The Power of Retrieval Augmented Generation (RAG) in AI
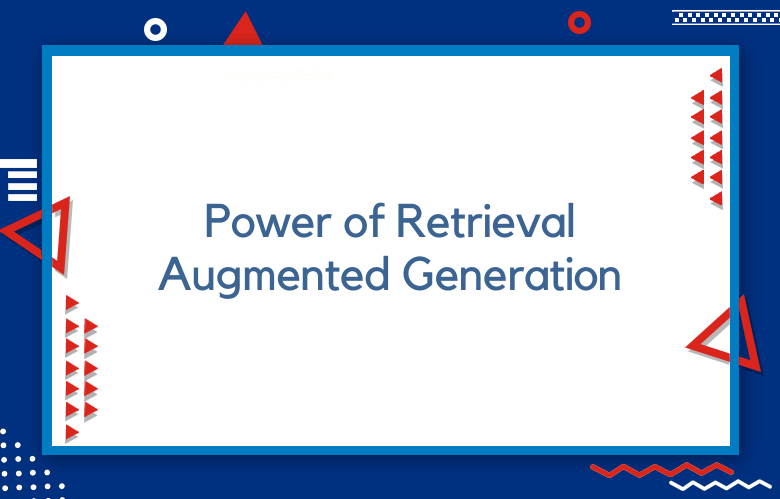
Artificial Intelligence (AI) has come a long way in recent years. AI is revolutionizing various industries with its capabilities, from self-driving cars to virtual assistants like Siri and Alexa.
However, AI still struggles with understanding context and generating relevant content. This is where Retrieval Augmented Generation (RAG) comes into play. We will explore the power of RAG in AI and how it is being used to generate human-like responses.
Artificial intelligence (AI) has revolutionized every industry today, from healthcare and finance to manufacturing. However, technology still has a long way to go before fully unlocking its potential.
That’s where retrieval-augmented generation (RAG) comes in. RAG is a new AI system that uses machine learning to combine natural language processing with deep understanding.
This technology combines the power of large-scale retrieval with generative models to generate better responses to complex queries. We will discuss the power of retrieval-augmented generation technology in AI and how it could help us unlock the full potential of AI.
The Power of Retrieval Augmented Generation (RAG) in Enterprise Backup and Recovery Software Solutions
With the exponential of data growth of data in the enterprise world, backup and recovery solutions have become integral to any organization’s infrastructure.
In the past, backup and recovery were mainly focused on storing and retrieving data. However, with the advent of new technologies, the focus has shifted towards making these solutions more intelligent and efficient.
One such technology that has gained traction in recent years is the Retrieval-Augmented Generation (RAG). RAG is an artificial intelligence-based technology that can augment natural language generation (NLG) models to assist in knowledge-based tasks.
RAG generates informative and precise responses to users’ queries by combining information retrieval techniques with language modeling.
The need for RAG in enterprise backup and recovery software solutions arises due to the sheer volume and complexity of data that organizations need to manage. These solutions need to handle large volumes of data quickly and efficiently. In addition, they must be able to identify patterns, anomalies, and errors to prevent data loss.
Cohesity’s RAG Platform: Enhancing AI-Driven Conversations with Context-Aware Answers
Cohesity, a leading data management company, has released its latest product: the Cohesity RAG Platform. This platform is designed to enhance the capabilities of AI-driven conversations by providing context-aware answers.
The Cohesity RAG Platform is built on natural language understanding (NLU) technology, allowing it to analyze the meanings of customers’ questions. By understanding the context of the question, the platform can provide more accurate and relevant answers, saving customers time and minimizing frustration.
The Cohesity RAG Platform goes beyond basic FAQs and scripted responses. Instead, it uses machine learning algorithms to continuously improve its understanding of customer needs and preferences. It integrates with existing customer relationship management (CRM) systems to provide seamless customer service.
One of the standout features of the Cohesity RAG Platform is its ability to scale to meet varying levels of demand. It can handle thousands of customer interactions simultaneously, ensuring no customer is left waiting for an answer.
The platform is also customizable, allowing businesses to tailor their responses to fit their brand voice and customer base. This includes the ability to incorporate company-specific terminology and jargon.
