title tags, meta descriptions, and other on-page elements.
By implementing these strategies, businesses can increase their visibility in search engine results pages (SERPs), attract more traffic to their website, and ultimately improve their bottom line.
One key benefit of LLM optimization is that it allows businesses to target concrete search terms relevant to their products or services. For example, instead of targeting a generic keyword like “shoes,” a shoe retailer might target a long-tail keyword like “comfortable running shoes for women.”
By doing so, they’re more likely to attract visitors explicitly searching for the type of product they offer, which can lead to higher conversion rates and increased revenue.
The Prospects and Resources Required for Successful LLM Optimization in SEO
LLM optimization can be a critical aspect of building a successful online presence. It involves tailoring your website’s content and structure to improve its search engine ranking, making it easier for potential customers to find you through search queries. However, like any aspect of digital marketing, LLM optimization requires specific prospects and resources to succeed.
Firstly, one of the most critical prospects required for successful LLM optimization is a deep understanding of your target audience. By understanding your target audience, you can tailor your website’s content to meet their needs and preferences, improving its relevance to search engine algorithms.
A comprehensive understanding of your target audience can help you identify the precise keywords and phrases your potential customers are searching for, allowing you to optimize your website’s content accordingly.
Conclusion:
LLM optimization is essential for businesses and bloggers looking to maximize their search engine presence for specific regions. With GAIO, you can use AI-powered data analysis to optimize your LLM content and generate more traffic.
GAIO provides valuable insights on improving your content, meta tags, keywords, and backlink structure by analyzing user behavior, competitor data, and search engine results.
GAIO offers other features to help you analyze website traffic, identify trends, and monitor user behavior. So, if you want to improve your SEO efforts, try GAIO and take advantage of the power of AI in your LLM optimization strategy.
GAIO for LLM optimization is a must-have tool for businesses that want to improve online visibility and generate more leads. This system is easy to use and provides actionable insights that can help you optimize your website’s content and improve your SEO.
With GAIO for LLM optimization, you can stay ahead of the competition and rank higher in search engine results pages. So what are you waiting for? Sign up for GAIO for LLM optimization today and watch your website’s SEO soar.
Call: +91 9848321284
Email: [email protected]