Advantages of Retrieval Augmented Generation (RAG) in AI Models
Improved Performance
Retrieval Augmented Generation (RAG) is a technique used in artificial intelligence models to improve the performance of natural language generation.
This technique combines a retrieval-based model with a generative one, allowing for more accurate and efficient text generation. RAG has been shown to outperform traditional generative models in accuracy and speed, making it an ideal choice for applications such as machine translation and summarization.
Increased Efficiency
RAG also increases AI models’ efficiency by reducing the required training data. Traditional generative models require large amounts of training data to generate accurate results, but RAG can yield more accurate results with less data. This makes it ideal for applications with limited data or where data needs to be updated frequently.
Reduced Cost
RAG also reduces the cost associated with developing AI models. By reducing the required training data, RAG can reduce the cost of collecting and labeling large datasets for training purposes.
Because RAG requires fewer resources than traditional generative models, it can help reduce the hardware and software infrastructure costs needed to run them.
Enhanced Accuracy
RAG also enhances the accuracy of generated text by allowing for more control over how words are chosen during generation. Using a retrieval-based model, RAG can select words from a pre-defined set that are most likely to represent the desired output text accurately.
This results in improved accuracy compared to traditional generative models that rely on random sampling techniques for word selection.
Improved Diversity
Another advantage of Retrieval Augmented Generation is its ability to produce diverse outputs without sacrificing accuracy or efficiency. With traditional generative models, diversity often comes at the expense of accuracy, as random sampling techniques can lead to incorrect word choices that result in poor-quality output text.
However, using a retrieval-based approach, RAG can select words from a pre-defined set that are most likely to accurately represent the desired output text while providing diversity in its outputs. This is because it can choose multiple terms from this set for each generated sentence or phrase.
Better Understanding Of Context
In addition to improving performance and efficiency, Retrieval Augmented Generation helps AI models better understand context when generating text or other outputs based on input data or user queries.
Combining retrieval-based and generative models allows RAG to consider past and current inputs when generating outcomes. This results in more accurate results that think contextual information such as user intent or sentiment expressed within an input query or dataset sample.
Improved Human Readability
Another benefit of Retrieval Augmented Generation is its ability to produce human-readable output texts that are easier for people to understand than those produced by traditional generative models, which often contain errors due to their reliance on random sampling techniques during word selection processes.
By combining a retrieval-based model with a generative model, RAG produces more accurate and grammatically correct outputs while maintaining natural-sounding sentences that humans easily understand.
Easier To Implement And Scale Up
Retrieval Augmented Generation is relatively easy to implement and scale up compared with other AI technologies, such as deep learning networks, which require significant computing power and resources.
As such, this makes it an attractive option for businesses looking for an efficient way of implementing AI capabilities without investing heavily in hardware and software infrastructure needed for deep learning networks.
Leveraging RAG to Achieve Accurate and Relevant Responses
Leveraging the RAG (Red, Amber, Green) rating system is essential for accurate and relevant responses. This system provides a visual cue to indicate the level of risk associated with a particular piece of information or response.
The RAG rating system is commonly used in project management and decision-making processes to judge the status or potential of a given situation.
Regarding response accuracy and relevance, the RAG system can be applied to evaluate and rank responses based on their credibility and usefulness.
A “green” reply would signify a reliable and highly relevant answer, whereas an “amber” response may indicate some uncertainty or lack of clarity. A “red” response would be considered unreliable or irrelevant and should be disregarded.
Cohesity at the Forefront: AI-Ready Platform for Retrieval Augmented Generation (RAG)
Cohesity, a leading provider of data management solutions, is at the forefront of innovation with its AI-ready Retrieval Augmented Generation (RAG) platform. The platform is designed to streamline data management and make data retrieval and analysis more efficient.
This innovative platform leverages advanced AI technologies, including natural language processing, machine learning, and deep learning, to deliver intelligent insights that help businesses maximize the value of their data.
The Cohesity RAG platform is built on a robust data management framework that provides a comprehensive layer for storing, protecting, and managing data across the entire enterprise.
With its unified interface, the platform allows businesses to easily access and analyze data from different sources, including on-premises data centers, cloud-based environments, and remote locations.
What Makes Retrieval Augmented Generation (RAG) Unique?
Retrieval Augmented Generation (RAG) is a state-of-the-art natural language processing (NLP) paradigm that combines the power of retrieval-based and generative-based models for a wide range of applications.
Unlike traditional generative models that produce language outputs from scratch, RAG is unique because it first retrieves relevant documents or passages from a large corpus of knowledge and then combines them with a generative model to produce fluent and coherent responses.
This hybrid approach is powerful because it allows the generative model to leverage external knowledge and context beyond the input prompt’s immediate scope.
The retrieval component is typically based on advanced neural network architectures, such as dense or dense passage retrievers, trained on large-scale data collections to retrieve the most relevant passages given the input prompt.
These retrieved passages are then fed into a generative model, often a transformer-based architecture such as GPT-2 or T5, which leverages the retrieved information to generate high-quality outputs that are accurate, coherent, and informative.
Integrating Retrieval and Generative AI: How RAG Works
As the world of artificial intelligence continues to evolve rapidly, researchers and engineers are constantly striving to improve the capabilities of these complex systems.
Recently, there has been growing interest in combining retrieval-based AI systems with generative-based AI systems to create a more robust and versatile tool. This integration is achieved through a framework called Retrieval-Augmented Generation (RAG).
RAG is a framework that incorporates both a retrieval and a generative system. The retrieval system retrieves relevant information from a large dataset, while the productive system generates responses or answers based on the retrieved data.
This framework is instrumental in natural language processing tasks where the AI system needs to understand the context of a question or statement, retrieve relevant information, and generate an appropriate response.
Benefits of Cohesity’s RAG Platform in Enterprise Data Management
Scalability:
Cohesity’s RAG platform is designed to provide enterprise-level scalability, allowing organizations to scale their data management infrastructure as their needs grow. The platform can easily accommodate large amounts of data, ensuring businesses can store and manage their data efficiently.
Data Protection:
Cohesity’s RAG platform provides comprehensive data protection features, including backup, replication, and disaster recovery capabilities. These features help ensure that businesses can protect their data from loss or corruption in the event of a system failure or natural disaster.
Automation:
The RAG platform is designed to automate many tedious and time-consuming data management tasks, such as backup and recovery operations. This automation helps reduce manual labor costs while increasing accuracy and efficiency in managing critical business data.
Security:
Cohesity’s RAG platform includes robust security features that help protect sensitive business data from unauthorized access or malicious attacks. The platform also supports encryption for added protection against external threats.
Cost Savings:
By leveraging the RAG platform, businesses can achieve significant cost savings in hardware, software, and personnel costs associated with traditional on-premise storage solutions. The platform also helps to reduce operational expenses by automating many routine tasks related to managing large amounts of data.
Flexibility:
With its modular architecture, the RAG platform allows organizations to customize their storage solutions according to their specific needs and requirements without sacrificing performance or reliability. This flexibility helps ensure that businesses can meet their unique requirements while maintaining a high level of performance at an affordable cost.
Efficiency:
Cohesity’s RAG platform is designed to maximize efficiency in managing large amounts of enterprise data by utilizing advanced technologies such as deduplication and compression algorithms, which reduce storage space requirements while maintaining high performance and reliability levels for critical business applications and processes.
Analytics & Insights:
With its built-in analytics engine, the RAG platform provides users with real-time insights into how their data is being used within the organization. It also provides predictive analytics capabilities that allow organizations to better understand trends in usage patterns over time so they can make informed decisions about how best to manage their enterprise data resources in the future.
Real-Life Applications of Retrieval Augmented Generation (RAG)
Autocomplete
Retrieval Augmented Generation (RAG) can create an autocomplete feature for text input fields. With RAG, an algorithm generates a list of suggestions based on the user’s input. This can help reduce typing errors and make it easier for users to find the information they seek.
Image Recognition
RAG can also be used in image recognition applications. For example, an algorithm could use RAG to recognize objects in a picture, such as animals or buildings. This could be useful for facial recognition or object detection in photos and videos.
Machine Translation
RAG can be used to create more accurate machine translations of text from one language to another. Using RAG, an algorithm can generate multiple translations of the same sentence and then select the most precise translation based on its understanding of the context of the sentence.
Natural Language Processing (NLP)
RAG has many applications in Natural Language Processing (NLP). For example, it can generate summaries of text documents or automatically detect sentiment in text data. It can also create questions from a given answer or vice versa, which is helpful for question-answering systems and chatbots.
Automated Essay Scoring
RAG algorithms can also automate essay scoring by identifying key phrases and concepts that indicate a high-quality essay has been written. This could reduce the time required for manual grading and increase accuracy by eliminating human bias when assessing articles written by students or job applicants.
Text Summarization
Text summarization is another area where RAG algorithms can be applied effectively. By analyzing a text document, an algorithm can identify important concepts and generate a summary that captures the document’s main points without losing any essential information or omitting important details. This could have many applications in education, journalism, and research writing.
Voice Generation
RAG algorithms can also generate synthetic voices that sound like real people speaking naturally without robotic intonation or awkward pauses between words and sentences. This technology could revolutionize how audio recordings are created for podcasts, audiobooks, video games, movies, TV shows, etc., reducing production costs while increasing audio quality.
Music Composition
With some impressive results, RAG algorithms have been used successfully to compose music. Algorithms trained on large datasets of existing songs have produced original compositions that sound like those written by professional musicians. This technology could open up new possibilities for creating realistic-sounding music with minimal effort.
The Future of AI Conversations: Cohesity’s RAG-Driven AI System
Utilizing Natural Language Processing (NLP)
Natural language processing (NLP) is a branch of artificial intelligence that enables machines to understand and interact with humans in natural language.
Cohesity’s Rag-driven AI System uses NLP to facilitate conversations between AI agents and humans. This system allows for more natural interactions between the two, as it can recognize and respond to human speech patterns.
Understanding Intent
The Rag-driven AI System also utilizes intent recognition technology to understand better what the user is asking or trying to accomplish. This allows the AI agent to interpret the user’s input more accurately and respond appropriately. The system also considers context, which helps it better understand the meaning behind a user’s words and phrases.
Generating Responses
Once the Rag-driven AI System has determined the user’s intent, it generates an appropriate response based on its understanding of the conversation. This response can be verbal or written, depending on how the conversation was initiated. The system can also generate multiple answers for each conversation, allowing greater flexibility in user interactions.
Utilizing Machine Learning
The RAG-Driven AI System utilizes machine learning algorithms to improve its performance continually. As it interacts with users, it collects data about their conversations, which is used to train its algorithms and make them more accurate and responsive in future discussions.
This ensures that users receive a more personalized experience each time they interact with the system as it learns from past conversations.
Enhancing Human Interactions
The Rag-driven AI System is designed to enhance human interactions by providing a more natural interface for communication between people and machines.
This system enables smoother conversations between humans and machines while providing accurate real-time responses by utilizing NLP, intent recognition technology, machine learning algorithms, and other advanced technologies.
Increasing Efficiency
Another benefit of using Cohesity’s Rag-driven AI System is that it increases efficiency when dealing with customer inquiries or tasks requiring extensive data processing or analysis.
By automating specific processes, this system can reduce costs associated with manual labor while still maintaining accuracy. This system can also help speed up customer service times, as customers won’t have to wait for an employee to manually process their request before receiving a response.
Conclusion:
Retrieval-augmented generation technology (RAG) is a game-changer for AI and its applications. As machine learning and AI expand, RAG technology will enable AI models to solve complex tasks more effectively. The technology has already been helpful in healthcare, customer service, and content creation.
RAG technology has significantly improved natural language understanding, making it a powerful tool for NLP tasks like search engine algorithms and IVR customer service. We should be excited about what the future holds for RAG technology and its potential contributions to the AI industry.
All in all, the Retrieval Augmented Generation model offers a significant breakthrough for AI. By combining the power of generative models with retrieval models, RAG can generate coherent and contextually relevant responses to complex queries.
RAG holds immense potential in many industries, including customer service and healthcare, and is set to revolutionize how AI operates. As technology evolves, we can expect RAG to become a crucial component in advancing AI, helping us solve complex problems and provide solutions in previously impossible ways.
Call: +91 9848321284
Email: [email protected]